Heart rate variability in acute pancreatitis: a narrative review
Introduction
Acute pancreatitis (AP) refers to an acute inflammation of the pancreas, often presenting classically as a ‘boring’ upper abdominal pain with radiation to the back, epigastric tenderness upon palpation, and fever. Currently, AP afflicts an estimated 33.7 per 100,000 individuals, with a mortality of 1.4 per 100,000 individuals (1). In the United States of America alone, it accounted for over 275,000 admissions, and was estimated to cost USD 2.6 billion per year, resulting in a huge healthcare burden to the country (2). Protracted bouts of AP may result in the sequelae of acute peripancreatic fluid collection, acute necrotic collection, pancreatic pseudocysts, and walled-off pancreatic necrosis. Potential systemic involvement could also result in sepsis, systemic inflammatory response syndrome (SIRS), multiple organ failure and shock, which may manifest as acute respiratory distress syndrome (ARDS) and renal failure (3). Since the progression from mild to severe AP increases the mortality rate significantly to 20–40%, urgent medical intervention is warranted to manage the underlying causes as well as prospective complications in this morbid condition (4-6). As such, the development of a prognostic tool to assess the complications and severity of AP is critical (7).
AP can be broadly categorised based on its severity; the most commonly used system is the 2012 modified Atlanta classification (8), where AP is stratified into mild, moderately severe and severe. Moderately severe AP is defined as the presence of local and/or systemic complications, and/or presence of organ failure not exceeding 48 hours, while severe AP is defined as the presence of persistent organ failure lasting more than 48 hours. In the minority of patients with severe AP, high mortality of 20–40% has been reported (4-6), hence several scoring systems exist to risk identify high-risk patients group for developing severe AP; these include the Acute Physiology and Chronic Health Evaluation II (APACHE II) score (9), Bedside Index of Severity of Acute Pancreatitis (BISAP) (10) and Sequential Organ Failure Assessment (SOFA) (11), which account for clinical and physiological features to prognosticate the disease. Although these scoring systems have been shown to be effective in categorising the risk of AP (12), there are a few drawbacks to each scoring system—cumbersome, requires “invasive” biochemistry investigations and/or advanced radiological imaging with radiation exposure (i.e., computed tomography scan). Furthermore, certain scoring systems do not account for the dynamic nature of AP and the disease evolution may be different from the initial prediction (13). As such, many of these systems though used routinely, the quest for novel, non-invasive, simple, accessible, and affordable prognostic tool continues.
One such suggested tool is heart rate variability (HRV), which is analysed from electrocardiogram (ECG) signals that are constantly monitored. HRV is a metric used to assess modulations in heart rate as it is able to act as a proxy to measure the sympathetic and parasympathetic effects of the autonomic nervous system (ANS) on the heart. Traditionally, HRV was calculated based on statistical operations on RR intervals (time-domain analysis) or spectral analysis of an array of RR intervals (frequency-domain analysis) but these methods are cumbersome and require 24-hour ECG recordings (14). Development in technology has led to the availability of photoplethysmography (PPG)-based devices for HRV measurement, in the form of wearable wrist and/or finger devices (15). PPG-based devices are non-invasive, portable and quick, and hence serve as an attractive bedside risk prediction tool for AP.
HRV was first applied clinically for its use in the prediction of fetal hypoxia, where acute changes in HRV represented fetal distress (16). Its use has however expanded to predict mortality in critically ill patients in intensive care unit (ICU) (17), progression of cardiovascular and neurological conditions, including myocardial infarction, heart failure, atrial fibrillation and Parkinson’s disease, amongst others (18). As AP is also known to influence the modulation by the ANS on the heart, the use of HRV as a risk stratification tool for AP is highly plausible. Recently, Zhang et al. (19) determined that short-term spectral HRV analysis could be used to identify patients with severe AP who are at risk of developing infected pancreatic necrosis (IPN), multi-organ dysfunction syndrome (MODS) and in-hospital mortality. Especially given the ease of assessment of HRV, the exploration of its utility as a prospective diagnostic tool for pancreatitis deserves attention. Therefore, this study aims to synthesize the existing literature regarding the usage of HRV as a tool for the prognostication and monitoring of AP. We present this article in accordance with the Narrative Review reporting checklist (available at https://tgh.amegroups.com/article/view/10.21037/tgh-24-22/rc).
Methods
We performed a literature search on PubMed, Cochrane Central Register of Controlled Trials (CENTRAL), Web of Science, Scopus and Embase, from inception till December 17, 2023. The following search terms were used: “acute pancreatitis”, “heart rate variability”, “infected pancreatic necrosis”, “systemic inflammatory response syndrome”, “sepsis”, “multiple organ dysfunction syndrome”, “acute respiratory distress syndrome”. Table 1 summarizes the search strategy for this review. Articles with mentions of AP and HRV were reviewed, and the complications of AP and its effects on HRV parameters are highlighted in subsequent segments.
Table 1
Items | Specification |
---|---|
Date of search | 17 December, 2023 |
Databases searched | PubMed, Cochrane Central Register of Controlled Trials (CENTRAL), Web of Science, Scopus and Embase |
Search terms used | “acute pancreatitis”, “heart rate variability”, “infected pancreatic necrosis”, “systemic inflammatory response syndrome”, “sepsis”, “multiple organ dysfunction syndrome”, “acute respiratory distress syndrome” |
Timeframe | Database inception to 17 December, 2023 |
Inclusion and exclusion criteria | All article types relevant to heart rate variability in acute pancreatitis and its complications were included |
Selection process | The search and selection of articles were conducted by primary authors M.Y.Q.L., J.Y.J.L. and S.V.S. These were then reviewed by senior authors K.S.C. and V.G.S. |
Physiology of HRV
The heart is driven by a cardiac rhythm, generated by sinus impulses from the sinoatrial node. The cardiac rhythm is modulated by various factors in response to different stimuli. In this regard, one of the most notable modulators of the cardiac rhythm would be the sympathetic and parasympathetic inputs from the ANS. Varying degrees of inputs from these two systems affect the duration between consecutive heartbeats; this variability is known as HRV, and is defined as the time interval between consecutive R wave peaks (i.e., RR intervals) (20).
HRV may be measured across various time-frames—24-hour period, short term (~5 min) or ultra-short term (<5 min). Measurements of HRV can be broadly classified into three domains—time domain, frequency domain, and non-linear measurements. Amongst these, time-domain and frequency-domain analyses are more commonly used due to their ease of analysis (Figure 1).
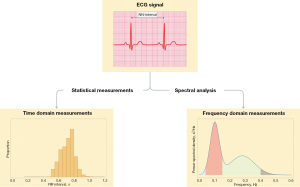
Time domain analysis of HRV utilises the measurements of standard deviation of average normal-normal intervals (SDANN), standard deviation of normal-normal intervals (SDNN), root mean square of successive differences (RMSSD), and percentage of successive normal-normal intervals that differ by more than 50 ms (pNN50) (Table 2) (27,28). These measurements peg differences in heart rate against temporal progression. SDNN is often measured in intervals of 5 minutes, or across a 24-hour interval in long-form measurements (23). It also measures cyclical variation of all factors that contribute to HRV, accounting for both parasympathetic and sympathetic input in cardiac control. In 24-hour recordings, SDNN was shown to be associated with SDANN, total power (TP) and ultra-low frequency (ULF) variability and is associated with variations in magnitude of the heart’s physiological circadian rhythm (29). On the other hand, RMSSD and pNN50 measure HRV between subsequent beats and are linked predominantly to the parasympathetic modulation of heart rate (30,31).
Table 2
Variables | Definition | Significance |
---|---|---|
Time domain analysis | ||
SDNN | Standard deviation of the NN-interval of inter-beat intervals | Direct measure of HRV within measured time period, affected by PNS and SNS modulation (21) |
SDANN | Standard deviation of the averages of NN intervals in all 5-minute segments of the entire recording | Measure of changes in heart rate due to cycles longer than 5 minutes. Correlated with SDNN (22) |
RMSSD | Square root of the mean of the sum of the squares of differences between adjacent NN interval | Linked to parasympathetic vagal modulation of heart rate (22) |
pNN50 | Percentage difference between adjacent NN intervals that are greater than 50 ms | Linked to PNS modulation of heart rate (21) |
Frequency domain analysis | ||
TP | Total power, given by HF + LF + VLF for short-term recordings and HF + LF + VLF + ULF for 24-h recordings | Reflects SNS and PNS activity (23) |
HF power | Power of HF range (0.15–0.4 Hz) | Power in HF, represents vagal modulation |
LF power | 0.04–0.15 Hz | Proxy for input by SNS and PNS |
VLF power | 0.003–0.04 Hz | Reflects slow regulatory mechanisms (e.g., the renin-angiotensin system, thermoregulation) (24) |
ULF power | <0.003 Hz | Reflects circadian rhythm (23,25) |
LF/HF ratio | LF power/HF power | Measure of SNS input against PNS input |
nLF | LF/(LF + HF) | Indicator of aggregate modulation of both the SNS and PNS branches of the ANS (26) |
nHF | HF/(LF + HF) | Indicator of modulation by the PNS (26) |
SDNN, standard deviation of normal-normal intervals; SDANN, standard deviation of average normal-normal intervals; RMSSD, root mean square of successive differences; pNN50, percentage of NN50; HRV, heart rate variability; SNS, sympathetic nervous system; PNS, parasympathetic nervous system; TP, total power; HF, high frequency; LF, low frequency; VLF, very-low frequency; ULF, ultra-low frequency; nLF, normalized low frequency; nHF, normalized high frequency; ANS, autonomic nervous system.
The analysis of HRV in the frequency domain requires conversion of the time domain information into a frequency domain, which is done by performing a Fourier transformation of the ECG signal to obtain spectral density plots tracking changes in amplitude of the ECG signal against frequency. These spectral bands are then interpreted as frequency domains. Frequency domains are banded into ranges and are subsequently classified based on physiological significance. In particular, the frequency bands in the ECG are segregated based on different intervals of frequency into four specific categories, namely the high frequency (HF) band (0.15–0.4 Hz), low frequency (LF) band (0.04–0.15 Hz), very-low frequency (VLF) band (0.003–0.04 Hz) and ULF band (<0.003 Hz).
Conventionally, quantities analysed in the frequency domain include the power of the LF band, which signifies the input due to both components of the ANS, as well as the power of the HF band that represents the parasympathetic input from the vagus nerve (25,32). Analysis of the LF/HF ratio provides a measure of balance between sympathetic and parasympathetic control of the heart, with the average LF/HF ratio in healthy individuals being 1:2 (25). LF and HF can also be normalized to obtain the normalized LF (nLF) and normalized HF (nHF) respectively by dividing the power of the LF and HF frequency bands by the TP of both bands. nLF serves to describe the total effects of the parasympathetic and sympathetic nervous systems, whereas nHF serves as an indicator for parasympathetic-dominant modulation of heart rate (26). Table 1 summarizes the HRV parameters measured in time and frequency domains.
The use of HRV as a diagnostic tool has been appraised in numerous studies and posited for use in the prediction and staging of various pathologies. For example, metabolic syndrome has been associated with a decrease in both LF and HF activity in patients (33). A previous meta-analysis by Williams et al. (34) also noted an association of HRV parameters with an elevation of inflammatory markers, including interleukin (IL)-6, white blood cell count and C-reactive protein levels, suggesting that HRV can be used as a surrogate indicator of inflammation. Such studies demonstrate the potential of the use of HRV as a diagnostic tool, and to predict and stage different pathological conditions.
HRV in AP
Pathophysiology of AP
AP develops from one or more of three main pathophysiological disturbances: duct obstruction, acinar cell injury of the pancreatic parenchyma, or defective intracellular transport of enzymes. These pathophysiological disturbances can occur due to a myriad of root causes such as gallstones, alcohol consumption, hypertriglyceridemia, hypercalcemia, parasites, viruses, medications, and following endoscopic interventions or trauma (35-37). Intrapancreatic activation of pancreatic enzymes such as trypsin leads to an increase in proteolytic and lipolytic activity within the pancreas itself. When combined with ductal obstruction of the pancreas due to gallstones, these activated enzymes are unable to move into the enteric system, resulting in damage to the pancreatic cells (38). This then leads to the release of inflammatory mediators IL-6, IL-8, and tumour necrosis factor-α (TNF-α) (Figure 2), which attract neutrophils and macrophages towards the site of inflammation (39). Activation of neutrophils and macrophages leads to the further release of inflammatory cytokines, resulting in vascular injury, vasodilation and increased vascular permeability. Intraparenchymal fluid accumulation leads to a phenomenon of pancreas compartment syndrome which perpetuates inflammatory cascade by disrupting the parenchymal microcirculation. Vasodilation of the capillaries and capillary leakage of fluid into the interstitium present the sequelae of distributive and hypovolemic shock. Furthermore, the decreased circulatory volume and blood pressure, in a protracted course of the disease, leads to decreased perfusion of various organs, particularly the gut and kidneys, which may result in dysfunction of the intestinal barrier, renal dysfunction and ultimately multiple organ failure (40). The disrupted gut barrier leads to bacterial translocation which is the prime reason for the sterile phenomenon to transit to an infective pathology, typically in the second week and beyond of the disease (41).
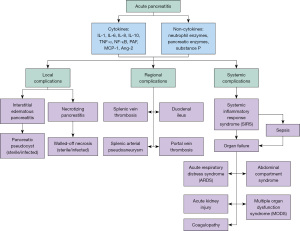
The release of various inflammatory cytokines such as IL-6, IL-8 and TNF-α into the bloodstream in the course of AP inevitably results in aberrations to autonomic function (42). These changes in autonomic function can then be monitored by measuring the HRV parameters, which have also been found to predict morbidity and mortality to a certain extent. Applications of HRV monitoring in the risk of AP and its sequelae will be discussed below, and are summarized in Table 3.
Table 3
Study | HRV parameters |
---|---|
Acute pancreatitis | |
Tryliskyy 2016 (43) | Higher LF/HF ratio predicts post-ERCP pancreatitis |
IPN | |
Zhang 2014 (19) | Lower LF and LF/HF ratio and higher nHF predicts IPN and mortality |
ARDS | |
Chen 2018 (44) | Higher TP and HF levels predicts mortality in ARDS |
SIRS | |
Kovatchev 2003 (45) | High sample asymmetry of RR intervals predicts onset of neonatal SIRS and return to baseline predicts recovery |
Seiver 2003 (46) | ULF-CO is associated with SIRS |
Sepsis | |
Pong 2019 (47) | High RMSSD and HF, and low LF/HF ratio and DFA α2 is associated with mortality in sepsis |
Chen 2018 (44) | Low SDNN, TP, VLF, LF, and LF/HF ratio and high nHF predicts mortality in sepsis |
Wong 2011 (48) | Low TP, LF, VLF and nLF predicts deterioration in sepsis |
Tateishi 2007 (49) | High IL-6 and low LF predicts sepsis, high HF predicts mortality |
MODS | |
Schmidt 2005 (50) | Low SDNN, pNN50, LF, HF, VLF and LF/HF ratio predicts MODS |
Pontet 2003 (51) | Low LF and RMSSD predicts MODS |
HRV, heart rate variability; LF, low frequency; HF, high frequency; ERCP, endoscopic retrograde cholangiopancreatography; IPN, infected pancreatic necrosis; nHF, normalized high frequency; ARDS, acute respiratory distress syndrome; TP, total power; SIRS, systemic inflammatory response syndrome; ULF-CO, ultra-low-frequency periodic oscillations; RMSSD, root mean square of successive differences; DFA α2, detrended fluctuation analysis α2; SDNN, standard deviation of normal-normal intervals; VLF, very-low frequency; nLF, normalized low frequency; IL-6, interleukin-6; pNN50, percentage of NN50; MODS, multiple organ dysfunction syndrome.
Prediction on risk of AP
Common causes of AP include gallstones (range, 40–70%), alcohol (range, 25–35%), hypertriglyceridemia (range, 1–14%) and post-endoscopic retrograde cholangiopancreatogram (ERCP) (range, 3–5%) (52-55). Other rare causes include presence of peri-ampullary tumors, autoimmune etiology, hypercalcemia, medications and genetic mutations. There is little clinical use of risk prediction scoring tools on predicting the risk of pancreatitis based on etiology; however, it is estimated that the risk of biliary pancreatitis is less than 2% over a 30-year period for patients with asymptomatic gallstones (56). Alcoholic pancreatitis usually occurs in patients with more than 5 years of ongoing and substantial alcohol use of about 4–5 unit of alcohol per day, rather than in patients who had binge drinking (57). However, it is important to stratify the risk of iatrogenic causes of AP, e.g., post-ERCP pancreatitis (PEP), as this will help in shared decision-making on whether to proceed with ERCP after weighing the risks and benefits. Tryliskyy et al. (43) found that the LF/HF ratio was significantly higher in patients with AP post-ERCP (median LF/HF: 2.58 vs. 2.10, P<0.001). In addition, the study suggested that it is possible to identify patients at substantial risk of PEP through HRV analysis using a determined LF/HF ratio cutoff of 2.43 with an area under the curve (AUC) of 0.827 and combined sensitivity of 83.3% and specificity of 81.6% (positive predictive value 42%, negative predictive value 97%). Special considerations need to be taken for patients deemed to be at higher risk of PEP, as PEP results in significant morbidity. For instance, patients with asymptomatic hyperbilirubinemia may proceed with magnetic resonance cholangiopancreatogram (MRCP) first, which is non-invasive and does not subject patients to procedural and sedation-related complications of ERCP.
IPN
Acute necrotizing pancreatitis (ANP) occurs in 5–10% of patients with AP, where individuals develop necrosis of the pancreatic parenchyma with or without peripancreatic necrosis. Patients with ANP may subsequently develop single and/or multi-organ systemic failure due to the inflammatory cascade of AP. A retrospective study conducted by Yu et al. (58) noticed that amongst 432 patients with necrotizing pancreatitis, 302 patients (69.9%) developed single organ failure, with a further 85 individuals (19.7%) developing multiple organ failure. In rare cases, the necrotic tissue may be infected, exacerbating the existing necrotic process. Pathogens present in IPN mainly include Enterococcus faecalis (22.5%), Enterococcus faecium (20.0%) and Escherichia coli (20.0%), which tend to thrive within the gastrointestinal and genitourinary systems, suggesting that bacterial translocation from the gut plays a key role in the pathogenesis of infected necrotizing pancreatitis (59). IPN is associated with more than twice the mortality compared to sterile ANP (odds ratio: 2.57, 95% confidence interval: 2.00–3.31) (60).
A risk stratification tool on the risk of IPN in patients with ANP may be useful to determine which high-risk patient groups for closer monitoring and decision for initiation of empirical antibiotics based on clinical judgement and shared decision-making with multi-disciplinary teams. In a study done by Zhang et al. (19), LF and LF/HF levels were decreased and nHF levels were increased in patients with IPN, suggesting that suppression of sympathetic activity was a predominant driving force in the pathophysiology of IPN. In addition, patients who demised from AP were found to have lower nLF and LF/HF levels and higher nHF levels as compared to those who survived. These findings suggest the prospective utility of HRV monitoring in predicting early IPN in patients with moderately severe to severe AP. Nevertheless, the role of prophylactic antibiotics in AP is controversial, with majority of guidelines (61-63) suggesting no role of prophylactic antibiotics in sterile ANP. However, this discussion falls beyond the scope of this review and should be discussed separately.
ARDS
The development of AP is linked to the dysregulation of balance between inflammatory mediators such as IL-6, TNF-α, IL-1β, IL-8 and NF-κB, nuclear factor kappa-light-chain-enhancer of activated B cells (NF-κB), and anti-inflammatory mediators including IL-10 and IL-1 receptor antagonists (64). This imbalance leads to systemic inflammation and results in the subsequent development of systemic complications. Amongst the numerous systemic complications of AP, respiratory complications such as acute lung injury (ALI) and ARDS are associated with the highest mortality within patients with single organ failure (65). The development of respiratory complications such as ALI and ARDS are posited to be linked to the presence of inflammatory mediators such as IL-6, TNF-α, IL-1β, IL-8, NF-κB, phospholipase A2 and platelet activating factor, and reduced levels of anti-inflammatory cytokine IL-10 (66-68). Such inflammatory mediators can travel via the bloodstream and lymphatics to the pulmonary circulation, where they cause damage to endothelial cells and increase endothelial permeability. In addition, Montravers et al. (69) found elevated levels of neutrophil enzymes myeloperoxidase and lactoferrin, and pancreatic enzymes amylase, lipase, and trypsin in plasma and lymph in patients with ALI complicating severe pancreatitis, suggesting the contribution of neutrophil and pancreatic enzymes towards the development of ALI and ARDS.
The physiological stress and respiratory failure associated with ARDS, due to the widespread inflammation in the lungs, can impact the ANS, and consequently, HRV. To attenuate cytokine-induced systemic inflammation in ARDS, the ANS reacts by increasing vagal modulation, which is reflected by changes in HRV parameters. Chen et al. (44) investigated the HRV parameters that are associated with outcomes of ARDS patients and found that the mean RR interval (mRRI), HF and the product of LF/HF and tidal volume were significantly lower, while the nHF to tidal volume ratio was significantly higher in ARDS patients. Moreover, TP and HF were also found to be significantly higher in non-survivor ARDS patients and are independent predictors of mortality in ARDS patients. These results suggest that ARDS patients have a higher vagal modulation and lower sympathetic modulation than non-ARDS patients, and that non-survivor ARDS patients had even higher vagal modulation than the survivors. Thus, HF and TP might be useful parameters in prognosticating outcomes in patients with ARDS.
SIRS and MODS
Another systemic complication of AP is SIRS, which has also been shown to be a predictor of severity of AP, and has been linked to greater severity of AP at initial presentation (70). While SIRS was initially used to identify patients with sepsis, this definition has now been abandoned in the Sepsis-3 consensus statements (71). Nevertheless, the pathophysiology behind SIRS should be delved into to understand the systemic inflammation and downstream complications associated with SIRS. The development of SIRS stems from the release of cytokines and pancreatic enzymes into the general circulation. These chemicals may affect peripheral organs, presenting as respiratory distress, renal failure, cardiac dysfunction and shock. Multiple studies report an early proinflammatory response in severe AP, characterized by elevated levels of pro-inflammatory cytokines including IL-6, IL-8, monocyte chemoattractant protein-1 (MCP-1), IL-1β, substance P and TNF-α, inter alia (72,73). The efflux of these mediators results in subsequent neutrophil and leukocyte activation, and further release of proinflammatory cytokines, thereby perpetuating systemic inflammation and resulting in SIRS (74). The same inflammatory cytokines also interfere with autonomic neuronal transmission to cardiomyocyte pacemaker and ventricular cells, resulting in myocardial depression, increased heart rate and strongly depressed HRV, all suggestive of a poorer prognosis (75).
In a case series of critically ill adults, it was found that ultra-low-frequency periodic oscillations in cardiac output (ULF-CO) were associated with SIRS and could serve as a potential pathophysiologic marker in its prognosis, though more research is required in this area (46). Another study by Kovatchev et al. (45) used sample asymmetry analysis to assess HRV in infants and found that the sample asymmetry of RR intervals increased in 3 to 4 days preceding SIRS, and returned to baseline after treatment and recovery, supporting the use of HRV monitoring in detecting the early stages of neonatal SIRS. Although HRV yields potential in predicting the onset and recovery of patients with SIRS, more research is required to demonstrate its effectiveness in patients who develop SIRS as a complication of AP in particular.
As systemic inflammation progresses, proinflammatory cytokines can mediate excessive leukocyte activation, causing leukocytes to be lodged in the microcirculation of organs and migrate into the interstitium, damaging these organs (usually lungs, kidneys and liver). The development of organ failure is also closely linked to heightened levels of proinflammatory cytokines. A study by Greer et al. (72) identified elevated levels of angiopoietin-2, IL-8, and MCP-1 in persistent organ failure as compared to transient organ failure and pancreatic necrosis. In addition, the translocation of toxins and bacteria from sepsis further aggravates the systemic inflammatory response and contributes further to the development of MODS (74).
In MODS, cardiac regulation is impaired, manifesting as reduced cardiac contractility, chronotropic incompetence that yields a reduced HRV and an increased heart rate (76). Schmidt and colleagues (50) found that HRV parameters SDNN, pNN50, LF, HF, VLF and LF/HF were significantly lower in patients with MODS, indicating blunted interorgan communication that is in keeping with the complementary hypothesis of an “uncoupling of biological oscillators” as described by Godin in 1996 (77). The study also found a decrease in baroreflex sensitivity in MODS patients, which, together with the HRV parameters, suggest a decrease in parasympathetic vagal activity. Since vagus stimulation attenuates the systemic inflammatory response to endotoxin, and inflammatory stimuli are able to activate sensory pathways that elicit a prompt cholinergic anti-inflammatory reaction to prevent the spillover of inflammatory products to the circulation, the decrease in vagal activity suggests a morbid turn of events that leads to a circulatory influx of proinflammatory products, which thereby results in MODS. Hence, HRV parameters which indicate decreased vagal stimulation can predict the onset of MODS (78-80). Another study by Pontet et al. (51) found that the baseline HRV measures LF and RMSSD on admission of patients who subsequently developed MODS were significantly lower as compared to those who did not. The decrease in sympathetic activity as indicated by the lower LF results in inotropic dysfunction of the heart, leading to the development of MODS (75). Therefore, HRV measures can be used to predict the onset and prognosticate patient outcomes in MODS, which is uncommon but entails serious complications in AP.
Sepsis
Apart from SIRS, sepsis is another potential complication with an incidence of up to 22% of patients with severe AP (81). As mentioned previously, patients with severe AP are at risk of developing IPN, and pathogens colonizing the necrotic foci may seed into the bloodstream, leading to bacteraemia. Coupled with the spillover of proinflammatory mediators, this may precipitate sepsis, an interminable systemic inflammatory response and eventually MODS, which has a higher rate of mortality (82). Another source of bacteria for sepsis has also been postulated to be due to increased intestinal permeability. Decreased perfusion to the gut during AP has been suggested as a cause of intestinal barrier dysfunction (83,84), which results in intestinal ischemia, damaging the mucosal epithelium and endothelium in the gut. Furthermore, during AP, tight junction proteins such as occludin, claudins and ZO-1 are downregulated, increasing the risk of endotoxin circulation and bacterial translocation (85). Such factors contribute to an increase in intestinal permeability, which increases the risk of translocation of intestinal bacteria to the pancreas (86). A previous study by Agarwal et al. (85) observed a close relation between AP and intestinal barrier dysfunction, primarily manifesting as increased intestinal permeability, serum endotoxemia and bacteraemia. Similarly, a study by Schietroma et al. (87) evaluated the intestinal permeability of 63 patients over a span of 11 days, and noted an impaired gut barrier function, which led to systemic endotoxemia. Whether from the necrotic foci of IPN or due to bacterial translocation from bowels, the sepsis that results from it leads to alterations in ANS activity, which can be picked up by HRV monitoring.
A study by Tateishi et al. (49) found that patients in septic shock present with high IL-6 levels and low LF, indicating systemic inflammation, as well as decreased sympathovagal activity. In addition, HF was found to be higher in non-survivors, indicating that an increase in vagus nerve activity prognosticates increased mortality (88). In another study investigating HRV in septic patients in Hong Kong, it was similarly found that TP, LF, VLF and nLF are significantly reduced in patients with sepsis whose condition deteriorated (48). The marked suppression of HRV is oftentimes observed before the onset of septic shock, suggesting that a collapse of homeostasis as mediated by the ANS is closely related to the onset of septic shock. The strong correlation between IL-6 levels and HRV indices also suggest that HRV indices can act as a surrogate marker for IL-6 markers in determining the severity of systemic inflammation in sepsis.
Prognostication of AP using HRV parameters
As described earlier, several scoring systems have been developed to stratify the severity of AP based on clinical and laboratory parameters but are cumbersome and are dynamic (will need repeat scoring as risk may change) (89). On the other hand, monitoring HRV parameters can be performed continuously to check disease progression, is non-invasive, and can be more efficient for measurement and interpretation. Zhang et al. (19) demonstrated that nHF and LF/HF could predict IPN better than the APACHE II scoring system (AUC for nHF: 0.927, AUC for LF/HF: 0.821, AUC for APACHE II: 0.785), and that LF/HF could also predict MODS better than APACHE II (AUC for LF/HF: 0.906, AUC for APACHE II: 0.899). This demonstrates the potential use of HRV parameters as a non-invasive method to predict and prognosticate the complications of AP.
Besides that, systemic complications of AP such as sepsis can also be identified by scoring systems such as the quick SOFA (qSOFA) score (sepsis-related) (90), National Early Warning Score (NEWS) (91) and Modified Early Warning Score (MEWS) (92). To compare the effectiveness of using HRV parameters to predict outcomes in septic patients, Samsudin et al. (93) came up with the Singapore Emergency Department Sepsis (SEDS) predictive model that utilized HRV parameters mRRI and detrended fluctuation analysis α2 (DFA α2) to predict 30-day in-hospital mortality in patients with sepsis. The study found that the SEDS model significantly outperformed the qSOFA, NEWS and MEWS score in predicting the primary and composite outcomes of 30-day in-hospital mortality in septic patients in the Singapore emergency department. Chiew et al. (94) further described the use of machine learning models to boost the said SEDS model to better predict 30-day in-hospital mortality in septic patients, which performed better than the qSOFA, NEWS and MEWS scores. In addition, Pong et al. (47) created a rapid triage model comprising of four clinical variables and the HRV parameter DFA α2 that can be determined quickly at the bedside and this was found to be superior at predicting mortality than qSOFA, NEWS, MEWS, SOFA and APACHE II, all while requiring less time, information and procedures to derive. Additionally, HRV parameters can also be applied to predict outcomes in ARDS. Chen et al. (44) found that HF and TP were more sensitive than APACHE II and the Acute Lung Injury Score (95) in predicting mortality. Coupled with the fact that continuous HRV monitoring is feasible in ambulatory patients and is non-invasive, it shows great potential to prognosticate the outcomes and severity of AP.
Despite its advantages, HRV monitoring is not without its limitations and challenges. Firstly, age has been found to be a confounding factor in HRV as physiological decreases in HRV are observed in older patients (48,96). In addition, the presence of cardiac arrhythmias such as atrial fibrillation, presence of ectopic beats and heart blocks precludes the measurement of HRV parameters in this important subset of patients who are at greater risk of mortality from AP in the first place. Last but not least, many studies have hitherto investigated the relationship of HRV parameters with systemic complications of pancreatitis such as SIRS, sepsis, MODS and ARDS, but the aetiologies of those syndromes may not have been from AP. Hence, more research is needed to further solidify the association of HRV measurements with outcomes of AP specifically and its effectiveness in prognosticating severity in the future.
Future directions
While the examples and case studies presented highlight the potential of HRV as a prognostic marker in AP, several challenges and avenues for future research should be acknowledged. The lack of standardized HRV metrics and normative values poses a challenge to the widespread adoption of HRV in AP. Establishing uniform guidelines for HRV assessment and interpretation is crucial for ensuring consistency and comparability across studies. The integration of HRV measurements into routine clinical practice also requires overcoming logistical and technological barriers. Clinicians need user-friendly tools and platforms for seamless HRV monitoring, and healthcare systems must adapt to accommodate this additional layer of information in patient care. It is also important to note that HRV measurements cannot be used exclusively in the diagnosis of AP, but can serve as a reinforcement for the prognosis of the disease. Despite these challenges, HRV monitoring still remains a promising tool for prognostication and monitoring of AP.
Conclusions
In conclusion, preliminary studies have shown that certain parameters of HRV may be used to predict the severity of AP and prognosticate outcomes. Although HRV monitoring demonstrates potential to be superior to existing scoring systems in AP, more research is needed to validate its use as a prognostic tool.
Acknowledgments
Funding: None.
Footnote
Reporting Checklist: The authors have completed the Narrative Review reporting checklist. Available at https://tgh.amegroups.com/article/view/10.21037/tgh-24-22/rc
Peer Review File: Available at https://tgh.amegroups.com/article/view/10.21037/tgh-24-22/prf
Conflicts of Interest: All authors have completed the ICMJE uniform disclosure form (available at https://tgh.amegroups.com/article/view/10.21037/tgh-24-22/coif). V.G.S. serves as an unpaid editorial board member of Translational Gastroenterology and Hepatology from August 2024 to July 2026. The other authors have no conflicts of interest to declare.
Ethical Statement: The authors are accountable for all aspects of the work in ensuring that questions related to the accuracy or integrity of any part of the work are appropriately investigated and resolved.
Open Access Statement: This is an Open Access article distributed in accordance with the Creative Commons Attribution-NonCommercial-NoDerivs 4.0 International License (CC BY-NC-ND 4.0), which permits the non-commercial replication and distribution of the article with the strict proviso that no changes or edits are made and the original work is properly cited (including links to both the formal publication through the relevant DOI and the license). See: https://creativecommons.org/licenses/by-nc-nd/4.0/.
References
- Li CL, Jiang M, Pan CQ, et al. The global, regional, and national burden of acute pancreatitis in 204 countries and territories, 1990-2019. BMC Gastroenterol 2021;21:332. [Crossref] [PubMed]
- Peery AF, Crockett SD, Barritt AS, et al. Burden of Gastrointestinal, Liver, and Pancreatic Diseases in the United States. Gastroenterology 2015;149:1731-1741.e3. [Crossref] [PubMed]
- Chan EE, Shelat VG. Pancreaticopleural Fistula Causing Massive Right Hydrothorax and Respiratory Failure. Case Rep Surg 2016;2016:8294056. [Crossref] [PubMed]
- Schepers NJ, Bakker OJ, Besselink MG, et al. Impact of characteristics of organ failure and infected necrosis on mortality in necrotising pancreatitis. Gut 2019;68:1044-51. [Crossref] [PubMed]
- van Santvoort HC, Bakker OJ, Bollen TL, et al. A conservative and minimally invasive approach to necrotizing pancreatitis improves outcome. Gastroenterology 2011;141:1254-63. [Crossref] [PubMed]
- Bang JY, Wilcox CM, Arnoletti JP, et al. Superiority of endoscopic interventions over minimally invasive surgery for infected necrotizing pancreatitis: meta-analysis of randomized trials. Dig Endosc 2020;32:298-308. [Crossref] [PubMed]
- Kiat TTJ, Gunasekaran SK, Junnarkar SP, et al. Are traditional scoring systems for severity stratification of acute pancreatitis sufficient? Ann Hepatobiliary Pancreat Surg 2018;22:105-15. [Crossref] [PubMed]
- Banks PA, Bollen TL, Dervenis C, et al. Classification of acute pancreatitis--2012: revision of the Atlanta classification and definitions by international consensus. Gut 2013;62:102-11. [Crossref] [PubMed]
- Knaus WA, Draper EA, Wagner DP, et al. APACHE II: a severity of disease classification system. Crit Care Med 1985;13:818-29. [Crossref] [PubMed]
- Gao W, Yang HX, Ma CE. The Value of BISAP Score for Predicting Mortality and Severity in Acute Pancreatitis: A Systematic Review and Meta-Analysis. PLoS One 2015;10:e0130412. [Crossref] [PubMed]
- Lambden S, Laterre PF, Levy MM, et al. The SOFA score-development, utility and challenges of accurate assessment in clinical trials. Crit Care 2019;23:374. [Crossref] [PubMed]
- Teng TZJ, Tan JKT, Baey S, et al. Sequential organ failure assessment score is superior to other prognostic indices in acute pancreatitis. World J Crit Care Med 2021;10:355-68. [Crossref] [PubMed]
- Ranson JH, Rifkind KM, Roses DF, et al. Prognostic signs and the role of operative management in acute pancreatitis. Surg Gynecol Obstet 1974;139:69-81. [PubMed]
- van Ravenswaaij-Arts CM, Kollée LA, Hopman JC, et al. Heart rate variability. Ann Intern Med 1993;118:436-47. [Crossref] [PubMed]
- Hinde K, White G, Armstrong N. Wearable Devices Suitable for Monitoring Twenty Four Hour Heart Rate Variability in Military Populations. Sensors (Basel) 2021;21:1061. [Crossref] [PubMed]
- Hon EH, Lee ST. Electronic evaluation of the fetal heart rate. VIII. Patterns preceding fetal death, further observations. Am J Obstet Gynecol 1963;87:814-26. [PubMed]
- Bishop DG, Wise RD, Lee C, et al. Heart rate variability predicts 30-day all-cause mortality in intensive care units. Southern African Journal of Anaesthesia and Analgesia 2016;22:125-8. [Crossref]
- Siepmann M, Weidner K, Petrowski K, et al. Heart Rate Variability: A Measure of Cardiovascular Health and Possible Therapeutic Target in Dysautonomic Mental and Neurological Disorders. Appl Psychophysiol Biofeedback 2022;47:273-87. [Crossref] [PubMed]
- Zhang L, Zhou J, Ke L, et al. Role of heart rate variability in predicting the severity of severe acute pancreatitis. Dig Dis Sci 2014;59:2557-64. [Crossref] [PubMed]
- De Couck M, Gidron Y. Norms of vagal nerve activity, indexed by Heart Rate Variability, in cancer patients. Cancer Epidemiol 2013;37:737-41. [Crossref] [PubMed]
- Umetani K, Singer DH, McCraty R, et al. Twenty-four hour time domain heart rate variability and heart rate: relations to age and gender over nine decades. J Am Coll Cardiol 1998;31:593-601. [Crossref] [PubMed]
- Shaffer F, McCraty R, Zerr CL. A healthy heart is not a metronome: an integrative review of the heart's anatomy and heart rate variability. Front Psychol 2014;5:1040. [Crossref] [PubMed]
- Shaffer F, Ginsberg JP. An Overview of Heart Rate Variability Metrics and Norms. Front Public Health 2017;5:258. [Crossref] [PubMed]
- Rajendra Acharya U, Paul Joseph K, Kannathal N, et al. Heart rate variability: a review. Med Biol Eng Comput 2006;44:1031-51. [Crossref] [PubMed]
- Tiwari R, Kumar R, Malik S, et al. Analysis of Heart Rate Variability and Implication of Different Factors on Heart Rate Variability. Curr Cardiol Rev 2021;17:e160721189770. [Crossref] [PubMed]
- Burr RL. Interpretation of normalized spectral heart rate variability indices in sleep research: a critical review. Sleep 2007;30:913-9. [Crossref] [PubMed]
- Johnston BW, Barrett-Jolley R, Krige A, et al. Heart rate variability: Measurement and emerging use in critical care medicine. J Intensive Care Soc 2020;21:148-57. [Crossref] [PubMed]
- Burr RL, Motzer SA, Chen W, et al. Heart rate variability and 24-hour minimum heart rate. Biol Res Nurs 2006;7:256-67. [Crossref] [PubMed]
- Bigger JT Jr, Fleiss JL, Steinman RC, et al. RR variability in healthy, middle-aged persons compared with patients with chronic coronary heart disease or recent acute myocardial infarction. Circulation 1995;91:1936-43. [Crossref] [PubMed]
- Constantinescu V, Arsenescu-Georgescu C, Matei D, et al. Heart rate variability analysis and cardiac dysautonomia in ischemic stroke patients. Clin Neurol Neurosurg 2019;186:105528. [Crossref] [PubMed]
- Sinha M, Behera AK, Sinha R, et al. Circadian rhythmicity of heart rate variability and its impact on cardiac autonomic modulation in asthma. Chronobiol Int 2021;38:1631-9. [Crossref] [PubMed]
- Spiers JP, Silke B, McDermott U, et al. Time and frequency domain assessment of heart rate variability: a theoretical and clinical appreciation. Clin Auton Res 1993;3:145-58. [Crossref] [PubMed]
- Ortiz-Guzmán JE, Mollà-Casanova S, Serra-Añó P, et al. Short-Term Heart Rate Variability in Metabolic Syndrome: A Systematic Review and Meta-Analysis. J Clin Med 2023;12:6051. [Crossref] [PubMed]
- Williams DP, Koenig J, Carnevali L, et al. Heart rate variability and inflammation: A meta-analysis of human studies. Brain Behav Immun 2019;80:219-26. [Crossref] [PubMed]
- Teng TZJ, Chua BQY, Lim PK, et al. Occam's razor or Hickam's dictum-COVID-19 is not a textbook aetiology of acute pancreatitis: A modified Naranjo Score appraisal. World J Gastroenterol 2023;29:2050-63. [Crossref] [PubMed]
- Shelat VG. Sulfasalazine induced acute pancreatitis in a patient with prior cholecystectomy. Postgrad Med J 2021;97:738-9. [Crossref] [PubMed]
- Lee KH, Shelat VG, Low HC, et al. Recurrent pancreatitis secondary to pancreatic ascariasis. Singapore Med J 2009;50:e218-9. [PubMed]
- Zilio MB, Eyff TF, Azeredo-Da-Silva ALF, et al. A systematic review and meta-analysis of the aetiology of acute pancreatitis. HPB (Oxford) 2019;21:259-67. [Crossref] [PubMed]
- Jakkampudi A, Jangala R, Reddy R, et al. Acinar injury and early cytokine response in human acute biliary pancreatitis. Sci Rep 2017;7:15276. [Crossref] [PubMed]
- Al Mofleh IA. Severe acute pancreatitis: pathogenetic aspects and prognostic factors. World J Gastroenterol 2008;14:675-84. [Crossref] [PubMed]
- Chan KS, Shelat VG. Diagnosis, severity stratification and management of adult acute pancreatitis-current evidence and controversies. World J Gastrointest Surg 2022;14:1179-97. [Crossref] [PubMed]
- Pooran N, Indaram A, Singh P, et al. Cytokines (IL-6, IL-8, TNF): early and reliable predictors of severe acute pancreatitis. J Clin Gastroenterol 2003;37:263-6. [Crossref] [PubMed]
- Tryliskyy Y, Bryce GJ. Role of Heart Rate Variability in Predicting Post-Endoscopic Retrograde Cholangiopancreatography Pancreatitis. Acta Gastroenterol Belg 2016;79:429-34. [PubMed]
- Chen IC, Kor CT, Lin CH, et al. High-frequency power of heart rate variability can predict the outcome of thoracic surgical patients with acute respiratory distress syndrome on admission to the intensive care unit: a prospective, single-centric, case-controlled study. BMC Anesthesiol 2018;18:34. [Crossref] [PubMed]
- Kovatchev BP, Farhy LS, Cao H, et al. Sample asymmetry analysis of heart rate characteristics with application to neonatal sepsis and systemic inflammatory response syndrome. Pediatr Res 2003;54:892-8. [Crossref] [PubMed]
- Seiver AJ, Szaflarski NL. Report of a case series of ultra low-frequency oscillations in cardiac output in critically ill adults with sepsis, systemic inflammatory response syndrome, and multiple organ dysfunction syndrome. Shock 2003;20:101-9. [Crossref] [PubMed]
- Pong JZ, Fook-Chong S, Koh ZX, et al. Combining Heart Rate Variability with Disease Severity Score Variables for Mortality Risk Stratification in Septic Patients Presenting at the Emergency Department. Int J Environ Res Public Health 2019;16:1725. [Crossref] [PubMed]
- Wong W, Lit A. Prospective Observational Study on Heart Rate Variability in Emergency Department Patients with Sepsis. Hong Kong Journal of Emergency Medicine 2011;18:307-15. [Crossref]
- Tateishi Y, Oda S, Nakamura M, et al. Depressed heart rate variability is associated with high IL-6 blood level and decline in the blood pressure in septic patients. Shock 2007;28:549-53. [Crossref] [PubMed]
- Schmidt H, Müller-Werdan U, Hoffmann T, et al. Autonomic dysfunction predicts mortality in patients with multiple organ dysfunction syndrome of different age groups. Crit Care Med 2005;33:1994-2002. [Crossref] [PubMed]
- Pontet J, Contreras P, Curbelo A, et al. Heart rate variability as early marker of multiple organ dysfunction syndrome in septic patients. J Crit Care 2003;18:156-63. [Crossref] [PubMed]
- Walkowska J, Zielinska N, Karauda P, et al. The Pancreas and Known Factors of Acute Pancreatitis. J Clin Med 2022;11:5565. [Crossref] [PubMed]
- Chatila AT, Bilal M, Guturu P. Evaluation and management of acute pancreatitis. World J Clin Cases 2019;7:1006-20. [Crossref] [PubMed]
- Mandalia A, Wamsteker EJ, DiMagno MJ. Recent advances in understanding and managing acute pancreatitis. F1000Res 2018;7:F1000 Faculty Rev-959.
- Gimenez TR, Calvo AG, Vicent JG. Etiology of acute pancreatitis. Cent Eur J Med 2014;9:530-42.
- Lowenfels AB, Lankisch PG, Maisonneuve P. What is the risk of biliary pancreatitis in patients with gallstones? Gastroenterology 2000;119:879-80. [Crossref] [PubMed]
- DiMagno MJ. Oktoberfest binge drinking and acute pancreatitis: is there really no relationship? Clin Gastroenterol Hepatol 2011;9:920-2. [Crossref] [PubMed]
- Yu L, Xie F, Luo L, et al. Clinical characteristics and risk factors of organ failure and death in necrotizing pancreatitis. BMC Gastroenterol 2023;23:19. [Crossref] [PubMed]
- Mowbray NG, Ben-Ismaeil B, Hammoda M, et al. The microbiology of infected pancreatic necrosis. Hepatobiliary Pancreat Dis Int 2018;17:456-60. [Crossref] [PubMed]
- Werge M, Novovic S, Schmidt PN, et al. Infection increases mortality in necrotizing pancreatitis: A systematic review and meta-analysis. Pancreatology 2016;16:698-707. [Crossref] [PubMed]
- Lee SH, Choe JW, Cheon YK, et al. Revised Clinical Practice Guidelines of the Korean Pancreatobiliary Association for Acute Pancreatitis. Gut Liver 2023;17:34-48. [Crossref] [PubMed]
- Leppäniemi A, Tolonen M, Tarasconi A, et al. 2019 WSES guidelines for the management of severe acute pancreatitis. World J Emerg Surg 2019;14:27. [Crossref] [PubMed]
- IAP/APA evidence-based guidelines for the management of acute pancreatitis. Pancreatology 2013;13:e1-15. [Crossref] [PubMed]
- Granger J, Remick D. Acute pancreatitis: models, markers, and mediators. Shock 2005;24:45-51. [Crossref] [PubMed]
- Kong L, Santiago N, Han TQ, et al. Clinical characteristics and prognostic factors of severe acute pancreatitis. World J Gastroenterol 2004;10:3336-8. [Crossref] [PubMed]
- Zhou J, Zhou P, Zhang Y, et al. Signal Pathways and Markers Involved in Acute Lung Injury Induced by Acute Pancreatitis. Dis Markers 2021;2021:9947047. [Crossref] [PubMed]
- Samanta J, Singh S, Arora S, et al. Cytokine profile in prediction of acute lung injury in patients with acute pancreatitis. Pancreatology 2018;18:878-84. [Crossref] [PubMed]
- De Campos T, Deree J, Coimbra R. From acute pancreatitis to end-organ injury: mechanisms of acute lung injury. Surg Infect (Larchmt) 2007;8:107-20. [Crossref] [PubMed]
- Montravers P, Chollet-Martin S, Marmuse JP, et al. Lymphatic release of cytokines during acute lung injury complicating severe pancreatitis. Am J Respir Crit Care Med 1995;152:1527-33. [Crossref] [PubMed]
- Singh VK, Wu BU, Bollen TL, et al. Early systemic inflammatory response syndrome is associated with severe acute pancreatitis. Clin Gastroenterol Hepatol 2009;7:1247-51. [Crossref] [PubMed]
- Singer M, Deutschman CS, Seymour CW, et al. The Third International Consensus Definitions for Sepsis and Septic Shock (Sepsis-3). JAMA 2016;315:801-10. [Crossref] [PubMed]
- Greer PJ, Lee PJ, Paragomi P, et al. Severe acute pancreatitis exhibits distinct cytokine signatures and trajectories in humans: a prospective observational study. Am J Physiol Gastrointest Liver Physiol 2022;323:G428-38. [Crossref] [PubMed]
- Malmstrøm ML, Hansen MB, Andersen AM, et al. Cytokines and organ failure in acute pancreatitis: inflammatory response in acute pancreatitis. Pancreas 2012;41:271-7. [Crossref] [PubMed]
- Bhatia M, Brady M, Shokuhi S, et al. Inflammatory mediators in acute pancreatitis. J Pathol 2000;190:117-25. [Crossref] [PubMed]
- Werdan K, Schmidt H, Ebelt H, et al. Impaired regulation of cardiac function in sepsis, SIRS, and MODS. Can J Physiol Pharmacol 2009;87:266-74. [Crossref] [PubMed]
- Heinroth KM, Kuhn C, Stache N, et al. Eingeschränkte Herzfrequenzvariabilität bei Patienten mit septischem und nicht-septischem Multiorgan-Dysfunktions-Syndrom. Intensivmedizin und Notfallmedizin 1999;36:436-45. [Crossref]
- Godin PJ, Buchman TG. Uncoupling of biological oscillators: a complementary hypothesis concerning the pathogenesis of multiple organ dysfunction syndrome. Crit Care Med 1996;24:1107-16. [Crossref] [PubMed]
- Tracey KJ. The inflammatory reflex. Nature 2002;420:853-9. [Crossref] [PubMed]
- Borovikova LV, Ivanova S, Zhang M, et al. Vagus nerve stimulation attenuates the systemic inflammatory response to endotoxin. Nature 2000;405:458-62. [Crossref] [PubMed]
- Libert C. Inflammation: A nervous connection. Nature 2003;421:328-9. [Crossref] [PubMed]
- Mifkovic A, Pindak D, Daniel I, et al. Septic complications of acute pancreatitis. Bratisl Lek Listy 2006;107:296-313. [PubMed]
- Wilson PG, Manji M, Neoptolemos JP. Acute pancreatitis as a model of sepsis. J Antimicrob Chemother 1998;41 Suppl A:51-63.
- Rahman SH, Ammori BJ, Holmfield J, et al. Intestinal hypoperfusion contributes to gut barrier failure in severe acute pancreatitis. J Gastrointest Surg 2003;7:26-36. [Crossref] [PubMed]
- Ammori BJ, Leeder PC, King RF, et al. Early increase in intestinal permeability in patients with severe acute pancreatitis: correlation with endotoxemia, organ failure, and mortality. J Gastrointest Surg 1999;3:252-62. [Crossref] [PubMed]
- Agarwal S, Goswami P, Poudel S, et al. Acute pancreatitis is characterized by generalized intestinal barrier dysfunction in early stage. Pancreatology 2023;23:9-17. [Crossref] [PubMed]
- Isaji S, Mizuno S, Tabata M, et al. Bacterial analysis of infected pancreatic necrosis and its prevention (Symposium 8: Pancreatobiliary infection (IHPBA)). J Hepatobiliary Pancreat Surg 2003;10:419-24. [Crossref] [PubMed]
- Schietroma M, Pessia B, Carlei F, et al. Intestinal permeability and systemic endotoxemia in patients with acute pancreatitis. Ann Ital Chir 2016;87:138-44. [PubMed]
- Heart rate variability: standards of measurement, physiological interpretation and clinical use. Task Force of the European Society of Cardiology and the North American Society of Pacing and Electrophysiology. Circulation 1996;93:1043-65. [Crossref] [PubMed]
- Mounzer R, Langmead CJ, Wu BU, et al. Comparison of existing clinical scoring systems to predict persistent organ failure in patients with acute pancreatitis. Gastroenterology 2012;142:1476-82; quiz e15-6. [Crossref] [PubMed]
- Govindan S, Prescott HC. Quick Sequential Organ Failure Assessment: Illness Severity Indicator, Clinical Decision Support Tool, or Both? Crit Care Med 2017;45:1947-9. [Crossref] [PubMed]
- Durr D, Niemi T, Despraz J, et al. National Early Warning Score (NEWS) Outperforms Quick Sepsis-Related Organ Failure (qSOFA) Score for Early Detection of Sepsis in the Emergency Department. Antibiotics (Basel) 2022;11:1518. [Crossref] [PubMed]
- Subbe CP, Kruger M, Rutherford P, et al. Validation of a modified Early Warning Score in medical admissions. QJM 2001;94:521-6. [Crossref] [PubMed]
- Samsudin MI, Liu N, Prabhakar SM, et al. A novel heart rate variability based risk prediction model for septic patients presenting to the emergency department. Medicine (Baltimore) 2018;97:e10866. [Crossref] [PubMed]
- Chiew CJ, Liu N, Tagami T, et al. Heart rate variability based machine learning models for risk prediction of suspected sepsis patients in the emergency department. Medicine (Baltimore) 2019;98:e14197. [Crossref] [PubMed]
- Murray JF, Matthay MA, Luce JM, et al. An expanded definition of the adult respiratory distress syndrome. Am Rev Respir Dis 1988;138:720-3. [Crossref] [PubMed]
- Korach M, Sharshar T, Jarrin I, et al. Cardiac variability in critically ill adults: influence of sepsis. Crit Care Med 2001;29:1380-5. [Crossref] [PubMed]
Cite this article as: Liau MYQ, Liau JYJ, Selvakumar SV, Chan KS, Shelat VG. Heart rate variability in acute pancreatitis: a narrative review. Transl Gastroenterol Hepatol 2024;9:68.