Pan-gastrointestinal adenocarcinoma analysis uncovers the prognostic and immune correlates of ferroptosis-related genes
Highlight box
Key findings
• A gene set variation analysis (GSVA)-based ferroptosis-score was significantly associated with favorable overall survival in gastrointestinal adenocarcinomas (GIACs).
• The ferroptosis-score was correlated with activation of the DNA damage repair pathway and resistance to cisplatin.
• GIACs with low ferroptosis-scores exhibited heightened expression of immune checkpoint molecules such as programmed death-(ligand) 1 and cytotoxic T lymphocyte antigen-4, elevated densities of tumor-infiltrating CD8+ T cells, and a favorable response to pembrolizumab monotherapy.
What is known and what is new?
• Ferroptosis plays a key role in the development of various tumors and the response to anti-cancer treatments.
• This study explored the potential role of ferroptosis-related genes on the prognosis and treatment of GIACs.
What is the implication, and what should change now?
• The ferroptosis-related signature can potentially change the management of GIAC patients by enabling risk stratification and predicting response to systemic therapy.
• The findings from our study can be applied to further studies and are highly promising for personalized treatment and clinical management.
Introduction
Ferroptosis, an iron-dependent form of oxidative cell death driven by lipid peroxidation, stands out with its distinctive mechanism compared to other regulated cell death modalities like apoptosis, autophagy, or necrosis (1). This process involves the catalysis of lipid peroxidation on cell membranes by ferrous iron or oxygenases, leading to cell death (1,2). The essence of ferroptosis is the peroxidative damage of the cell membrane or organelle membrane caused by the inactivation of the intracellular reducing system, resulting in the rupture of the cell membrane (3).
Current evidence has shown that ferroptosis is involved in cancer development and anti-cancer treatment (4,5). Iron, a fundamental nutrient for human biological functioning (6), when dysregulated, can promote cancer risk and progression (7,8). As for anti-cancer treatment, several studies have identified a critical role of ferroptosis in killing tumor cells and inhibiting tumor growth among multiple cancers, such as non-small cell lung cancer (9,10), ovarian cancer (11), hepatocellular carcinoma (12), and pancreatic cancer (13). Immunotherapy-activated CD8+ T cells enhanced ferroptosis-specific lipid peroxidation in tumor cells and induced ferroptosis, contributing to the anti-tumor efficacy of immunotherapy (14). In addition, chemotherapy can also induce ferroptosis, and the genetic and biochemical hallmarks of ferroptosis were observed in radiation-treated cancer cells (15,16). Combination regimens of chemotherapy and immunotherapy have displayed synergistic effects in enhancing tumor control through mechanisms like downregulating SLC7A11 to promote ferroptosis (17,18). Taken together, ferroptosis plays a key role in tumor development and response to anti-cancer treatments.
Gastrointestinal (GI) cancers are common worldwide with high rates of morbidity and mortality (19). Given that ferroptosis plays an important role in the tumor microenvironment and the development and progression of GI cancers (20-22), we speculated that ferroptosis may have great potential in prognostication and predicting response to immunotherapy in GI cancers. In this study, we aimed to first delineate the genomic and transcriptomic landscapes of the ferroptosis-related genes among GI cancers and then explored their associations with prognosis, cancer-related signaling pathways, tumor immune microenvironment, and immunotherapy efficacy. We present this article in accordance with the STREGA reporting checklist (available at https://tgh.amegroups.com/article/view/10.21037/tgh-24-15/rc).
Methods
Data acquisition and analysis
Genomic, transcriptomic, and clinical data of eight different types of GI cancers were downloaded from The Cancer Genome Atlas (TCGA), including esophageal adenocarcinoma (ESAD), esophagus squamous cell carcinoma (ESSC), pancreatic adenocarcinoma (PAAD), cholangiocarcinoma (CHOL), liver hepatocellular carcinoma (LIHC), colon adenocarcinoma (COAD), rectum adenocarcinoma (READ), and stomach adenocarcinoma (STAD). Moreover, the genomic and transcriptomic data of these types of cancer were retrieved from the Gene Expression Omnibus (GEO) and the Cancer Cell Line Encyclopedia (CCLE) for verifying the results of molecular profiling of ferroptosis-related genes in the TCGA cohort. Only patients with genomic, transcriptomic, and clinical data were included into the analysis.
The ferroptosis-score was trained in the TCGA dataset of gastrointestinal adenocarcinoma (GIAC) (n=828) and validated in GSE19417 (ESAD/STAD, n=64), GSE84437 (STAD, n=433), GSE17536 (COAD, n=177), and GSE103479 (COAD/READ, n=154). The baseline characteristics of the training and validation cohorts are described in the Table S1. Analyses of drug sensitivity and immunotherapy efficacy were implemented in the Genomics of Drug Sensitivity in Cancer (GDSC) dataset, and the PRJEB25780 cohort including 45 patients with advanced STAD treated with pembrolizumab monotherapy, respectively.
The study was conducted in accordance with the Declaration of Helsinki (as revised in 2013).
Identification and functional enrichment of differentially expressed ferroptosis-related genes
The “limma” R package was used to identify the differentially expressed genes (DEGs) between tumor tissues and adjacent non-tumorous tissues in the TCGA cohort, with the criteria of adjusted false discovery rate (FDR) P value<0.05 and |log2[fold change]| >1.
Gene set enrichment analysis (GSEA) was performed to divide the GIAC samples into two groups based on the mRNA expression of ferroptosis-related genes by the R package “ConsensusClusterPlus” (23). Gene set variation analysis (GSVA) can be used to ascribe signaling pathway variation scores to the gene sets to evaluate their biological functions (24). Based on the Z-score transformed from the “fragments per kilobase million (FPKM)” data by the “zFPKM” package, Pearson correlation coefficients (PCC) were calculated between the expression levels of ferroptosis-related genes and the activation levels of hallmark pathways. Associations between genes and hallmark pathways were deemed statistically significant if |PCC| >0.5 and P.adj<0.01, elucidating robust correlations between these entities.
Construction of protein-protein interaction (PPI) network
To predict potential interactions of DEGs, the DEGs were submitted to the Search Tool for the Retrieval of Interacting Genes (version 10.5). Then, the network was visualized by Cytoscape software (version 3.6.0).
Identification of gene expression-based subtypes
Based on the gene expression profile, unsupervised clustering was performed to discover classes by using the “ConsensusClusterPlus” R package (25,26). The clustering approach adopted in this study was based on partitioning around medoids (PAM), a robust method frequently employed for clustering tasks in genomics research.
Survival analysis of ferroptosis-related signature
Ferroptosis-related genes have been classified into distinct categories, categorized as “good” and “bad” predicated on their elevated expression in samples with improved or less favorable prognosis. Then GSVA scores were computed for both groups based on the log2 (FPKM + 1) values of individual genes, employing the GSVA algorithm as described previously. Subsequently, the ferroptosis score is defined as the score of the “good” group minus the score of the “bad” group.
The patients were classified into high- and low-ferroptosis score cohorts according to the median value. Subsequently, the optimal cutoff value for the ferroptosis score was computed. We conducted univariable Cox analysis and Kaplan-Meier survival analysis between the two ferroptosis-score groups via the “survival” R package. Following this, a multivariate Cox regression analysis was employed to assess the independence of the ferroptosis-score as a prognostic factor.
Analysis of the associations of the ferroptosis-score with immune checkpoints, GSVA score, tumor-infiltrating immune cells, and drug sensitivity
To characterize the abundance of immune cells in malignant tissues, we employed the Estimation of STromal and Immune cells in MAlignant Tumor tissues using Expression data (ESTIMATE) approach via the “estimate” R package, along with immune infiltration identification through estimating relative subsets of RNA transcripts (CIBERSORT) method. Single-sample GSEA (ssGSEA) was used to infer the level of infiltrating immune cells (27). The ssGSEA value was normalized to a percentile distribution, where 0 was the minimum value of immune cell abundance, and 1 was the maximum score. The R package “GSEABase” with 29 immunity-related signatures was employed to predict the relative enrichment of tumor-infiltrating immune cells.
Based on the Genomics of Drug Sensitivities in Cancer (GDSC) database, we calculated the correlations (Spearman correlation analysis) of the half maximal (50%) inhibitory concentration (IC50) with the ferroptosis-score. The results were obtained with the “pRRophetic” and “ggplot2” R package. P values were adjusted by the FDR method.
Statistical analysis
All statistical analyses were performed with R software (Version 4.1.3), SPSS (Version 23.0), and GraphPad Prism (Version 8). Wilcox test was used to analyze the association between the ferroptosis-related gene signature and immune characteristics. Kaplan-Meier analyses with log-rank tests were performed to assess survival differences between groups. Univariable and multivariable Cox regression analyses were conducted to determine factors with a prognostic value. If not specified, a P value <0.05 was considered statistically significant.
Results
Genomic and transcriptomic alterations of ferroptosis-related genes in different cancers
Based on the previous studies (28-30), 59 ferroptosis-related genes were selected for the following analyses in our study (Figure 1A), which were categorized into three groups: 35 as drivers, 22 as suppressors, and 2 as dual-role genes in ferroptosis (31-33). The frequencies of copy number variations (CNV) and somatic mutation of ferroptosis-related genes are shown in Figure 1B,1C, respectively. Of note, SQLE and TFRC had the highest frequency of amplification across the eight cancer types, and the mutational frequencies of all 59 genes except TP53 were below 10%, which was consistent with the results from the cancer cell lines of CCLE (Figure S1).
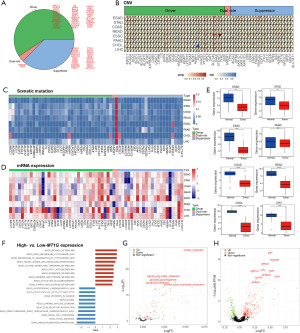
As for mRNA expression, the difference between cancer and normal tissues from the TCGA and the GEO datasets were shown in Figure 1D and Figure S2A. In particular, MT1G expression was lower in tumor samples among all GI cancers (Figure 1E and Figure S2B), which suggests the tumor-suppressive role of MTIG. Compared to the GI tumor samples with lower MT1G expression (below median value), those with higher MT1G expression had higher scores of the signatures related to retinol metabolism, cytochrome metabolism, and xenobiotics metabolism pathways and lower scores of the signatures related to focal adhesion and ubiquitin-mediated proteolysis pathways (Figure 1F). Given the association of MT1G with metabolism pathways, we further compared the scores of metabolism-related genes between the high- and low-MT1G expression groups. The expression of MT1G was up-regulated in the cancer-related pathways, such as glycolysis and gluconeogenesis pathway (Figure 1G), which indicated that the ferroptosis-related genes may be involved in cancer cell plasticity and tumor growth among the different cancer types. Besides, the metabolism-related genes (e.g., GPT, ACADS, PCK1, CTH) were significantly up-regulated in these cancers (Figure 1H).
Biological relevance of ferroptosis-related genes in GIAC
To further clarify the biological relevance of ferroptosis-related genes, the correlation between the expression of individual genes of the signature and the activity of cancer-related pathways was calculated. The correlation between gene expression and the activation or inhibition of multiple cancer-related pathways is illustrated in Figure 2A,2B, indicating the potential involvement of ferroptosis in the tumorigenesis of GIACs. Based on the pairwise correlations, there were more positive correlations than negative correlations among the 59 ferroptosis-related genes (Figure 2C). In the PPI network, the drivers, suppressors, and dual-function ferroptosis genes exhibited frequent interactions with each other (Figure 2D). This interplay underscores the intricate connections and potential crosstalk between different classes of ferroptosis-related genes, suggesting a complex regulatory network underlying the process of ferroptosis and its implications in cancer biology.
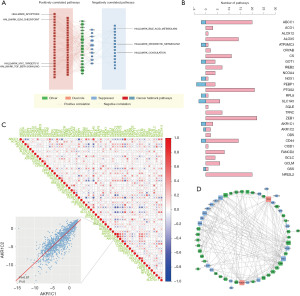
Additionally, we compared the association of the 59 ferroptosis-related genes with the proportion of leukocytes among 8 cancers. Some ferroptosis-related genes (e.g., ALOX12) were up-regulated in ESAD, STAD, COAD, and READ, while down-regulated in ESCC, PAAD, CHOL, and LUSC (Figure 3A). Considering the pivotal role of the adaptive/innate immune system in tumorigenesis, we analyzed the association between the ferroptosis-related genes and the score of Reactome adaptive/innate immune system pathways. Although there were differences between these cancers, these ferroptosis-related genes were associated with more than one cancer (e.g., HMOX1, CD44, and ACSL4, Figure 3B), suggesting that the expression of ferroptosis-related genes was associated with the immune response process. Recent evidence has demonstrated the prognostic value of the mRNA expression-based stemness index (mRNAsi) in cancers (34,35). We calculated the correlation between the expression of ferroptosis-related genes and the score of mRNAsi. Most of the ferroptosis-related genes were positively correlated with the mRNAsi (Figure 3C). Additionally, the correlations of the ferroptosis-related genes with tumor mutation burden (TMB) were significant among GIAC (Figure 3D).
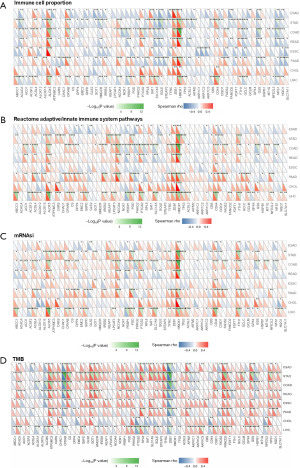
Taken together, ferroptosis-related genes showed similar expression patterns in GIAC. Besides, GIAC of the tubular GI tract including esophagus, stomach, colon, and rectum comprise most GI cancers and share a spectrum of genomic features (36). This led us to perform a comprehensive analysis of the different types of GIAC.
Construction and validation of the prognostic model based on ferroptosis-related genes
To evaluate the potential prognostic role of the ferroptosis-related genes in patients with GIAC, we further assessed the overall survival (OS) of the signature in four cancers (COAD, ESAD, READ, and STAD). Unsupervised clustering based on the mRNA levels of the ferroptosis-related genes classified all GIAC samples into 2 clusters (Figure 4A). The cluster 1 (C1) group had a better OS than those of the cluster 2 (C2) group [P<0.001; hazard ratio (HR), 0.58, confidence interval (95% CI): 0.40–0.83; Figure 4B]. A total of 33 ferroptosis-related genes, exhibiting higher expression in C1 associated with improved OS, were defined as “good” genes, while 8 genes showing increased expression in C2 were classified into the “bad” group. Additionally, 18 genes displaying no significant expression difference between the two groups were excluded from the ferroptosis-score calculation (Table S2). The ferroptosis-related genes with higher expression in clusters with longer OS were called “good” genes, or else they were called “bad” genes. The ferroptosis score is defined as the score of the “good” group minus the score of the “bad” group. Cases of a high-ferroptosis score had a better OS than those of a low-ferroptosis score (P=0.003; HR, 0.67, 95% CI: 0.52–0.87; Figure 4C). In the validation cohort [GSE17536 (COAD), GSE19417 (ESAD/STAD), GSE84437 (STAD), GSE103479 (COAD/READ)], a high ferroptosis-score was associated with better OS (GSE17536: P=0.03; HR, 0.57, 95% CI: 0.34–0.96; GSE19417: P=0.047; HR, 0.53, 95% CI: 0.28–1.01; GSE84437: P=0.004; HR, 0.68, 95% CI: 0.51–0.90; GSE103479: P=0.03; HR, 0.55, 95% CI: 0.32–0.96; Figure 4D). In addition, the ferroptosis-score was determined to be an independent predictor of survival analysis by multivariable Cox regression analysis in the TCGA (P=0.04; HR, 0.75, 95% CI: 0.57–0.98; Table S3) and GEO cohorts (GSE17536: P=0.03; HR, 0.56, 95% CI: 0.33–0.94; GSE19417: P=0.05; HR, 0.53, 95% CI: 0.28–1.01; GSE84437: P=0.009; HR, 0.67, 95% CI: 0.52–0.91 GSE103479: P=0.009; HR, 0.47, 95% CI: 0.27–0.83; Table S3), adjusted for age, sex, tumor stage and cancer types in multivariable models. We then verified that there was no difference in tumor stage and cancer types between clusters with high- and low-ferroptosis scores (Figure S3).
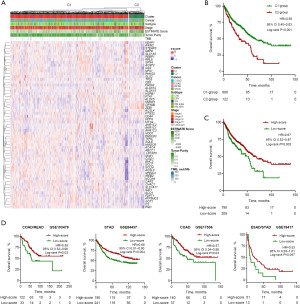
Associations of the ferroptosis-score with treatment responses
Given the relationship between the ferroptosis-related genes and immune features, we further explored the associations of the ferroptosis-score with treatment responses. The correlation between the ferroptosis-score and immune checkpoints [cytotoxic T lymphocyte antigen-4 (CTLA4), T cell immunoglobulin mucin 3 (TIM-3), lymphocyte activation gene-3 (LAG3), and T cell immunoreceptor with Ig and ITIM domains (TIGIT), etc.] was performed, indicating the high expression of immune checkpoints was observed in the low ferroptosis-score group (Figure 5A). The ferroptosis-score was negatively correlated with the GSVA score of the immune-related pathways, such as programmed death-1 (PD-1) signaling (Figure 5B).
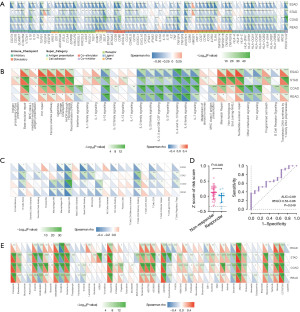
Next, we estimated the associations of the ferroptosis-score with the absolute density of 22 types of immune cells and response to pembrolizumab in patients with advanced/metastatic STAD. A low ferroptosis-score was correlated with high infiltration levels of CD8+ T cells and several immunosuppressive cells, e.g., M2 macrophage (P<0.001), resting memory CD4+ T cell (P<0.01), and naïve B cells (P<0.01, Figure 5C). In addition, patients who responded to immunotherapy had a lower score compared to the non-responders in the PRJEB25780 cohort (P=0.049, AUC =0.69, 95% CI: 0.53–0.86; Figure 5D). These results indicated the association of the high ferroptosis-score group with a poor response to immunotherapy.
We also analyzed the associations between the ferroptosis-score and the anti-tumor efficacy of multiple chemicals in the GIAC cell lines. As for chemotherapeutic drugs, a low ferroptosis-score was associated with increased sensitivities to lapatinib, sorafenib, and paclitaxel, etc. (Figure 5E). Taken together, these results may indicate the potential effect of the ferroptosis-score in predicting response to systemic therapy in the GIAC.
Discussion
In our study, we first described the genomic and transcriptomic alterations of ferroptosis-related genes in different cancers. Based on the mRNA expression of 59 ferroptosis-related genes, we explored the biological relevance of ferroptosis-related genes in GIACs. We further constructed the ferroptosis-score for predicting the prognosis in GIAC patients, which was validated in 4 independent GEO cohorts. As for predicting treatment responses, the low ferroptosis-score group had lower expression levels of immune checkpoints, higher infiltration levels of CD8+ T cells, a better response to immunotherapy, and higher sensitivities to several anti-tumor drugs (e.g., lapatinib, sorafenib, and cisplatin), compared to the high ferroptosis-score group.
Data from TCGA and GEO showed a significant difference in the mRNA expression of ferroptosis-related genes between normal and cancer tissues, and this difference was validated in cancer and non-cancer cell lines, which demonstrated that ferroptosis may be involved in the initiation and development of GI cancers. Interestingly, MT1G, as a key ferroptosis-related gene (37), was lowly expressed across multiple GI cancers. In hepatocellular carcinoma cells, the knockdown of MT1G could induce ferroptosis and thereby restore the sensitivity to sorafenib, a tyrosine kinase inhibitor mainly targeting vascular endothelial growth factor receptor (VEGFR) (38), indicating the potential association between ferroptosis and anti-angiogenic therapies. Given the wide adoption of anti-angiogenic agents like bevacizumab, ramucirumab, regorafenib, and lapatinib in the management of GI cancers (39-41), our results hint at the potential of ferroptosis in forecasting responses to anti-angiogenic treatments in this context. These findings advocate for further investigations into the role of ferroptosis in predicting responses to anti-angiogenic therapies in GI cancers, providing a promising avenue for future research endeavors.
Prognosis prediction of cancer is critical for improving the survival rate of patients, as it can aid the subsequent clinical management of patients. In our study, the ferroptosis-score based on mRNA expression of 41 genes could effectively predict the prognosis of GIAC patients, which was validated in multiple independent cohorts. This ferroptosis-score not only effectively predicted the prognosis of GIAC patients, but could be further used to explore the relationship between ferroptosis and other clinical characteristics in GIAC prognosis. The prognostic effect of this score was independent of many clinical characteristics, such as age, sex, and TNM stage, suggesting that ferroptosis-score could be used in combination with other prognostic indicators to optimize existing clinical prognostic models. Currently, quantitative polymerase chain reaction (qPCR) emerges as a favored method for precise, rapid, and cost-effective gene detection (42), which is suitable for evaluating the expression patterns of the 41 ferroptosis-related genes. Leveraging qPCR promises a feasible approach to assess the ferroptosis-related risk profiles in patients, alongside with our validated ferroptosis-score algorithm. This amalgamation of techniques holds great promise for individualized risk stratification in clinical practice, ultimately empowering clinicians to make informed prognostic decisions for each patient. To be specific, patients with higher ferroptosis-scores are tend to have worse prognosis, which implies that aggressive surgical treatment, as well as more focused medication, including adjuvant, neoadjuvant, and other therapies can be considered in these patients. Nevertheless, further clinical research is imperative to determine a universally applicable cut-off value for ferroptosis score-based risk stratification. Comprehensive clinical implementation warrants further validation in prospective cohorts to consolidate the robustness and reliability of our findings.
Immunotherapy is an emerging novel treatment for GI cancers (43). Immune-infiltrating cells were widely considered to be central players in the initiation and execution of anti-cancer immune responses driven by immunotherapy (44). Our findings suggested the association between a low ferroptosis-score and elevated levels of tumor-infiltrating CD8+ T cells, accompanied with increased expression of immune checkpoint molecules. This implies that individuals with low ferroptosis-scores may experience immune escape mediated by immune checkpoint pathways (45) despite the cytotoxic activity by CD8+ T cells, consequently contributing to an unfavorable prognosis. Besides, previous studies have linked PD-L1 overexpression with poorer prognosis in GC (46) and CRC (47) despite subsequent chemotherapies, coincides with the poorer OS observed in patients with lower ferroptosis-scores, hinting at a limited efficacy of traditional medications in these cases. Furthermore, we also observed a heightened response to pembrolizumab in these patients, aligning with the prevailing consensus correlating immune checkpoint expression with outcomes in immunotherapy (48). Notably, the cohorts we analyzed did not receive immunotherapy or other anti-tumor therapies except for chemotherapy, therefore the potential therapeutic benefits were not captured in the prognostic results. Nevertheless, the existing literature on the interplay between the tumor immune microenvironment and susceptibility to chemotherapy and targeted therapies remains limited. This gap in knowledge underscores the need for further investigations to elucidate the heightened sensitivity to other anti-tumor drugs observed in patients with lower ferroptosis-scores.
A positive correlation between the ferroptosis-score and the activation of the DNA damage repair (DDR) pathway was revealed in our study, suggesting that the GIACs with a high ferroptosis-score may have high DNA repair capacity and thereby might be insensitive to platinum-based chemotherapy. We further observed the insensitivity to cisplatin in the GIAC cell lines with a high ferroptosis-score. Nowadays, the combination of immune checkpoint inhibitors (ICI) and platinum-based chemotherapy is recommended for advanced/metastatic ESAD and STAD (49,50). In our study, the predictive roles of the ferroptosis-score for platinum-based agent and ICI were consistent, suggesting the predictive power of the ferroptosis-score for the efficacy of the combination therapy of platinum-based agent and ICI.
As for limitations, first, the ferroptosis-related signature merely came from retrospective analysis, and more prospective data are needed to prove the clinical utility. Second, the association between the ferroptosis-related score and drug sensitivities was explored in cancer cell lines, which requires further validation in animal models. Third, due to the limited source of data, we did not analyze the benefit of immunotherapy in ESAD, COAD, and READ. Despite this, the associations of the ferroptosis-score with the expression of immune checkpoints, immune cell infiltration, and the activation of immune-related pathways were highly consistent among GIACs, indicating that the ferroptosis-score might also be useful in predicting immunotherapy efficacy in the GIACs besides STAD.
Conclusions
In conclusion, we systematically described the biological and clinical relevance of ferroptosis-associated signature in GIAC. Additionally, the score of this model was associated with treatment responses. The ferroptosis-related signature can potentially change the management of GIAC patients by enabling risk stratification and predicting response to systemic therapy. The findings from our study can be applied to further studies and are highly promising for personalized treatment and clinical management.
Acknowledgments
None.
Footnote
Reporting Checklist: The authors have completed the STREGA reporting checklist. Available at https://tgh.amegroups.com/article/view/10.21037/tgh-24-15/rc
Peer Review File: Available at https://tgh.amegroups.com/article/view/10.21037/tgh-24-15/prf
Funding: This work was funded by
Conflicts of Interest: All authors have completed the ICMJE uniform disclosure form (available at https://tgh.amegroups.com/article/view/10.21037/tgh-24-15/coif). W.C., Y.X., Q.Z., T.Z., G.W., and S.C. are employees of Burning Rock Biotech. The other authors have no conflicts of interest to declare.
Ethical Statement: The authors are accountable for all aspects of the work in ensuring that questions related to the accuracy or integrity of any part of the work are appropriately investigated and resolved. The study was conducted in accordance with the Declaration of Helsinki (as revised in 2013).
Open Access Statement: This is an Open Access article distributed in accordance with the Creative Commons Attribution-NonCommercial-NoDerivs 4.0 International License (CC BY-NC-ND 4.0), which permits the non-commercial replication and distribution of the article with the strict proviso that no changes or edits are made and the original work is properly cited (including links to both the formal publication through the relevant DOI and the license). See: https://creativecommons.org/licenses/by-nc-nd/4.0/.
References
- Chen F, Kang R, Tang D, et al. Ferroptosis: principles and significance in health and disease. J Hematol Oncol 2024;17:41. [Crossref] [PubMed]
- Bell HN, Stockwell BR, Zou W. Ironing out the role of ferroptosis in immunity. Immunity 2024;57:941-56. [Crossref] [PubMed]
- von Krusenstiern AN, Robson RN, Qian N, et al. Identification of essential sites of lipid peroxidation in ferroptosis. Nat Chem Biol 2023;19:719-30. [Crossref] [PubMed]
- Mao C, Liu X, Zhang Y, et al. DHODH-mediated ferroptosis defence is a targetable vulnerability in cancer. Nature 2021;593:586-90. [Crossref] [PubMed]
- Diao J, Jia Y, Dai E, et al. Ferroptotic therapy in cancer: benefits, side effects, and risks. Mol Cancer 2024;23:89. [Crossref] [PubMed]
- Berndt C, Alborzinia H, Amen VS, et al. Ferroptosis in health and disease. Redox Biol 2024;75:103211. [Crossref] [PubMed]
- Lei G, Zhuang L, Gan B. The roles of ferroptosis in cancer: Tumor suppression, tumor microenvironment, and therapeutic interventions. Cancer Cell 2024;42:513-34. [Crossref] [PubMed]
- Zhou Q, Meng Y, Li D, et al. Ferroptosis in cancer: From molecular mechanisms to therapeutic strategies. Signal Transduct Target Ther 2024;9:55. [Crossref] [PubMed]
- Tian HX, Mei J, Cao L, et al. Disruption of Iron Homeostasis to Induce Ferroptosis with Albumin-Encapsulated Pt(IV) Nanodrug for the Treatment of Non-Small Cell Lung Cancer. Small 2023;19:e2206688. [Crossref] [PubMed]
- Yu J, Zhong B, Zhao L, et al. Fighting drug-resistant lung cancer by induction of NAD(P)H:quinone oxidoreductase 1 (NQO1)-mediated ferroptosis. Drug Resist Updat 2023;70:100977. [Crossref] [PubMed]
- Wu N, Zhang X, Fang C, et al. Progesterone Enhances Niraparib Efficacy in Ovarian Cancer by Promoting Palmitoleic-Acid-Mediated Ferroptosis. Research (Wash D C) 2024;7:0371.
- Ding Z, Pan Y, Shang T, et al. URI alleviates tyrosine kinase inhibitors-induced ferroptosis by reprogramming lipid metabolism in p53 wild-type liver cancers. Nat Commun 2023;14:6269. [Crossref] [PubMed]
- Schneider C, Hilbert J, Genevaux F, et al. A Novel AMPK Inhibitor Sensitizes Pancreatic Cancer Cells to Ferroptosis Induction. Adv Sci (Weinh) 2024;11:e2307695. [Crossref] [PubMed]
- Zhao L, Zhou X, Xie F, et al. Ferroptosis in cancer and cancer immunotherapy. Cancer Commun (Lond) 2022;42:88-116. [Crossref] [PubMed]
- Kciuk M, Gielecińska A, Kałuzińska-Kołat Ż, et al. Ferroptosis and cuproptosis: Metal-dependent cell death pathways activated in response to classical chemotherapy - Significance for cancer treatment? Biochim Biophys Acta Rev Cancer 2024;1879:189124. [Crossref] [PubMed]
- Ning J, Chen L, Zeng Y, et al. The scheme, and regulative mechanism of pyroptosis, ferroptosis, and necroptosis in radiation injury. Int J Biol Sci 2024;20:1871-83. [Crossref] [PubMed]
- Wang W, Green M, Choi JE, et al. CD8(+) T cells regulate tumour ferroptosis during cancer immunotherapy. Nature 2019;569:270-4. [Crossref] [PubMed]
- Wang S, Guo Q, Zhou L, et al. Ferroptosis: A double-edged sword. Cell Death Discov 2024;10:265. [Crossref] [PubMed]
- Rauth S, Malafa M, Ponnusamy MP, et al. Emerging Trends in Gastrointestinal Cancer Targeted Therapies: Harnessing Tumor Microenvironment, Immune Factors, and Metabolomics Insights. Gastroenterology 2024;167:867-84. [Crossref] [PubMed]
- Le J, Pan G, Zhang C, et al. Targeting ferroptosis in gastric cancer: Strategies and opportunities. Immunol Rev 2024;321:228-45. [Crossref] [PubMed]
- Li R, Wu Y, Li Y, et al. Targeted regulated cell death with small molecule compounds in colorectal cancer: Current perspectives of targeted therapy and molecular mechanisms. Eur J Med Chem 2024;265:116040. [Crossref] [PubMed]
- Zhang H, Zhang J, Luan S, et al. Unraveling the Complexity of Regulated Cell Death in Esophageal Cancer: from Underlying Mechanisms to Targeted Therapeutics. Int J Biol Sci 2023;19:3831-68. [Crossref] [PubMed]
- Subramanian A, Tamayo P, Mootha VK, et al. Gene set enrichment analysis: a knowledge-based approach for interpreting genome-wide expression profiles. Proc Natl Acad Sci U S A 2005;102:15545-50. [Crossref] [PubMed]
- Hu J, Tzeng JY. Integrative gene set analysis of multi-platform data with sample heterogeneity. Bioinformatics 2014;30:1501-7. [Crossref] [PubMed]
- Wilkerson MD, Hayes DN. ConsensusClusterPlus: a class discovery tool with confidence assessments and item tracking. Bioinformatics 2010;26:1572-3. [Crossref] [PubMed]
- Wu F, Chai RC, Wang Z, et al. Molecular classification of IDH-mutant glioblastomas based on gene expression profiles. Carcinogenesis 2019;40:853-60. [Crossref] [PubMed]
- Shen S, Wang G, Zhang R, et al. Development and validation of an immune gene-set based Prognostic signature in ovarian cancer. EBioMedicine 2019;40:318-26. [Crossref] [PubMed]
- Liang JY, Wang DS, Lin HC, et al. A Novel Ferroptosis-related Gene Signature for Overall Survival Prediction in Patients with Hepatocellular Carcinoma. Int J Biol Sci 2020;16:2430-41. [Crossref] [PubMed]
- Sun X, Niu X, Chen R, et al. Metallothionein-1G facilitates sorafenib resistance through inhibition of ferroptosis. Hepatology 2016;64:488-500. [Crossref] [PubMed]
- Sun X, Ou Z, Chen R, et al. Activation of the p62-Keap1-NRF2 pathway protects against ferroptosis in hepatocellular carcinoma cells. Hepatology 2016;63:173-84. [Crossref] [PubMed]
- Stockwell BR, Jiang X, Gu W. Emerging Mechanisms and Disease Relevance of Ferroptosis. Trends Cell Biol 2020;30:478-90. [Crossref] [PubMed]
- Doll S, Freitas FP, Shah R, et al. FSP1 is a glutathione-independent ferroptosis suppressor. Nature 2019;575:693-8. [Crossref] [PubMed]
- Wang Y, Wei Z, Pan K, et al. The function and mechanism of ferroptosis in cancer. Apoptosis 2020;25:786-98. [Crossref] [PubMed]
- Chen E, Zou Z, Wang R, et al. Predictive value of a stemness-based classifier for prognosis and immunotherapy response of hepatocellular carcinoma based on bioinformatics and machine-learning strategies. Front Immunol 2024;15:1244392. [Crossref] [PubMed]
- Fu S, Tan Z, Shi H, et al. Development of a stemness-related prognostic index to provide therapeutic strategies for bladder cancer. NPJ Precis Oncol 2024;8:14. [Crossref] [PubMed]
- Pan J, Silva TC, Gull N, et al. Lineage-Specific Epigenomic and Genomic Activation of Oncogene HNF4A Promotes Gastrointestinal Adenocarcinomas. Cancer Res 2020;80:2722-36. [Crossref] [PubMed]
- Cheng B, Lai Y, Huang H, et al. MT1G, an emerging ferroptosis-related gene: A novel prognostic biomarker and indicator of immunotherapy sensitivity in prostate cancer. Environ Toxicol 2024;39:927-41. [Crossref] [PubMed]
- Pandey P, Elsori D, Kumar R, et al. Ferroptosis targeting natural compounds as a promising approach for developing potent liver cancer agents. Front Pharmacol 2024;15:1399677. [Crossref] [PubMed]
- Saeed A, Park R, Sun W. The integration of immune checkpoint inhibitors with VEGF targeted agents in advanced gastric and gastroesophageal adenocarcinoma: a review on the rationale and results of early phase trials. J Hematol Oncol 2021;14:13. [Crossref] [PubMed]
- Li H, Shen M, Wang S. Current therapies and progress in the treatment of advanced gastric cancer. Front Oncol 2024;14:1327055. [Crossref] [PubMed]
- Corrias G, Lai E, Ziranu P, et al. Prediction of Response to Anti-Angiogenic Treatment for Advanced Colorectal Cancer Patients: From Biological Factors to Functional Imaging. Cancers (Basel) 2024;16:1364. [Crossref] [PubMed]
- de Souza da Fonseca A, de Souza Alves E, de Paoli F, et al. Low-power therapeutic lasers on mRNA levels. Lasers Med Sci 2022;37:2353-62. [Crossref] [PubMed]
- Gervaso L, Ciardiello D, Oliveira RA, et al. Immunotherapy in the neoadjuvant treatment of gastrointestinal tumors: is the time ripe? J Immunother Cancer 2024;12:e008027. [Crossref] [PubMed]
- Hu M, Deng F, Song X, et al. The crosstalk between immune cells and tumor pyroptosis: advancing cancer immunotherapy strategies. J Exp Clin Cancer Res 2024;43:190. [Crossref] [PubMed]
- Lin X, Kang K, Chen P, et al. Regulatory mechanisms of PD-1/PD-L1 in cancers. Mol Cancer 2024;23:108. [Crossref] [PubMed]
- Fan Y, Che X, Qu J, et al. Exosomal PD-L1 Retains Immunosuppressive Activity and is Associated with Gastric Cancer Prognosis. Ann Surg Oncol 2019;26:3745-55. [Crossref] [PubMed]
- Yang L, Xue R, Pan C. Prognostic and clinicopathological value of PD-L1 in colorectal cancer: a systematic review and meta-analysis. Onco Targets Ther 2019;12:3671-82. [Crossref] [PubMed]
- Doroshow DB, Bhalla S, Beasley MB, et al. PD-L1 as a biomarker of response to immune-checkpoint inhibitors. Nat Rev Clin Oncol 2021;18:345-62. [Crossref] [PubMed]
- Wang FH, Zhang XT, Tang L, et al. The Chinese Society of Clinical Oncology (CSCO): Clinical guidelines for the diagnosis and treatment of gastric cancer, 2023. Cancer Commun (Lond) 2024;44:127-72. [Crossref] [PubMed]
- Ajani JA, D'Amico TA, Bentrem DJ, et al. Esophageal and Esophagogastric Junction Cancers, Version 2.2023, NCCN Clinical Practice Guidelines in Oncology. J Natl Compr Canc Netw 2023;21:393-422. [Crossref] [PubMed]
Cite this article as: Dong X, Xie Y, Chen W, He M, Liu H, Wang B, Xu Y, Zhou Q, Zhu T, Wang G, Xu C, Wang W, Cai S, Xu M, Wang J. Pan-gastrointestinal adenocarcinoma analysis uncovers the prognostic and immune correlates of ferroptosis-related genes. Transl Gastroenterol Hepatol 2025;10:7.