Predicting waitlist dropout in hepatocellular carcinoma: a narrative review
Introduction
Hepatocellular carcinoma (HCC) is the sixth most common cancer and the third leading cause of cancer-related mortality worldwide. The most frequent causes of HCC are liver cirrhosis with alcoholic and viral etiology [hepatitis B virus (HBV)], although non-alcoholic fatty liver disease (NAFLD) etiology has been increasing in recent years. Among all available treatments, liver transplantation (LT) is the gold standard if patients meet specific established criteria.
The imbalance between available organs and recipients on the waiting list requires an appropriate prioritization among recipients. The current allocation systems are based on the Model of End-Stage Liver Disease (MELD) score and its modifications, which prioritize patients based on the principle of urgency (Sickest-first). However, there is a group of indications such as HCC or metabolic diseases in which the waitlist drop-out risk or death is not determined by liver function. Hence, the MELD score fails to prioritize these patients. Patients with HCC can drop out of the waiting list for three main reasons: (I) tumor progression; (II) death on the waiting list; or (III) to develop contraindications to transplantation (1).
According to data from the Organ Procurement and Transplantation Network (OPTN), 1,043 people died while on the waiting list for LT. Of those, only 71 had HCC (including patients with cirrhosis). In contrast, 262 people died due to alcoholic cirrhosis (excluding those with acute alcoholic hepatitis or hepatitis virus) (2). In fact, these patients initially suffered from an increased time on the waiting list as they preserved their liver function. To address this issue, they are granted access to LT through the allocation of standardized exception points (3,4). This system is also implemented for HCC allocation in Europe (5). However, in the United States (US), this scoring system initially conferred an advantage to HCC over non-HCC patients. Several strategies have been proposed to address this issue, such as extra points modifications, postponing the MELD exception by 6 months, and limiting the maximum MELD score to 34 points. Despite these changes, equitable access to transplantation remains elusive (6,7). Although there have been ongoing refinements to the exception point system, patients still receive the same priority for LT, irrespective of their tumor size or biology (8). Therefore, although waitlist mortality exists in these patients, disease progression contributes to higher degree of waitlist dropout.
To avoid disease progression, HCC patients included on waiting list frequently undergo locoregional therapy (LRT) as bridging strategy. One of the goals is to stabilize the tumor and reduce the quantifiable disease load of HCC, enabling them to remain on the waiting list until a suitable graft is available (9,10). The stage of the disease and the probability of being on the waiting list for more than 6 months must also be taken into account when deciding whether to apply locoregional therapies as bridging therapy (11). Patients who underwent a bridging liver transplant exhibited a higher overall survival rate one year after transplantation compared to those who did not. In cirrhotic patients with HCC already within the Milan criteria at diagnosis, it remains uncertain there would be a difference between cases with bridging liver transplant and cases without in terms of dropout rates, disease-free survival, and survival at 3 and 5 years after transplant (12).
There is currently no optimal patient selection system for selecting HCC patients, assigning them an appropriate risk stratification to avoid waitlist dropout. This narrative review provides a summary of the available literature on current prediction models of waitlist dropout in HCC, ranging from statistical to machine learning (ML) algorithms-based models. Figure 1 shows a visual summary of the contribution of different authors to the subject, as well as the methodology and the most frequent variables. We present this article in accordance with the Narrative Review reporting checklist (available at https://tgh.amegroups.com/article/view/10.21037/tgh-24-24/rc).
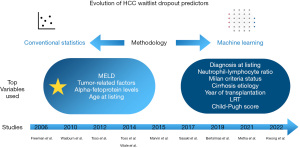
Methods
A review of all available literature in the English language through December 25, 2023, utilizing PubMed database was conducted. All types of studies related to waitlist dropout in HCC were included. All articles that were not based on prediction models based on conventional statistical methods or ML models were excluded. Two authors independently screened articles included. All data related to search strategy summary are shown in Table 1.
Table 1
Items | Specification |
---|---|
Dates of search | 20/10/2023; 25/12/2023 |
Databases searched | PubMed |
Search terms used | Waitlist dropout; HCC; machine learning; predictive factors; prediction models |
Timeframe | All published articles up to December 25, 2023 |
Inclusion and exclusion criteria | All types of studies related to waitlist dropout in HCC were included. All articles that were not based on prediction models based on conventional statistical methods or machine learning models were excluded. Case reports and case series and manuscripts written in languages other than English were also excluded |
Selection process | The selection of articles was conducted by two authors. One author focused on the search for predictive factors and the other on machine learning algorithms related to the topic. Once the search was completed, the opposing author confirmed that the proposed article met the criteria for inclusion in this review. If there was a discrepancy between the two authors, a third author was proposed for the final decision |
HCC, hepatocellular carcinoma.
Prediction models of HCC waitlist dropout
Risk factors for dropout
The major contributor to HCC waitlist dropout is disease progression. Tumor characteristics and time on waitlist have a significant influence on this progression (13-16). For the majority of authors, time on waitlist is the best selector for LT in HCC, selecting patients with low risk of HCC recurrence and better survival rates after LT (17). However, the length of time on the waiting list is a variable that depends on the region where we are, donation policies and access to transplantation. Identifying tumor-related factors that are associated with a higher risk of HCC progression and therefore, waitlist dropout, is an issue of interest.
Tumor size, alpha-fetoprotein (AFP) levels, and response to LRT are significant indicators of biological tumor aggressiveness and the MELD score represents the severity or the underlying liver disease. Tumor diameter or multinodularity were identified as risk factors in several studies (8,13,16-18). It has been known for years that serum AFP levels correlate with a worse prognosis and are also related to tumor size and the likelihood of vascular invasion (19). MELD score is a good predictor of waiting list mortality, and reflects the severity of liver disease. However, as previously mentioned, it has its limitations in these patients. Patients with a high MELD may condition the LRT and therefore increase the likelihood of waitlist dropout. These patients may not be suitable candidates for LRT or receive a non-optimal treatment to avoid hepatic decompensation (3). The consequence is an increase in the risk of tumor progression. Finally, the response to LRT may be an important contributor to the probability of patient waiting list removal (20,21).
However, although the above-mentioned factors can be estimated over a frame-time, in clinical practice they are usually used at a specific time point (22). The advantage of these factors is that they are easy to calculate and their use is not complex. However, it would not be appropriate to use one of them exclusively, as the biology of the tumor and the patient is influenced by several factors. These variables do not provide a continuum of risk, but identifying them has assisted us in improving the decision to prioritize these patients. The most important lesson learned about these markers is that the variations over time offer more value in predicting the risk of mortality or progression on the waiting list than a static knowledge. This is the main reason why these variables constitute a part of the prediction models as they form part of the strongest predictors in many of them. However, models increase in complexity as variables are added, which makes them less attractive.
Statistical models
Understanding which factors contribute significantly to the waitlist dropout is important, but being able to combine them and obtain a prediction model that allows to estimate a risk probability is what makes prediction models attractive. In this concern, selection models for HCC candidates such as the French AFP model or the Metroticket 2.0, are reliable predictors of post-LT outcomes compared to Milan criteria. However, they fail to predict HCC waitlist dropout or progression (23,24).
The first model in the literature related to HCC waitlist dropout was reported by Freeman et al. (25). They analyzed a cohort of patients comprising non-HCC (n=9,379) and HCC patients (n=2,052). A Cox regression model was established, revealing that MELD score at listing was the main predictor of waitlist dropout in both groups. Additionally, factors related to a high dropout rate in non-HCC patients were age and metabolic disease diagnosis. However, in the HCC patient group, the combination of MELD, maximum tumor size, AFP level, and age at listing defined the probability of dropout at 90 days, with an area under the curve (AUC) of 0.781. Indeed, among these factors, the MELD score, maximum tumor size, and AFP level were identified as independent risk factors. The equation 1 − 0.920 exp [0.09369 (MELD at listing 12.48) + 0.00193 (AFP − 97.4) + 0.1505 (maximum tumor size − 2.59) defined the probability of dropout for HCC candidates within 90 days of listing. This study did not achieve an external validation, and the described model did not surpass the MELD score as a single predictive factor, suggesting that the progression of liver disease in the selected patient cohort influenced dropout rates. Nevertheless, due to its relevance, this score was proposed in a national conference as a system to improve the allocation system in HCC candidates (26).
Washburn et al. (27) based on the OPTN data, reported a model based on a Cox regression and a competing risk (CR) analysis. The difference between the two is that while Cox regression considers only one cause as the event of interest, risk competition analysis takes into account that the event under study may be caused by several causes. As a consequence, Cox analysis models tend to reduce the size of the at-risk population and tend to overestimate events rates. The univariate statistical analysis of dropout rates was based on CR and the multivariable analyses of dropout rates were based on the Cox and CR models. The CR methods classified patients in transplanted, dropped out or still waiting. In the Cox analysis, patients were censored for any removal other than death. Cox and CR methods demonstrated that MELD score, tumor size and AFP are the most significant predicting variables, regardless of analysis used. The C-index for predicting dropout was 0.70 for both models. Interestingly, LRT did not influence a lower dropout rate of HCC patients in this cohort, justified by an early access to transplantation due to priority score before tumor progression. However, this model is not as accurate as MELD in predicting death in non-HCC patients (C-index: 0.68). So, the score is not useful in a common waitlist, being a significant limitation.
To solve this issue, Toso et al. (28) proposed a proportional hazard competitive risk model, identifying independent prediction factors such as MELD score, HCC number and size and AFP. The combination of these factors with diagnosis and age at listing variables, allowed to estimate the risk of dropout (C-index 0.72). However, due to the model and MELD score were not comparable scales, a correlation was established between both models (MELD and drop-put equivalent MELD or deMELD). This approach allowed to integrate HCC and non-HCC patients on a common waiting list. The most relevant limitations were: (I) a lack of external validation conditioned by the estimation of deMELD (developed in a specific cohort); (II) recipient age and liver disease were included in this model. These variables were not predictors of risk dropout and including them, may penalize older recipients or i.e., non-alcoholic steatohepatitis (NASH). To overcome these limitations, the author developed a new deMELD model (29), including only tumor-related variables such as size and number of HCC or AFP levels and MELD score. In this model, 49,026 HCC and non-HCC patients were included. The model reached a C-index of 0.66, showing similar distribution as MELD in non-HCC patients and subsequently was externally validated in an US and United Kingdom (UK) cohorts. However, a high proportion of deMELD patients (up to 72%) who are assigned a lower deMELD score compared to their theoretical MELD score was observed. Additionally, this study did not consider the influence of LRT or complications derived from cirrhosis.
Other scores such as MELD-eq (30) or HCC-MELD (31) have tried to calibrate HCC and non-HCC patients. Vitale et al. (31) based their model in the concept of survival benefit. The authors argue that most previous studies had based their models in 3-month dropout risk as end-point. As a result, HCC with higher biological aggressiveness may be prioritized, impacting on surveillance and recurrence. The methodology aims, by performing and integrating several equations, to obtain a “MELD-equivalent” score for HCC patients that equals to patients without HCC in a given MELD subgroup. The equation proposed was HCC-MELD = 1.27 × MELD − 0.51 × logAFP + 4.59. Marvin et al. (30) proposed the MELD-eq model. The final HCC equivalent Model for End-Stage Liver Disease (MELDEQ) score was MELDEQ = max (MELDCALC-EQ, MELDLAB). The results of this study suggest that HCC patients with a MELDEQ score ≤15 would receive advantage compared with patients with MELD scores in a similar range, so a 6-month delay before the granting of exception points would be a satisfactory approach. However, patients with a high MELD-eq score would be penalized by this delay policy. Whereas MELD-eq considers MELD, AFP, tumor number and size, and waitlist time; HCC-MELD considers only MELD and AFP levels. However, if we exclude the inherent limitations of each study, similar to deMELD score, they have a significant barrier in their application. These scores tend to assign lower scores to HCC patients than those obtained with exception points. As a result, their access to transplantation would decrease, penalizing them with longer waiting times and a higher probability of waitlist dropout (22).
The HALT-HCC model proposed by Sasaki et al. (32) was trained on a 420 HCC patient cohort from US and externally validated on a cohort of 13,717 patients from the Scientific Registry of Transplant Recipients (SRTR). The model performance reached a 0.613. One of the advantages of this model is that represents a risk continuum, improving the accuracy due to avoiding variable categorization. However, represents a limitation that the influence of LRT is not included and to extrapolate this model to other regions different to US may be challenge.
The most recent model proposed was published by Mehta et al. (8). The authors hypothesized that the highest risk of HCC dropout is better estimated in long wait time regions (LWR). Once the prediction model was created in these regions, it was applied in mid (MWR) and short wait (SWR) regions. Conclusions were that patients with a risk score >30, have an aggressive tumor biology leading to poor post-LT outcomes. However, in patients stratified under this threshold, the waitlist dropout could be estimated with no differences in post-LT survival. The authors used a cohort of 2,092 LWR, 1,735 SWR and 2,894 MWR patients. SWR and MWR patients were used as validation cohorts. The model developed may be used as continuous risk prediction model (more complex) or a risk stratification score were tumor size, AFP levels or Child-Pugh and MELD score were independent predictors of waitlist dropout. The proposed MELD exception after 6 months on waitlist was ranged in four categories: no MELD exception [>30], median MELD at transplant (MMaT) [21–30], MMaT-3 [8–20] and MMaT-5 [<7]. This model showed a C-index value of 0.74. Nevertheless, this study is not exempt of limitations. The lack of external validation and dynamic data, as well as the lack of variables related to response to LRT in the United Network for Organ Sharing (UNOS) database, and potential under-reporting of HCC recurrence, represent the primary limitations. Nonetheless, the contribution is significant, as the proposed model is easy to use and demonstrates that a higher risk of dropout does not necessarily imply worse outcomes, only within a subgroup of patients. Therefore, the remaining patients may benefit from a risk stratification improving graft allocation. Table 2 summarizes the studies in the literature related to statistical models developed to predict HCC waitlist dropout.
Table 2
Study | Variables | End-point | Dataset | Methodology | Model performance | Limitations | Advantages |
---|---|---|---|---|---|---|---|
Freeman (25) 2006 | MELD score, maximum tumor size, AFP level and age at listing | 90-days dropout | 9,379 non-HCC patients, 2,052 HCC patients | Unadjusted dropout rate was using Kaplan-Meier. Cox regression model to estimate risk factors. Factors were included in an equation | AUC 0.78 | - No external validation | - Lack of previous models |
- Not outperform MELD as an only predictive factor | - Reasonable predictive value for HCC patients meeting Milan criteria | ||||||
Washburn (27) 2010 | MELD score, AFP level and tumor size | Dropout at 30, 60, 90, 180 and 365 days | 4,197 HCC patients, 18,068 non-HCC patients | Competing risk model, Cox regression model | Cox model only MELD =0.68 | - Lack of correlation between HCC and non-HCC candidates | - Cox method overestimate the risk of waitlist dropout for HCC patients |
Cox model [log (AFP), max tumor size, MELD, 3 tumors] =0.70 | - Lack of applicability on a common waiting list | - LRT does not influence dropout | |||||
CR model [log (AFP), max tumor size, MELD] = 0.70 | - AFP as a continuous variable (logarithmically transformed) is strongly associated with HCC dropout | ||||||
Toso (28) 2012 | Age at listing, primary underlying liver disease, MELD score at listing, date of listing, number of tumors, maximum HCC size, total tumor volume and AFP | Dropout at 3-months | A total of 5,498 HCC and 43,528 non-HCC patients were included | Competing risk model, Cox regression model | C-index 0.72 | - Lack of external validation | - Integration patients with HCC and non-HCC on a common and dynamic waiting list |
- Diagnosis at listing and recipient age weren’t predictors of risk dropout | |||||||
Toso (29) 2014 | HCC number and size, AFP level and MELD score | Dropout at 3-months | A total of 4,926 patients with and without HCC were included | Competitive risk model, a new deMELD model | C-index 0.66 | - Failure to consider the influence of LRT and complications arising from cirrhosis | - Only includes tumor-related variables, and therefore more objective and easily measurable information |
- Underestimation of de-MELD values with respect to the corresponding MELD values in 72% of patients | |||||||
- External validation | |||||||
Vitale (31) 2014 | MELD score and AFP level | 5-year transplant benefit | A total of 2,697 patients in WL group and 1,702 in LT group were included | Survival models were calculated by Competing risk model and Cox regression model. Multi-state Markov model and Monte-Carlo simulation were performed to calculate survival benefit | Median 5-year transplant benefit was 15.12 months (8.75–25.35) for the non-HCC patients, and 28.18 months (15.11–36.38) for the T2-HCC patients | - Assignment of lower scores to HCC patients compared to exception points | HCC and non-HCC patients model calibrated according to survival benefit |
- Lack of external validation | |||||||
- Tumor related characteristics not included | |||||||
Marvin (30) 2015 | MELD score, AFP level, HCC number and size | Probability of dropout at 3,6,12-months | 7,491 HCC and 34,310 non-HCC patients | Competing risk model, Cox regression model, MELD score (MELDEQ) was calculated for HCC patients by equating the hazard for wait-list dropout for HCC patients with that for non-HCC patients based on the laboratory MELD | C-index 0.741 | - Assignment of lower scores to HCC patients compared to exception points. | - Attempts to establish an equitable model between HCC and non-HCC patients |
- Lack of external validation | - Patients with a high MELDEQ score are penalized with the 6 months delay policy | ||||||
- Tumor related characteristics are included | |||||||
Sasaki (32) 2017 | MELD-Na, TBS, AFP level, year of transplantation, cause of cirrhosis, neutrophil–lymphocyte ratio, history of LRT and Milan criteria status | NR | A total of 420 patients with HCC were included of a cohort of 13,717 patients | Multivariate Cox regression model | C-index 0.613 | - Lack of external validation outside to ultrasound | - Continuous risk metric |
- Exclusion of LRT influence | - This score could supplant the Milan criteria in assessment of candidacy and allocation preference for LT | ||||||
- This model stratified the risk of mortality for patients within and outside the Milan criteria | |||||||
Mehta (8) 2021 | Tumor size, AFP level, Child-Pugh and MELD score | 1-year dropout risk | A total of 2,092 patients from LWR, 1,735 patients from SWR and 2,894 MRW patients were included | Multivariate Cox regression model | C-index 0.74 | - Lack of external validation | - Simplicity |
- Potential under-reporting of HCC recurrence | - Takes account of regional variations | ||||||
- Higher risk of dropout does not necessarily imply worse outcomes |
HCC, hepatocellular carcinoma; MELD, Model of End-Stage Liver Disease; AFP, alpha-fetoprotein; AUC, area under curve; CR, competing risk; LRT, locoregional therapy; deMELD, drop-put equivalent MELD; WL, waitlist; LT, liver transplant; MELDEQ, Equivalent Model of End-Stage Liver Disease; MELD-Na, MELD-sodium; TBS, tumor burden score; NR, not reported; LWR, long wait time region; SWR, short-wait time region; MWR, mid-wait time region.
ML models
The interest in artificial intelligence and ML has increased in recent years. ML algorithms are able to handle large amounts of data in a fast way, obtaining promising results. In this regard, there are several published papers in hepatology related to imagine-based diagnosis, histology, treatment, prognosis or donor-recipient (D-R) matching in LT (33). However, although the findings are promising, they currently present barriers and limitations conditioning a real applicability.
The publications related to the application of ML algorithms in HCC are related to prevention, diagnosis, prognosis or recurrence (34). However, its application as a predictor of waitlist dropout in HCC is limited. To date, only two authors have developed a specific ML prediction model for waitlist dropout in HCC liver transplant candidates (Table 3) (3,6). Kwong et al. (3) used a 18,920 dataset of HCC liver transplant candidates and 1,181 variables from the OPTN. The end-point considered were 3-, 6-, and 12-month waitlist dropout. They obtained 12 prediction features consisting of 5 variables, combining the top variables identified by a Random Forest (RF) algorithm and a Spearman’s correlation analysis. The variables identified were: maximum and average AFP levels (ng/mL), minimum and average of largest tumor size (cm), minimum and average of serum bilirubin levels (mg/dL), minimum and average of international normalized ratio (INR), maximum and average of different grades of ascites and presence of recent ascites. Finally, these variables were integrated in a Cox proportional hazards model, developing an online calculator. The final model C-index reached a 0.74 value.
Table 3
Study | Variables | End-point | Dataset | Methodology | Model performance | Limitations | Advantages |
---|---|---|---|---|---|---|---|
Bertsimas (6) 2019 | - Albumin level | 3-month waitlist mortality or removal | A total of 1,618,966 HCC and non-HCC patients were included | OCTs. 50% training, 20% validation and 30% test | - OPOM model 0.859 | - Missing values percentage were not reported | - Equalize HCC and non-HCC patients |
- Serum bilirubin | - MELD-Na 0.841 | - Limited differences from MELD-Na and Match MELD in terms of AUC | - Improve waitlist dropout capacity above MELD score especially in sicker patients | ||||
- Serum creatinine | - Match MELD 0.823 | - The LSAM analysis considered is not effective for predicting exit from the waiting list | |||||
- INR | |||||||
- Serum sodium level | - Failure to take into account regional variations | ||||||
- Dialysis in the last week or not | - LRT not included | ||||||
- Number of years the candidate has accrued on the waitlist | |||||||
- Age (years) | |||||||
- Lab MELD score provided by SRTR | |||||||
- Albumin level at previous check in | |||||||
- Serum bilirubin at previous check in | |||||||
- Serum creatinine at previous check in | |||||||
- INR at previous check in | |||||||
- Previous serum sodium at | |||||||
- Previous lab MELD score at | |||||||
- Dyalisis at previous check in or not | |||||||
- Change in bilirubin level since previous check in | |||||||
- Change in creatinine level since previous check in | |||||||
- Change in INR since previous checkin | |||||||
- Change in albumin level sin previous check in | |||||||
- Change in sodium level since previous check in | |||||||
- Change in lab MELD score | |||||||
- Log of the candidate’s bilirubin level | |||||||
- Log of the candidate’s creatinine level | |||||||
- Log of the candidate’s INR | |||||||
- AFP | |||||||
- Number of tumor | |||||||
- Sum of size of tumor | |||||||
Kwong (3) 2022 | - Maximum and average of AFP levels | 3-, 6-, and 12-month waitlist dropout | A total of 18,920 patients with HCC were included | Cox proportional hazards model | - C-index 0.74 | - Lack of external validation | - Simplified prediction model, avoiding traditional machine learning models with a high number of variables |
- Minimum and average of largest tumor size (cm) | The variables included were combined using a Random Forest algorithm and a Spearman correlation analysis | - Non specification of LRT influence | |||||
- Minimum and average of serum bilirubin levels (mg/dL) | - Only includes HCC patients | ||||||
- Minimum and average of INR | |||||||
- Maximum and average of different grade of ascites | |||||||
- Presence of recent ascites |
HCC, hepatocellular carcinoma; OCTs, optimal classification trees; OPOM, Optimized Prediction of Mortality; MELD-Na, MELD-sodium; MELD, Model of End-Stage Liver Disease; AUC, area under curve; LSAM, Liver Simulated Allocation Model; INR, international normalized ratio; LRT, locoregional therapy; SRTR, Scientific Registry of Transplant Recipients; AFP, alpha-fetoprotein.
It is important to mention that the ML algorithm selected (RF) was used as a filter of relevant variables and not as a prediction model itself, unlike other works that have used RF as a prediction algorithm (35). Although the results were satisfactory, the results in terms of C-index compared to other publications in other areas are modest (36). While in other research, ML algorithms outperform traditional scores, in this context, the C-index is similar to the scores published in the literature. Some of the most important limitations of this study are: retrospective nature, lack of external validation and the influence of LRT was not specified. Due to population characteristics used, the authors suggest that this model may be more representative of regions with longer waiting times.
Bertsimas et al. (6) developed the Optimized Prediction of Mortality (OPOM) model based on optimal classification trees (or OCTs). This ML algorithm stands out for its interpretability and accuracy. End-point was the probability of waitlist removal within 3 months (patient death or becoming unsuitable for LT). Twenty-eight variables (Table 3) were used (20 of them associated with MELD score). Two OPOM models were built for HCC and non-HCC patients. This model was integrated into the Liver Simulated Allocation Model (LSAM) to simulate liver allocation replacing MELD score by OPOM model. The conclusions of this work are promising. On the one hand, OPOM is able to improve waitlist dropout capacity above the MELD score, especially in patients who are sicker (up to 16%). On the other hand, this model tends to equalize the priority between HCC and non-HCC candidates, where the extra points allocated traditionally tended to penalize non-HCC patients as these points were based on an arbitrary mortality prediction. However, some limitations must be noted. First, missing value percentages were not reported. Second, although results were satisfactory in terms of AUC (0.859), MELD-sodium (MELD-Na) and Match MELD reached 0.841 and 0.823 respectively, differences were limited. The biggest difference was noted in the cohort of sickest patients. Third, the LSAM analysis considered is effective in predicting waitlist mortality but not in predicting waitlist removal, as this includes both worsening and improving patients. Finally, this model does not consider regional variations in liver graft distribution.
As described in the previous section, a comparative table of the two ML models is presented below.
Limitations and future perspectives
Efforts to improve the prioritization of HCC patients are evident, with the majority of published articles originating from the US. In Europe, most HCC allocation policies are based on extra points assigned by center criteria combined with MELD/MELD like systems. In the US, the current organ allocation system for HCC patients has undergone several modifications over time. After 2015, a 6-month delay in receiving exception points was implemented. Posteriorly, the maximum score these patients could reach was limited to 34 points. The latest proposal has been the inclusion of the MMaT system, which limits the first HCC patient exception score to the MMaT in the area.
All the reviewed articles have contributed to the current evidence but it is necessary to highlight their limitations, which often condition their applicability. First, all models in the studies reviewed are based on retrospective databases. The issue with this type of data is that there may be errors during reporting or a high proportion of missing values, as is the case with the UNOS database (37). A clearer report on the percentage of missing values in different studies would be desirable, especially when referring to ML algorithms. Several ML algorithms are highly sensitive to the presence of missing values. A high proportion of these values influences training and, consequently, the model’s performance (36).
Secondly, only two authors have externally validated their methodology (8,29), and one of them did so in a cohort from their own country (8). The absence of external validation leads most clinicians to question the applicability of these models. However, what we have learned in recent years with the development of ML models is that perhaps we should develop region-specific models rather than universal models (36). Demographic data and access to LT and organ allocation policies vary across different countries. Finally, we must be cautious regarding the obtained results. Most models C-index ranges around 0.7, which is a modest outcome, with only minor differences in some cases compared to the systems they try to overcome. Several reasons such as databases robustness, the inclusion of dynamic variables or variables that are not recorded, such as LRT, may contribute to improving these values.
Reviewing the literature, we can generally divide two groups of works. One group tries to estimate the probability of waitlist dropout based on a continuous risk system or a risk score. The aim is to complement the current organ allocation system. The other group aims to “equalize” the MELD scores of HCC and non-HCC patients so that they can coexist without the need for prioritization rules. However, although this concept is attractive, as affirmed by Norman et al. (22), the consequence of this MELD calibration negatively impacts HCC patients by allocating them fewer points (90% are below 20) than they would receive under the current system, penalizing their access to LT. In this regard, it seems more optimal to adjust prioritization based on the MMaT score.
It is worth to mentioning that various attempts have been made to improve D-R matching in LT based on ML algorithms (36). However, these D-R matching models do not take into account the waitlist dropout risk. Developing new models or adapt the current waitlist dropout prediction models to D-R matching system proposals, can improve them.
Conclusions
Several attempts have been made to find the best model to stratify the risk of waitlist dropout in HCC patients. However, to date, none of the models explored have been implemented. The allocation of these grafts is still based on extra scoring system or geographical criteria. Clearly, factors such as tumor size, AFP levels, MELD score, or LRT response have been shown to have an impact on the disease progression of these patients, conditioning the waitlist dropout. The improvement of methodology and databases in future research is crucial to obtain accurate and reliable models for clinicians. This is the only way to achieve real applicability.
Acknowledgments
Funding: None.
Footnote
Reporting Checklist: The authors have completed the Narrative Review reporting checklist. Available at https://tgh.amegroups.com/article/view/10.21037/tgh-24-24/rc
Peer Review File: Available at https://tgh.amegroups.com/article/view/10.21037/tgh-24-24/prf
Conflicts of Interest: All authors have completed the ICMJE uniform disclosure form (available at https://tgh.amegroups.com/article/view/10.21037/tgh-24-24/coif). The authors have no conflicts of interest to declare.
Ethical Statement: The authors are accountable for all aspects of the work in ensuring that questions related to the accuracy or integrity of any part of the work are appropriately investigated and resolved.
Open Access Statement: This is an Open Access article distributed in accordance with the Creative Commons Attribution-NonCommercial-NoDerivs 4.0 International License (CC BY-NC-ND 4.0), which permits the non-commercial replication and distribution of the article with the strict proviso that no changes or edits are made and the original work is properly cited (including links to both the formal publication through the relevant DOI and the license). See: https://creativecommons.org/licenses/by-nc-nd/4.0/.
References
- Majno P, Giostra E, Morel P, et al. Management of hepatocellular carcinoma in the waiting list before liver transplantation. J Hepatol 2005;42:S134-43. [Crossref] [PubMed]
- National data. OPTN - Organ Procurement and Transplantation Network - HRSA. Available online: https://optn.transplant.hrsa.gov/data/view-data-reports/national-data/
- Kwong A, Hameed B, Syed S, et al. Machine learning to predict waitlist dropout among liver transplant candidates with hepatocellular carcinoma. Cancer Med 2022;11:1535-41. [Crossref] [PubMed]
- Lee HA, Cho EY, Kim TH, et al. Risk Factors for Dropout From the Liver Transplant Waiting List of Hepatocellular Carcinoma Patients Under Locoregional Treatment. Transplant Proc 2018;50:3521-6. [Crossref] [PubMed]
- De Carlis L, Di Sandro S, Centonze L, et al. Liver-allocation policies for patients affected by HCC in Europe. Curr Transplant Rep 2016;3:313-8. [Crossref] [PubMed]
- Bertsimas D, Kung J, Trichakis N, et al. Development and validation of an optimized prediction of mortality for candidates awaiting liver transplantation. Am J Transplant 2019;19:1109-18. [Crossref] [PubMed]
- Heimbach JK, Hirose R, Stock PG, et al. Delayed hepatocellular carcinoma model for end-stage liver disease exception score improves disparity in access to liver transplant in the United States. Hepatology 2015;61:1643-50. [Crossref] [PubMed]
- Mehta N, Dodge JL, Roberts JP, et al. A novel waitlist dropout score for hepatocellular carcinoma - identifying a threshold that predicts worse post-transplant survival. J Hepatol 2021;74:829-37. [Crossref] [PubMed]
- Di Martino M, Vitale A, Ferraro D, et al. Downstaging Therapies for Patients with Hepatocellular Carcinoma Awaiting Liver Transplantation: A Systematic Review and Meta-Analysis on Intention-to-Treat Outcomes. Cancers (Basel) 2022;14:5102. [Crossref] [PubMed]
- Majno P, Lencioni R, Mornex F, et al. Is the treatment of hepatocellular carcinoma on the waiting list necessary? Liver Transpl 2011;17:S98-108. [Crossref] [PubMed]
- Cescon M, Cucchetti A, Ravaioli M, et al. Hepatocellular carcinoma locoregional therapies for patients in the waiting list. Impact on transplantability and recurrence rate. J Hepatol 2013;58:609-18. [Crossref] [PubMed]
- Kostakis ID, Dimitrokallis N, Iype S. Bridging locoregional treatment prior to liver transplantation for cirrhotic patients with hepatocellular carcinoma within the Milan criteria: a systematic review and meta-analysis. Ann Gastroenterol 2023;36:449-58. [Crossref] [PubMed]
- Serenari M, Ratti F, Zanello M, et al. Minimally Invasive Stage 1 to Protect Against the Risk of Liver Failure: Results from the Hepatocellular Carcinoma Series of the Associating Liver Partition and Portal Vein Ligation for Staged Hepatectomy Italian Registry. J Laparoendosc Adv Surg Tech A 2020;30:1082-9. [Crossref] [PubMed]
- Cucchetti A, Cescon M, Bigonzi E, et al. Priority of candidates with hepatocellular carcinoma awaiting liver transplantation can be reduced after successful bridge therapy. Liver Transpl 2011;17:1344-54. [Crossref] [PubMed]
- Faitot F, Allard MA, Pittau G, et al. Impact of clinically evident portal hypertension on the course of hepatocellular carcinoma in patients listed for liver transplantation. Hepatology 2015;62:179-87. [Crossref] [PubMed]
- Mehta N, Dodge JL, Goel A, et al. Identification of liver transplant candidates with hepatocellular carcinoma and a very low dropout risk: implications for the current organ allocation policy. Liver Transpl 2013;19:1343-53. [Crossref] [PubMed]
- Samoylova ML, Dodge JL, Yao FY, et al. Time to transplantation as a predictor of hepatocellular carcinoma recurrence after liver transplantation. Liver Transpl 2014;20:937-44. [Crossref] [PubMed]
- Huang AC, Dodge JL, Yao FY, et al. National Experience on Waitlist Outcomes for Down-Staging of Hepatocellular Carcinoma: High Dropout Rate in All-Comers. Clin Gastroenterol Hepatol 2023;21:1581-9. [Crossref] [PubMed]
- Hakeem AR, Young RS, Marangoni G, et al. Systematic review: the prognostic role of alpha-fetoprotein following liver transplantation for hepatocellular carcinoma. Aliment Pharmacol Ther 2012;35:987-99. [Crossref] [PubMed]
- Otto G, Herber S, Heise M, et al. Response to transarterial chemoembolization as a biological selection criterion for liver transplantation in hepatocellular carcinoma. Liver Transpl 2006;12:1260-7. [Crossref] [PubMed]
- Vitale A, D'Amico F, Frigo AC, et al. Response to therapy as a criterion for awarding priority to patients with hepatocellular carcinoma awaiting liver transplantation. Ann Surg Oncol 2010;17:2290-302. [Crossref] [PubMed]
- Norman J, Mehta N, Kwong A. Optimizing liver transplant prioritization for hepatocellular carcinoma through risk stratification. Curr Opin Organ Transplant 2023;28:265-70. [Crossref] [PubMed]
- Duvoux C, Roudot-Thoraval F, Decaens T, et al. Liver transplantation for hepatocellular carcinoma: a model including α-fetoprotein improves the performance of Milan criteria. Gastroenterology 2012;143:986-94.e3; quiz e14-5. [Crossref] [PubMed]
- Mazzaferro V, Sposito C, Zhou J, et al. Metroticket 2.0 Model for Analysis of Competing Risks of Death After Liver Transplantation for Hepatocellular Carcinoma. Gastroenterology 2018;154:128-39. [Crossref] [PubMed]
- Freeman RB, Edwards EB, Harper AM. Waiting list removal rates among patients with chronic and malignant liver diseases. Am J Transplant 2006;6:1416-21. [Crossref] [PubMed]
- Pomfret EA, Washburn K, Wald C, et al. Report of a national conference on liver allocation in patients with hepatocellular carcinoma in the United States. Liver Transpl 2010;16:262-78. [Crossref] [PubMed]
- Washburn K, Edwards E, Harper A, et al. Hepatocellular carcinoma patients are advantaged in the current liver transplant allocation system. Am J Transplant 2010;10:1643-8. [Crossref] [PubMed]
- Toso C, Dupuis-Lozeron E, Majno P, et al. A model for dropout assessment of candidates with or without hepatocellular carcinoma on a common liver transplant waiting list. Hepatology 2012;56:149-56. [Crossref] [PubMed]
- Toso C, Majno P, Berney T, et al. Validation of a dropout assessment model of candidates with/without hepatocellular carcinoma on a common liver transplant waiting list. Transpl Int 2014;27:686-95. [Crossref] [PubMed]
- Marvin MR, Ferguson N, Cannon RM, et al. MELDEQ : An alternative Model for End-Stage Liver Disease score for patients with hepatocellular carcinoma. Liver Transpl 2015;21:612-22. [Crossref] [PubMed]
- Vitale A, Volk ML, De Feo TM, et al. A method for establishing allocation equity among patients with and without hepatocellular carcinoma on a common liver transplant waiting list. J Hepatol 2014;60:290-7. [Crossref] [PubMed]
- Sasaki K, Firl DJ, Hashimoto K, et al. Development and validation of the HALT-HCC score to predict mortality in liver transplant recipients with hepatocellular carcinoma: a retrospective cohort analysis. Lancet Gastroenterol Hepatol 2017;2:595-603. [Crossref] [PubMed]
- Schattenberg JM, Chalasani N, Alkhouri N. Artificial Intelligence Applications in Hepatology. Clin Gastroenterol Hepatol 2023;21:2015-25. [Crossref] [PubMed]
- Feng S, Wang J, Wang L, et al. Current Status and Analysis of Machine Learning in Hepatocellular Carcinoma. J Clin Transl Hepatol 2023;11:1184-91. [Crossref] [PubMed]
- Lau L, Kankanige Y, Rubinstein B, et al. Machine-Learning Algorithms Predict Graft Failure After Liver Transplantation. Transplantation 2017;101:e125-32. [Crossref] [PubMed]
- Calleja Lozano R, Hervás Martínez C, Briceño Delgado FJ. Crossroads in Liver Transplantation: Is Artificial Intelligence the Key to Donor-Recipient Matching? Medicina (Kaunas) 2022;58:1743. [Crossref] [PubMed]
- Briceño J, Calleja R, Hervás C. Artificial intelligence and liver transplantation: Looking for the best donor-recipient pairing. Hepatobiliary Pancreat Dis Int 2022;21:347-53. [Crossref] [PubMed]
Cite this article as: Calleja R, Aguilera E, Durán M, Pérez de Villar JM, Padial A, Luque-Molina A, Ayllón MD, López-Cillero P, Ciria R, Briceño J. Predicting waitlist dropout in hepatocellular carcinoma: a narrative review. Transl Gastroenterol Hepatol 2024;9:72.