Development and validation of a basement membrane-associated immune prognostic model for hepatocellular carcinoma
Highlight box
Key findings
• We developed a prognostic model for hepatocellular carcinoma (HCC) based on basement membrane (BM)-related genes, termed BMscore, which effectively predicts patient survival and immune characteristics. Key genes P3H1 and ADAMTS5 were identified as significantly associated with poor prognosis in HCC.
What is known and what is new?
• It is known that HCC has a poor prognosis and a complex immune response, with BM proteins playing critical roles in tumor invasion and immune regulation.
• Our study is the first to identify P3H1 and ADAMTS5 as biomarkers for prognosis and immune microenvironment in HCC, demonstrating that BMscore aids in more precise immunotherapy selection.
What is the implication, and what should change now?
• BMscore can be utilized for risk stratification and immunotherapy decision-making in HCC patients. Future research should focus on functional validation of these biomarkers and the development of targeted therapies to optimize individualized treatment for HCC.
Introduction
Hepatocellular carcinoma (HCC) is the most prevalent form of liver cancer, marked by high incidence and mortality rates (1). Due to its insidious onset and rapid progression, most patients are diagnosed at advanced stages, missing the opportunity for surgical intervention (2,3). While targeted and immune therapies have improved outcomes for advanced HCC to some extent (4-6), prognosis remains unsatisfactory. Moreover, patients with similar pathological staging and clinicopathological characteristics exhibit diverse prognoses, potentially due to varying responses to chemotherapy or immunotherapy. This underscores the need to identify underlying molecular factors that can modulate the tumor microenvironment, thereby influencing HCC treatment and prognosis. Hence, identifying reliable biomarkers is crucial for enhancing the diagnosis, prognosis, and therapeutic strategies for HCC.
Basement membrane (BM) is the thin, dense extracellular matrix (ECM) that covers the basal surface of epithelial cells and endothelial cells (7,8). The major components of it are laminin, collagen IV, heparan sulfate proteoglycans, and nidogen, which serve as structural barriers against cancer cell invading adjacent or distant tissues (9-11). Tumor metastasis is the leading causes of treatment failure and cancer-related mortality, and the degradation of the cellular BM is the basis of tumor metastasis. Considerable evidence suggests that BM-related genes play an important role in the prognosis of various tumors, including renal cell carcinoma (12-14), bladder cancer (15), lung adenocarcinoma (16), breast cancer (17), and colorectal cancer (18). Recently, a growing body of evidence suggests that HCC invasion and metastasis are linked to BM destruction (19-22). However, research exploring the prognostic significance of BM-associated gene signatures in HCC, including their impact on prognosis and treatment outcomes, remains limited. Developing a BM-related signature capable of predicting HCC prognosis and improving immunotherapy efficacy would be highly beneficial.
In this study, following consensus clustering analysis using BM-related genes, HCC samples were effectively stratified into two distinct groups based on their molecular profiles: a high-expression group and a low-expression group. After comparing the differences between the two groups, we found significant disparities in overall survival (OS) and levels of immune cell infiltration. Additionally, functional enrichment analysis was conducted on differentially expressed genes (DEGs) between the two groups. Univariate Cox regression and least absolute shrinkage and selection operator (LASSO) regression were used to construct a BMscore model involving 11 BM-related risk genes, which could potentially predict prognostic outcomes for HCC patients. Moreover, employing advanced machine learning techniques, we streamlined the initial set of 11 genes to highlight two critical ones: P3H1 and ADAMTS5. These study findings contribute to a better understanding of the potential mechanisms through which BM-related genes influence the prognosis of patients with HCC. The authors of this study believe that the identified signature represents a promising advancement in early diagnosis and immunotherapy for HCC patients. We present this article in accordance with the TRIPOD reporting checklist (available at https://tgh.amegroups.com/article/view/10.21037/tgh-24-89/rc).
Methods
Data acquisition and processing
The RNA sequencing (RNA-seq) transcriptome data of liver hepatocellular carcinoma (LIHC) (374 HCC and 50 normal) cohort, along with corresponding clinicopathologic characteristics and prognostic information, were obtained from The Cancer Genome Atlas (TCGA; https://cancergenome.nih.gov/) as a training dataset, excluding cases with no survival data. Gene expression data from TCGA-LIHC were downloaded in the format of fragments per kilobase million (FPKM) and analyzed. Transcriptome profiling data of GSE54236 were downloaded from the Gene Expression Omnibus (GEO; http://www.ncbi.nlm.nih.gov/geo) database as a validation cohort, which contains survival time and survival status. A total of 222 BM-related genes were used for subsequent analysis according to a previous study by Jayadev et al. (23). The study was conducted in accordance with the Declaration of Helsinki (as revised in 2013).
Identification of differentially expressed BM-related genes between HCC and adjacent normal tissues
Based on the cutoff values of |log fold change (logFC)| >0 and P value <0.05, the differentially expressed BM-related genes between HCC and adjacent normal tissues were identified using the R package “limma”.
Consensus clustering analysis
We analyzed BM-related genes using unsupervised consensus clustering and determined subtype differentiation through principal component analysis (PCA). The R package “ConsensusClusterPlus” was used to determine the number of clusters, repeating the process 100 times with pItem =0.8 to test cluster stability. The best clustering effect was observed when the k value was 2. Kaplan-Meier analysis was conducted to estimate OS differences between clusters, and the log-rank test was used to evaluate these survival differences.
Identification of DEGs between clusters and enrichment analysis
We conducted enrichment analyses, including Gene Ontology (GO), Kyoto Encyclopedia of Genes and Genomes (KEGG), and gene set enrichment analysis (GSEA), based on the DEGs between clusters using the R package “clusterProfiler”. GO functional annotation covered biological process (BP), cellular component (CC), and molecular function (MF). GSEA analysis utilized the “c5.go.symbols.gmt” and “c2.cp.kegg.symbols.gmt” databases as references.
Tumor immune landscape analysis between different clusters
We analyzed the proportions of 22 immune cell subtypes in the TCGA cohort using the Cell type Identification By Estimating Relative Subsets Of known RNA Transcripts (CIBERSORT) algorithm (24). The Estimation of STromal and Immune cells in MAlignant Tumours using Expression data (ESTIMATE) algorithm (25) was employed to calculate ESTIMATE scores, immune scores, stromal scores, and tumor purity, aiming to explore the tumor immune microenvironment (TIME) across different clusters. The CIBERSORT algorithm was configured with 1,000 permutations to accurately estimate immune cell proportions. One-way analysis of variance (ANOVA) was conducted to assess differences in the expression of human leukocyte antigen (HLA) proteins and immune checkpoint molecules among the identified clusters.
Construction and validation of prognostic model based on BM-related genes
To construct and validate a prognostic model based on BM-related genes, we first identified the intersection of genes between the TCGA-LIHC and GSE54236 datasets. Batch correction was performed to harmonize the gene expression data across datasets. Gene expression levels associated with the BM were extracted for subsequent analysis. Univariate Cox regression analysis was employed to identify prognostic genes associated with OS. Subsequently, LASSO regression was utilized to develop a risk score calculation formula. This formula was applied to both the TCGA dataset and an external validation dataset to stratify patients into high- and low-risk groups, using the median score as the cutoff point. To assess the predictive performance of the model, Kaplan-Meier survival analysis was conducted, and risk score distribution figures were plotted.
Identification of hub BM-related gene using machine learning techniques
To identify central hub genes crucial for the prognosis and treatment of HCC, we utilized random forest (RF) and support vector machine (SVM) algorithms. The “e1071” R package was employed for SVM, while the “randomForest” R package was used for RF (26). We intersected the genes identified by LASSO regression with those identified by RF and SVM algorithms. Genes identified through overlapping analyses were considered central hub biomarkers.
Exploring hub biomarkers expression differences, clinical associations, and tumor microenvironment distribution
Wilcoxon’s test was employed to analyze the expression levels of potential hub biomarkers between HCC and adjacent normal tissues. It is crucial to investigate the correlation between biomarker expression levels and clinicopathological characteristics, immune checkpoints, and various functional pathways to comprehend their diagnostic and predictive roles. Additionally, single-cell RNA-seq analysis was utilized to explore the expression abundance of biomarker genes across different cell types.
Cell culture
L02, HepG2, Hep3B, and Huh7 cells were purchased from the American Type Culture Collection (ATCC, Rockville, MD, USA). The human normal liver cell line L02 was cultured in RPMI-1640 medium supplemented with 10% fetal bovine serum (FBS), 100 U/mL penicillin, and 100 µg/mL streptomycin. The human HCC cell lines HepG2, Hep3B, and Huh7 were cultured in high-glucose Dulbecco’s Modified Eagle Medium (DMEM) with 10% FBS, 100 U/mL penicillin, and 100 µg/mL streptomycin. All cells were incubated at 37 ℃ in a humidified atmosphere with 5% CO2. Medium was replaced every 2 days, and cells were passaged at 80–90% confluence using 0.25% trypsin- ethylenediaminetetraacetic acid (EDTA). Cell counts were determined using a hemocytometer prior to seeding for experiments.
RNA extraction and quantitative real-time polymerase chain reaction (qRT-PCR)
Total RNA was extracted from L02, Huh7, HepG2, and Hep3B cells using the FastPure Cell/Tissue Total RNA Isolation Kit V2 (No. RC112-01, Vazyme Biotech Co., Ltd., Nanjing, China) following the manufacturer’s instructions. For reverse transcription, 1 µg of total RNA was converted into complementary DNA (cDNA) using the HiScript III 1st Strand cDNA Synthesis Kit (+gDNA wiper) (No. R312-01, Vazyme Biotech Co., Ltd.). RNA concentration and purity were assessed by evaluating the optical density (OD) of 260 nm/280 nm, with acceptable A260/A280 ratios between 1.8 and 2.0. β-actin was used as the internal control. The primers for P3H1, ADAMTS5, and β-actin were designed as follows: P3H1, forward 5'-GATCCAGGACAGGGTGCAG-3', reverse 5'-GCTCATCCTTGGGCTTCGAT-3'; ADAMTS5, forward 5'-TGTGCTGTGATTGAAGACGAT-3', reverse 5'-GACTGCAGGAGCGGTAGATCG-3'; β-actin, forward 5'-TTCCTTCCTGGGCATGGAGTC-3', reverse 5'-TCTTCATTGTGCTGGGTGCC-3'.
qRT-PCR was conducted using the ChamQ SYBR qPCR Master Mix (No. Q311-02, Vazyme Biotech Co., Ltd.) in the AB7500 RT-PCR instrument (Applied Biosystems, Foster City, CA, USA). Relative expression levels of the target genes were calculated using the 2−ΔΔCt method, and all experiments were performed in triplicate.
Statistical analysis
All statistical analyses were performed using R version 4.4.0. Differences between the two groups were analyzed using either the Wilcoxon rank-sum test (for nonparametric data) or Student’s t-test (for parametric data). One-way ANOVA was used for comparisons among three or more groups. The Mann-Whitney U test was employed as a nonparametric alternative when appropriate. Pearson’s correlation test was used for assessing linear relationships between two variables, while Spearman’s correlation test was applied for non-linear relationships or rank-based correlations. Survival differences were evaluated using Kaplan-Meier curves and assessed with the log-rank test. All statistical tests were two-sided, and P value <0.05 was considered statistically significant.
Results
DEGs identification and BM-related tumor cluster classification
The study flow chart is depicted in Figure 1. A total of 166 DEGs, including 147 up-regulated and 19 down-regulated genes, were identified between HCC and adjacent normal tissues (Figure S1). Cluster analysis was conducted on 370 tumor samples from the TCGA cohort based on 222 BM-related gene expressions. Optimal clustering at k=2 revealed distinct groups: the high BM-related gene expression group (BM high group, C1) and the low BM-related gene expression group (BM low group, C2) (Figure 2A). We identified 6,221 DEGs between the two groups, including 5,863 upregulated genes and 358 downregulated genes, with a screening criterion of |logFC| >1 and P value <0.05. A heatmap illustrated gene expression differences between these groups (Figure 2B). Kaplan-Meier survival analysis indicated poorer prognosis in the BM high group compared to the BM low group (Figure 2C).
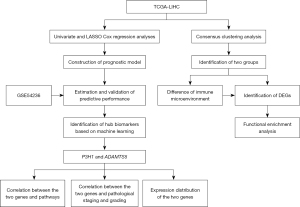
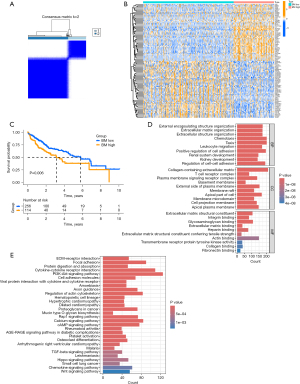
Functional enrichment analysis between high and low BM groups
We identified DEGs between the high and low BM groups and conducted functional pathways enrichment analyses using GO, KEGG, and GSEA. GO analysis revealed several significant pathways: in BP, pathways such as external encapsulating structure organization, ECM organization, extracellular structure organization, chemotaxis, taxis, leukocyte migration, positive regulation of cell adhesion, and regulation of cell-cell adhesion were enriched (Figure 2D). The CC category highlighted pathways including collagen-containing ECM, T cell receptor complex, plasma membrane signaling receptor complex, BM, external side of plasma membrane, membrane raft, apical part of cell, membrane microdomain, cell projection membrane, and apical plasma membrane (Figure 2D). MF pathways such as ECM structural constituent, integrin binding, glycosaminoglycan binding, ECM binding, heparin binding, ECM structural constituent conferring tensile strength, actin binding, transmembrane receptor protein tyrosine kinase activity collagen binding, and fibronectin binding were prominent (Figure 2D).
KEGG pathway analysis highlighted several key pathways influenced by DEGs, including ECM-receptor interaction, focal adhesion, protein digestion and absorption, cytokine-cytokine receptor interaction, PI3K-Akt signaling pathway, cell adhesion molecules, viral protein interaction with cytokine and cytokine receptor and others (Figure 2E). These findings underscore the impact of these genes on specific cellular and molecular activities.
GSEA further revealed enriched pathways in the BM high group, including collagen fibril organization, external encapsulating structure organization, BM, ECM structural constituent, ECM structural constituent conferring tensile strength, cytokine cytokine-receptor interaction, ECM receptor interaction, and focal adhesion (Figure S2). However, the BM low group seemed to be positively associated with metabolism (Figure S2).
This comprehensive analysis across GO, KEGG, and GSEA provides detailed insights into the functional pathways influenced by DEGs, enhancing our understanding of their biological implications.
Immune landscape analysis between BM high and low groups
The ESTIMATE algorithm was utilized to estimate immune and stromal components in the tumor microenvironment. We observed higher immune score, stromal score, and ESTIMATE score in the BM high group, whereas tumor purity was lower in the BM low group (Figure 3A). Figure 3B,3C also highlighted differences in HLA and immune checkpoints expression between the two groups. Comparative analysis revealed that the BM high group exhibited significantly lower infiltration abundances of plasma cells, CD8+ T cell, CD4 memory-activated T cells, follicular helper T cells, along with higher infiltration abundances of CD4 memory resting T cells and macrophage M0 compared to the BM low group (Figure 3D). These findings underscore the significant role of BM-related genes in modulating the immune microenvironment.
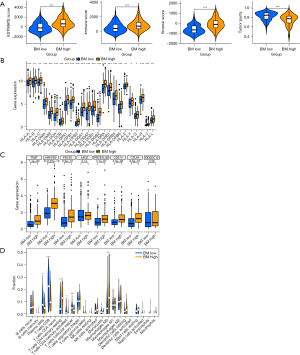
Construction of genetic risk model
The results of univariate Cox regression analysis were visualized using a forest plot. A total of 60 genes showed significant associations with the prognosis of HCC (Figure 4A). LASSO regression analysis was then conducted to reduce the number of genes and refine the model (Figure 4B). Ultimately, 11 genes were selected to construct a risk model named BMscore, and prognostic Kaplan-Meier curves were plotted (Figure 4C,4D). The high-risk group exhibited a worse prognosis compared to the low-risk group in both the training and validation datasets. The distribution plot of the risk score suggested that BMscore is a robust prognostic indicator for HCC in both datasets (Figure S3).
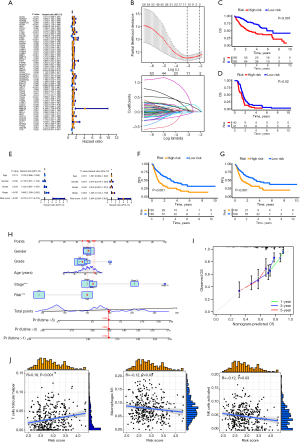
Furthermore, the univariate regression analysis showed that the TNM stage and risk score were significantly related to HCC prognosis (Figure 4E). The multivariate regression analysis showed that higher tumor-node-metastasis (TNM) stage and higher risk score suggested worse prognosis (Figure 4E). We observed that the high-risk group had a poorer prognosis compared to the low-risk group based on TCGA-LIHC database for disease-free survival (DFS) (Figure 4F) and progression-free survival (PFS) (Figure 4G).
Additionally, we plotted a nomogram based on clinical characteristics and BMscore, and investigated the C-index to estimate the predictive performance of the nomogram (Figure 4H,4I). Meanwhile, we observed positive correlation between risk score and T cells follicular helper (R=0.18, P<0.001), and negative correlation with macrophages M1 (R=−0.12, P=0.03) and NK cells activated (R=−0.12, P=0.03) (Figure 4J).
Identification and functional analysis of hub biomarkers in HCC
To identify pivotal biomarkers for diagnosis and prognosis in HCC patients, we conducted an intersection analysis of three machine learning-derived genes using a Venn plot (Figure 5A,5B), resulting in the identification of three hub biomarkers: P3H1, ADAMTS5, and GPC1 (Figure 5C). Notably, the expression of GPC1 did not significantly differ between HCC and adjacent normal tissues (Figure 5D). We found that high expression of P3H1 and ADAMTS5 was each associated with a poorer prognosis, indicating that both are potential high-risk genes in HCC (Figure 5E). Interestingly, compared to ADAMTS5, P3H1 showed a stronger positive correlation with tumor mutational burden (Figure 5F). However, the two genes are strongly correlated with each other, suggesting potential interaction mechanisms that warrant further analysis (Figure 5G).
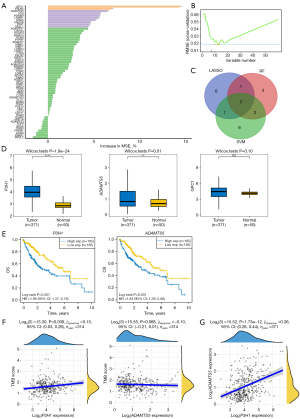
Further analysis revealed that P3H1 and ADAMTS5 were positively associated with several pathways, including DNA replication, tumor proliferation signature, G2M checkpoint, degradation of ECM, epithelial-mesenchymal transition (EMT) markers, and ECM-related genes. Interestingly, P3H1 primarily functions in the context of cellular proliferation, whereas ADAMTS5 predominantly regulates ECM metabolism (Figure 6A,6B). Additionally, we investigated the relationship between the expression levels of the P3H1 and ADAMTS5 genes and the pathological staging and grading of HCC patients. We discovered a commonality between the two, specifically that the expression levels of P3H1 and ADAMTS5 are significantly correlated with the T staging and overall staging of HCC (Figures S4,S5). However, we also identified a difference between them: the expression level of P3H1 is related to tumor grading, while ADAMTS5 is associated with lymph node metastasis (Figures S4,S5).
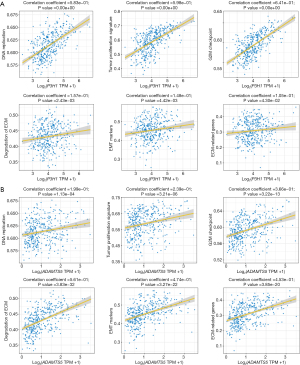
Furthermore, single-cell sequencing analysis indicated that ADAMTS5 is expressed in mast cells (10× GSE140228), endothelial cells (GSE125449 and GSE166635), and fibroblasts (GSE125449 and GSE166635) (Figure 7A). In contrast, besides its expression in endothelial and fibroblast cells, P3H1 showed predominant expression in immune cell types such as Tprolif, CD8Tex, dendritic cells (DCs), regulatory T cells (Tregs), natural killer (NK) cells, innate lymphoid cells (ILCs), CD4Tconv cells, CD8T cells, and B cells (Figure 7B).
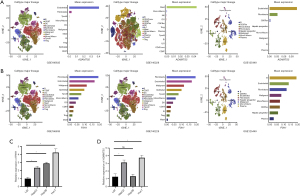
qRT-PCR analysis revealed that the expression level of P3H1 was higher in HepG2, Hep3B, and Huh7 cell lines compared to the normal liver cell line L02 (Figure 7C), while the expression level of ADAMTS5 was higher in HepG2 and Huh7 compared to L02, but showed no significant difference in Hep3B compared to L02 (Figure 7D).
These findings underscore the diverse roles of P3H1 and ADAMTS5 across different cellular contexts and highlight their potential implications in the pathogenesis and treatment of HCC.
Discussion
In this study, we developed a robust prognostic model for HCC by systematically analyzing BM-related genes. Through comprehensive gene expression analysis, we constructed an 11-gene prognostic model, termed BMscore, which effectively stratified patients based on their risk and predicted OS. Within this model, P3H1 and ADAMTS5 were identified as particularly significant genes due to their strong association with HCC prognosis. Additionally, BMscore was instrumental in assessing the prognosis, immune landscape, and potential response to immunotherapy, offering a valuable tool for personalized treatment strategies in HCC. These findings underscore the critical role of BM-related genes, especially P3H1 and ADAMTS5, in HCC progression and highlight the potential of our model to enhance prognostic accuracy and guide therapeutic decisions.
HCC, the most common primary liver cancer and one of the deadliest cancers globally, is marked by its poor prognosis, primarily due to the tumor’s aggressive invasiveness and metastatic potential (27). The BM, which plays a crucial role in impeding tumor cell invasion, thereby influences the prognosis of HCC patients significantly. Typically, tumor cells generate invadosomes rich in proteases, which can break down BM components to create entry points. Research indicates that cells can penetrate the BM using proteolytic processes, and cancer-associated fibroblasts (CAFs) can widen the BM to form gaps, facilitating the migration of tumor cells (28). This conclusion aligns closely with our observations. Therefore, gaining a deeper understanding of the expression patterns and mechanisms of BM proteins in HCC patients could potentially improve their prognosis.
In this study, we constructed a predictive model based on BM-related genes, which can be used to forecast the prognosis and immunotherapy outcomes for HCC patients. We first divided the samples into two groups according to the expression pattern of 222 BM-related genes by consistency cluster analysis. Then, the difference of OS, immune landscape, and enrichment pathway between the two groups were examined. The univariate regression results indicated that a total of 60 factors could serve as prognostic indicators for HCC. The LASSO model was then applied to further identify 11 BM-related genes for constructing the prognostic model. The GSE54236 dataset was used to verify the prognostic value and accuracy of the model. The results indicated that the high-risk group had a poor prognosis, and the model’s prognostic performance was satisfactory. Additionally, correlation analysis revealed different immune cell infiltrations between the two groups, including activated NK cells, M1 macrophages, and naive B cells. Notably, the results demonstrated that our model could be used to predict the prognosis of HCC patients.
To identify more reliable prognostic and therapeutic targets from the genes used in the construction of the prediction model, we utilized multiple machine learning approaches to identify intersecting genes. For ADAMTS5, it appears that some cancers have increased ADAMTS5 expression, including hepatocellular cancer (29). Zhu et al. found that high ADAMTS5 expression was positively correlated with the development of HCC and significantly associated with poorer survival (30). The above arguments were well-demonstrated in HCC in our study. P3H1, the enzyme encoded by the prolyl 3-hydroxylase 1 gene, is responsible for collagen synthesis and assembly (31). Previous reports have shown that in HCC, high P3H1 expression is associated with aggressive tumors and poor prognosis (32). Other studies have suggested that P3H1 can serve as a prognostic biomarker for various tumors, with its elevated expression linked to poor outcomes in most cancers (31,33). P3H1 expression is also strongly associated with immune cell infiltration and immune-related genes (31).
Furthermore, this study comprehensively analyzed the interactions of P3H1 and ADAMTS5 with key pathways regulating cell proliferation and the ECM, and employed single-cell datasets to explore the distribution of these genes across different cell types within the HCC immune microenvironment. In HCC, we observed that P3H1 primarily participates in cell replication and proliferation, while ADAMTS5 is mainly involved in ECM metabolism. Furthermore, compared to its distribution in the tumor microenvironment, P3H1 exhibits higher expression in immune cells. These findings provide valuable guidance for future improvements in targeted therapy for HCC.
In recent years, more and more patients with HCC have gained considerable survival benefits from immunotherapies, such as immune-checkpoint inhibitors (ICIs), which are revolutionizing the management of HCC. The combination of atezolizumab and bevacizumab has outperformed sorafenib in advanced HCC, setting a new first-line median OS benchmark of 19 months and marking a breakthrough in disease management (34). Likewise, the combination of the sintilimab and a bevacizumab biosimilar (IBI305) has shown improved OS in Chinese patients with advanced hepatitis B virus (HBV)-associated HCC compared to sorafenib (35). Unfortunately, HCC patients sometimes fail to exhibit enduring and sufficient immune responses to immunotherapies due to multiple tumor-evolved mechanisms for evading immune surveillance and destruction (36). These include regional immunosuppression, dysregulation of T-cell signaling, and upregulation of inhibitory immune checkpoints, which normally prevent the immune system from overreacting to healthy cells (37).
In our study, we discovered that BM-related genes regulate the immune microenvironment in HCC. This regulation is due to their association with immune cell infiltration, ESTIMATE score, immune checkpoint gene expression levels, and HLA expression levels. Considering these facts, it is reasonable to suggest that appropriate immunotherapeutic strategies could be selected for HCC patients by classification based on the BM-related genes.
Taken together, the BM-related genes provide effective means to predict the survival rate and immunotherapeutic response for patients with HCC. BMscore has been indicated by cross-validation as performing effectively across different datasets and showing high robustness in survival rate prediction.
Conclusions
Overall, we developed a prognostic model called BMscore by systematically analyzing BM-related genes, which was used to assess prognosis, immune landscape, and immunotherapy effectiveness in HCC patients based on their risk scores. We analyzed the differences in gene expression levels between HCC and adjacent normal tissues, identifying P3H1 and ADAMTS5 as potential regulators of cell proliferation and the immune system. These genes may play a role in suppressing the development of HCC. Nevertheless, several limitations should be acknowledged. First, the sample size was relatively small, and the study relied on retrospective data, which may limit the generalizability of our findings. Second, although we identified potential biomarkers and therapeutic targets, functional validation experiments were not conducted to confirm their biological relevance. Lastly, the heterogeneity of HCC and its microenvironment, including variations in genetic, epigenetic, and immune profiles, were not fully addressed. This lack of comprehensive analysis could affect the applicability of our results to all patient subsets. Future research should focus on addressing these limitations to strengthen the clinical utility of our findings.
Acknowledgments
None.
Footnote
Reporting Checklist: The authors have completed the TRIPOD reporting checklist. Available at https://tgh.amegroups.com/article/view/10.21037/tgh-24-89/rc
Data Sharing Statement: Available at https://tgh.amegroups.com/article/view/10.21037/tgh-24-89/dss
Peer Review File: Available at https://tgh.amegroups.com/article/view/10.21037/tgh-24-89/prf
Funding: This work was supported by
Conflicts of Interest: All authors have completed the ICMJE uniform disclosure form (available at https://tgh.amegroups.com/article/view/10.21037/tgh-24-89/coif). The authors have no conflicts of interest to declare.
Ethical Statement: The authors are accountable for all aspects of the work in ensuring that questions related to the accuracy or integrity of any part of the work are appropriately investigated and resolved. The study was conducted in accordance with the Declaration of Helsinki (as revised in 2013).
Open Access Statement: This is an Open Access article distributed in accordance with the Creative Commons Attribution-NonCommercial-NoDerivs 4.0 International License (CC BY-NC-ND 4.0), which permits the non-commercial replication and distribution of the article with the strict proviso that no changes or edits are made and the original work is properly cited (including links to both the formal publication through the relevant DOI and the license). See: https://creativecommons.org/licenses/by-nc-nd/4.0/.
References
- Cao W, Chen HD, Yu YW, et al. Changing profiles of cancer burden worldwide and in China: a secondary analysis of the global cancer statistics 2020. Chin Med J (Engl) 2021;134:783-91. [Crossref] [PubMed]
- Torre LA, Bray F, Siegel RL, et al. Global cancer statistics, 2012. CA Cancer J Clin 2015;65:87-108. [Crossref] [PubMed]
- Yang JD, Roberts LR. Hepatocellular carcinoma: A global view. Nat Rev Gastroenterol Hepatol 2010;7:448-58. [Crossref] [PubMed]
- Sperandio RC, Pestana RC, Miyamura BV, et al. Hepatocellular Carcinoma Immunotherapy. Annu Rev Med 2022;73:267-78. [Crossref] [PubMed]
- Li J, Yang F, Li J, et al. Postoperative adjuvant therapy for hepatocellular carcinoma with microvascular invasion. World J Gastrointest Surg 2023;15:19-31. [Crossref] [PubMed]
- Damaskos C, Garmpis N, Dimitroulis D, et al. Targeted Therapies for Hepatocellular Carcinoma Treatment: A New Era Ahead-A Systematic Review. Int J Mol Sci 2022;23:14117. [Crossref] [PubMed]
- Yurchenco PD. Basement membranes: cell scaffoldings and signaling platforms. Cold Spring Harb Perspect Biol 2011;3:a004911. [Crossref] [PubMed]
- Kelley LC, Chi Q, Cáceres R, et al. Adaptive F-Actin Polymerization and Localized ATP Production Drive Basement Membrane Invasion in the Absence of MMPs. Dev Cell 2019;48:313-328.e8. [Crossref] [PubMed]
- Chang J, Chaudhuri O. Beyond proteases: Basement membrane mechanics and cancer invasion. J Cell Biol 2019;218:2456-69. [Crossref] [PubMed]
- Lambert AW, Pattabiraman DR, Weinberg RA. Emerging Biological Principles of Metastasis. Cell 2017;168:670-91. [Crossref] [PubMed]
- Chen G, Wang Y, Wu P, et al. Reversibly Stabilized Polycation Nanoparticles for Combination Treatment of Early- and Late-Stage Metastatic Breast Cancer. ACS Nano 2018;12:6620-36. [Crossref] [PubMed]
- Zhou T, Chen W, Wu Z, et al. A newly defined basement membrane-related gene signature for the prognosis of clear-cell renal cell carcinoma. Front Genet 2022;13:994208. [Crossref] [PubMed]
- Tao J, Li X, Liang C, et al. Expression of basement membrane genes and their prognostic significance in clear cell renal cell carcinoma patients. Front Oncol 2022;12:1026331. [Crossref] [PubMed]
- Xiong X, Chen C, Yang J, et al. Characterization of the basement membrane in kidney renal clear cell carcinoma to guide clinical therapy. Front Oncol 2022;12:1024956. [Crossref] [PubMed]
- Feng L, Yang J, Zhang W, et al. Prognostic significance and identification of basement membrane-associated lncRNA in bladder cancer. Front Oncol 2022;12:994703. [Crossref] [PubMed]
- Chen K, Liu S, Lu C, et al. A prognostic and therapeutic hallmark developed by the integrated profile of basement membrane and immune infiltrative landscape in lung adenocarcinoma. Front Immunol 2022;13:1058493. [Crossref] [PubMed]
- Wisdom KM, Indana D, Chou PE, et al. Covalent cross-linking of basement membrane-like matrices physically restricts invasive protrusions in breast cancer cells. Matrix Biol 2020;85-86:94-111. [Crossref] [PubMed]
- Luo X, Fong ELS, Zhu C, et al. Hydrogel-based colorectal cancer organoid co-culture models. Acta Biomater 2021;132:461-72. [Crossref] [PubMed]
- Li Y, Yang D, Wang Y, et al. Co-delivery doxorubicin and silybin for anti-hepatoma via enhanced oral hepatic-targeted efficiency. Int J Nanomedicine 2019;14:301-15. [Crossref] [PubMed]
- Brown GT, Murray GI. Current mechanistic insights into the roles of matrix metalloproteinases in tumour invasion and metastasis. J Pathol 2015;237:273-81. [Crossref] [PubMed]
- Jiang Z, Han B, Li H, et al. Preparation and anti-tumor metastasis of carboxymethyl chitosan. Carbohydr Polym 2015;125:53-60. [Crossref] [PubMed]
- Shen Q, Cicinnati VR, Zhang X, et al. Role of microRNA-199a-5p and discoidin domain receptor 1 in human hepatocellular carcinoma invasion. Mol Cancer 2010;9:227. [Crossref] [PubMed]
- Jayadev R, Morais MRPT, Ellingford JM, et al. A basement membrane discovery pipeline uncovers network complexity, regulators, and human disease associations. Sci Adv 2022;8:eabn2265. [Crossref] [PubMed]
- Chen B, Khodadoust MS, Liu CL, et al. Profiling Tumor Infiltrating Immune Cells with CIBERSORT. Methods Mol Biol 2018;1711:243-59. [Crossref] [PubMed]
- Yoshihara K, Shahmoradgoli M, Martínez E, et al. Inferring tumour purity and stromal and immune cell admixture from expression data. Nat Commun 2013;4:2612. [Crossref] [PubMed]
- Noble WS. What is a support vector machine? Nat Biotechnol 2006;24:1565-7. [Crossref] [PubMed]
- Nguyen AT, Emelyanov A, Koh CH, et al. A high level of liver-specific expression of oncogenic Kras(V12) drives robust liver tumorigenesis in transgenic zebrafish. Dis Model Mech 2011;4:801-13. [Crossref] [PubMed]
- Glentis A, Oertle P, Mariani P, et al. Cancer-associated fibroblasts induce metalloprotease-independent cancer cell invasion of the basement membrane. Nat Commun 2017;8:924. [Crossref] [PubMed]
- Haraguchi N, Ohara N, Koseki J, et al. High expression of ADAMTS5 is a potent marker for lymphatic invasion and lymph node metastasis in colorectal cancer. Mol Clin Oncol 2017;6:130-4. [Crossref] [PubMed]
- Zhu Z, Xu J, Wu X, et al. In Silico Identification of Contradictory Role of ADAMTS5 in Hepatocellular Carcinoma. Technol Cancer Res Treat 2021;20:1533033820986826. [Crossref] [PubMed]
- Li Y, Wang T, Jiang F. Pan-Cancer Analysis of P3H1 and Experimental Validation in Renal Clear Cell Carcinoma. Appl Biochem Biotechnol 2024;196:5974-93. [Crossref] [PubMed]
- Xiong C, Wang G, Bai D. A novel prognostic models for identifying the risk of hepatocellular carcinoma based on epithelial-mesenchymal transition-associated genes. Bioengineered 2020;11:1034-46. [Crossref] [PubMed]
- Zhang Y, Chen Y, Chen Z, et al. Identification of P3H1 as a Predictive Prognostic Biomarker for Bladder Urothelial Carcinoma Based on the Cancer Genome Atlas Database. Pharmgenomics Pers Med 2023;16:1041-53. [Crossref] [PubMed]
- Finn RS, Qin S, Ikeda M, et al. Atezolizumab plus Bevacizumab in Unresectable Hepatocellular Carcinoma. N Engl J Med 2020;382:1894-905. [Crossref] [PubMed]
- Ren Z, Xu J, Bai Y, et al. Sintilimab plus a bevacizumab biosimilar (IBI305) versus sorafenib in unresectable hepatocellular carcinoma (ORIENT-32): a randomised, open-label, phase 2-3 study. Lancet Oncol 2021;22:977-90. [Crossref] [PubMed]
- Li J, Wang W, Zhou Y, et al. m6A Regulator-Associated Modification Patterns and Immune Infiltration of the Tumor Microenvironment in Hepatocarcinoma. Front Cell Dev Biol 2021;9:687756. [Crossref] [PubMed]
- Hanahan D, Weinberg RA. Hallmarks of cancer: the next generation. Cell 2011;144:646-74. [Crossref] [PubMed]
Cite this article as: Ma Q, Wang Y, Xing J, Wang T, Wang G. Development and validation of a basement membrane-associated immune prognostic model for hepatocellular carcinoma. Transl Gastroenterol Hepatol 2025;10:28.