Clinical significance of peripheral T-cell receptor repertoire profiling and individualized nomograms in patients with gastrointestinal cancer treated with anti-programmed death 1 antibody
Highlight box
Key findings
• High diversity evenness 50 (DE50) scores were associated with better responses to anti-programmed death 1 (PD-1) treatment and longer progression-free survival (PFS) in gastrointestinal cancer (GIC) patients.
• A nomogram based on T-cell receptor (TCR) repertoire profiling was developed to aid in risk assessment and guide clinical decision-making for anti-PD-1 antibody treatment.
What is known and what is new?
• Immune checkpoint inhibitors have shown clinical benefits in a subset of GIC patients, but predicting responses has been challenging.
• Peripheral TCR repertoire profiling could be a potential predictor for clinical efficacy. A new, highly predictive nomogram based on TCR repertoire profiling was introduced, showing promise in predicting treatment outcomes.
What is the implication, and what should change now?
• TCR repertoire profiling, especially the DE50 scores, can be an essential tool in predicting patient responses to anti-PD-1 treatment and PFS.
• Given the association between high DE50 scores and good survival, clinicians could monitor DE50 scores to direct future immunotherapy.
Introduction
Gastrointestinal cancers (GICs) including esophageal, gastric, and colorectal cancers are common malignancies which are often diagnosed at a late stage (1). The lack of effective early diagnosis and treatment strategies of GIC result in a poor prognosis. Currently, immunotherapy such as anti-programmed death 1 (PD-1) antibody has gained a significant value in the treatment of some GICs and has been incorporated into treatment regimens (2). However, a large number of patients do not respond to these treatment approaches, and toxicity may be substantial. Thus, it is of great significance to identify a biomarker to predict patients who will respond to anti-PD-1 therapy and then help optimizing patients’ stratification for the best possible therapeutic outcome.
T-cell receptor (TCR) is a surface molecule of T cells that could specifically recognize antigens presented by the major histocompatibility complex (MHC) and mediate immune response (3). It is a heterodimer, the majority (∼95%) of which consists of an α-chain and a β-chain in human (4). The complimentary determining region 3 (CDR3) of TCRβ chain is the highly variable component of TCRs and is critical for the specificity of each T cell clone for antigen recognition (5). CDR3 polymorphisms generated by random rearrangements of the variable (V), diversity (D), and joining (J) gene segments, determines TCR diversity. Random insertion or deletion of non-template nucleotides in V-D and D-J junction regions during rearrangement increase TCR diversity. Theoretically, the ability of the immune responses to a variety of different antigens depends on a large repertoire of unique TCR.
Several studies have shown that TCR repertoire diversity plays an important role in tumor immunity (6-9). Analysis of TCR repertoire profiling can be used to reflect the immune responses for some patients and may help predict the clinical outcomes of anti-PD-1 therapy. In this study, we used the Oncomine TCR Beta-LR Assay to detect the possible V-J rearrangement in the CDR3 region of the TCRβ chain in peripheral blood samples from GIC patients. We showed that patients with a high diversity evenness 50 (DE50) score could benefit from anti-PD-1 treatment. These findings would help to characterize the TCR repertoire profiling in the tumor microenvironment in GICs. Furthermore, we suggested that the quantification of TCR repertoire diversity in the peripheral blood, prior to treatment initiation, could represent a predictive biomarker to guide the use of immunotherapies to the most appropriate GIC patients. We present this article in accordance with the TRIPOD reporting checklist (available at https://tgh.amegroups.com/article/view/10.21037/tgh-23-61/rc).
Methods
Patients
Peripheral blood samples were obtained from a total of 31 GICs patients between April 2019 and January 2021 at Zhongshan Hospital in this single-center retrospective study, including esophageal cancer patients (n=7), gastric cancer patients (n=20) and colorectal cancer patients (n=4). The study enrolled GIC patients with histologically confirmed unresectable disease or metastatic disease. Patients included in the study ranged from 19 to 80 years of age with Eastern Cooperative Oncology Group (ECOG) performance status of 0–2. Patients who had previously received immunotherapy were excluded. All patients were followed up and the median duration of patient follow-up was 11.9 months at the data cutoff of June 23th, 2021. Peripheral blood was collected and laboratory indices, including carcinoembryonic antigen (CEA) levels, neutrophil, platelet, lymphocyte and leukocyte counts were recorded within 7 days prior to the beginning of the first cycle of anti-PD-1 treatment. All subjects were recruited from the Fudan University Shanghai Cancer Center and proved by pathologic examination. Cross-sectional imaging of the chest, abdomen, and pelvis was obtained every three cycles. The objective response rate (ORR) and progression-free survival (PFS) were evaluated independently by physicians and two experienced radiologists according to immune-related response evaluation criteria in solid tumors (irRECIST) for each patient.
Peripheral blood mononuclear cells (PBMCs) were isolated by density gradient centrifugation using Ficoll-PaqueTM PLUS (GE Healthcare, NY, USA; Cat. No. 17-1440-03). The fresh PBMCs were lysed with Lysis buffer using the MagMAX™ mirVana™ Total RNA Isolation Kit (Thermo Fisher Scientific, Waltham, USA; Cat. No. A27828) and stored at −80 ℃ until further use. This study was conducted in accordance with the Declaration of Helsinki (as revised in 2013) and approved by Ethics Committee of Zhongshan Hospital Affiliated to Fudan University (approval number: B2018-219). All patients signed the written informed consents before participation.
TCRβ sequencing
For the Ion Torrent-based approach, ribonucleic acid (RNA) was extracted from PBMC using the MagMAX™ mirVana™ Total RNA Isolation Kit (Thermo Fisher Scientific Cat. No. A27828). Purified RNA samples were quantified using Qubit RNA HS Assay Kit (Thermo Fisher Scientific Cat. No. Q32852). The Agilent 2100 Bioanalyzer and Agilent RNA 6000 Nano Kit were used to quantify and evaluate RNA integrity. Fifty ng of total RNA was reverse transcribed using SuperScript IV VILO Master Mix (Thermo Fisher Scientific Cat. No. 11756050). For each sample, 25 ng cDNA was amplified using the Oncomine TCR Beta-LR Assay (Thermo Fisher Scientific Cat. No. A35386), and protocol as described in Oncomine™ Human Immune Repertoire User Guide MAN0017438 Revision C.0. Libraries were purified with Agencourt AMPure XP beads (Beckman Coulter, Pasadena, CA, USA; Cat. No. A63880), washed with 70% ethanol, and eluted in 50 µL Low TE buffer. Resulting library samples were diluted and quantified using the Ion Library Quantitation Kit (Thermo Fisher Scientific Cat. No. 4468802), then diluted to 25 pM with Low TE buffer. Equal volumes from 6–7 samples at a time were pooled together for sequencing on one Ion 530 chip, followed by analysis via Ion Reporter version 5.14.
Sequencing data analysis
The analysis process was shown in Figure S1. The raw sequencing data (.bcl) generated from the Ion Torrent S5 XL sequencer were demultiplexed and preprocessed using BaseCaller plugin (5.10) to produce sample-specific UBAM files. Briefly, the sequencing adaptors and target-specific primers were trimmed, before further trimming of the reads to retain only high-quality data (Q20 or higher). The run result files were then transferred to the Ion Reporter™ Software 5.14 using Ion Reporter Uploader plugin, followed by post-processing to filter low quality and off-target reads, remove or correct error containing reads, generate report for VDJ rearrangements and perform secondary analysis of repertoire features, including evenness and Shannon diversity index. Shannon diversity index was calculated as below:
where pi was the frequency of clonotype i for the sample with n unique clonotypes. Evenness was a normalized Shannon diversity index as indicated below (10,11):
All the analyses mentioned above (including Shannon diversity index and evenness) used the default parameters of Ion Torrent S5 XL Server or Ion Reporter™ Software 5.14, two well-established commercial software. As for DE50 calculation, we used our in-house workflow adapted from a previous report (12):
A schematic graph is provided to help understand the meaning of DE50 in Figure S2.
Statistical analysis
SPSS 26.0 software and GraphPad Prism 8.0 were used to perform the analysis. Statistical significance was declared based on P value <0.05 with two-sided test. The Kaplan-Meier was used to estimate PFS. To compare the continuous variable, such as curative effect between groups and TCR repertoire diversity, Mann-Whitney U test was used. Independent predictive factors for clinical efficacy were investigated by multivariable logistic regression models. Univariable logistic regression models with the objective response (OR) status as the binary outcome and sex, age, evenness, Shannon diversity, DE50, neutrophil-lymphocyte ratio (NLR), platelet-lymphocyte ratio (PLR), or CEA as the predictor were built. According to the P value (<0.05) of the outputs from the univariable logistic regression models, a multivariable logistic regression model was built with the OR status as the binary outcome and Shannon diversity, DE50, and CEA as the predictors. Cox proportional-hazards regression models were used to evaluate the relationship between PFS and each of the same variables as used in the univariable logistic regression models. A multivariable Cox regression analysis was then performed with selected variables DE50, PLR, and CEA according to the P value of the outputs from the univariable analyses. Both the univariable and the multivariable logistic regression were run using R with method = “glm”, family = “binomial” and link = “logit”. The Cox Proportional-Hazards Regression was fit in R with the survival package and its coxph function. All the forest plots were drawn in R with package “forestplot”. Variables with P<0.2 in univariable logistic regression models were included in a multivariable logistic regression model.
To ascertain independent prognostic factors, univariate Cox analysis, multivariate Cox analysis, and Kaplan-Meier survival analysis were utilized. A Cox proportional hazard regression model and a nomogram were constructed to forecast PFS. The predictive model was evaluated using the receiver operating characteristic (ROC) curve, concordance index (C-index), calibration curve, and decision curve analysis (DCA). Furthermore, the nomogram’s performance was internally validated through the random allocation of all cases into the training cohort and the validation cohort, with a 7:3 ratio.
Results
Characteristics of the patients
A total of 31 patients with GICs treated with PD-1 inhibitors were enrolled in this study. The demographic and baseline characteristics were depicted in Table 1. The majority of the patients were male (74.2%) with a median age of 64 years (range, 39–79 years) and an ECOG PS of 0–2 at baseline. Of the local GIC therapies, 36% of patients received anti-PD-1 antibody combined with anti-angiogenics, 19% received anti-PD-1 antibody combined with chemotherapy and 45% received anti-PD-1 monotherapy. For data analysis, partial response (PR, n=5) and stable disease (SD, n=14) were considered as treatment responders whereas progression disease (PD, n=12) groups are defined as non-responders. Survival plot for PFS was shown in Figure S3.
Table 1
Patient characteristics | Number |
---|---|
Sex, n (%) | |
Male | 23 (74.2) |
Female | 8 (25.8) |
Age, years; median [range] | 64 [39–79] |
Tumor types, n (%) | |
Esophageal cancer | 7 (22.6) |
Gastric cancer | 20 (64.5) |
Colorectal cancer | 4 (12.9) |
ECOG score, n (%) | |
0–1 | 23 (74.2) |
2 | 8 (25.8) |
Treatment line, n (%) | |
<3 | 19 (61.3) |
≥3 | 12 (38.7) |
PD-L1 agents, n (%) | |
22C3 | 8 (25.8) |
E1L3N | 5 (16.1) |
PD-L1 expression, n (%) | |
Negative | 6 (19.4) |
Positive* | 7 (22.6) |
Missing | 18 (58.1) |
CEA, n (%) | |
<5 μg/L | 18 (58.1) |
≥5 μg/L | 13 (41.9) |
*, PD-L1 positivity was defined as CPS ≥1. GIC, gastrointestinal cancer; ECOG, Eastern Cooperative Oncology Group; PD-L1, programmed death ligand-1; CEA, carcinoembryonic antigen; CPS, combined positive score.
Profiling of TCRβ CDR3 in all patients
A total of 31,531,729 TCRβ CDR3 aa sequence reads were produced from PBMCs of the 31 patients, with an average of 1,017,153 TCRβ CDR3 aa sequence reads per sample. Fifty-three distinct Vβ gene segments, 13 various Jβ gene segments, and 664 unique V-J pairs were identified in all of the samples. First, we examined the effects of pre-existing TCR repertoire characteristics from baseline PBMC on patient response to anti-PD-1 therapy. For the patient response outcomes, we classified patients into three groups based on the best response, that is, CR/PR, SD, and PD. As shown in Figure S4, we found that the number of distinct Vβ gene segments per sample in SD + PR group was slightly higher than that in PD group (Mann-Whitney test, P=0.0236). The most frequent Vβ gene segments in all of the samples were T-cell receptor beta variable region (TRBV) 20.1 (8.17%±0.38%), TRBV28 (6.87%±0.50%), TRBV7.2 (6.24%±0.45%), TRBV5.1(5.54%±0.33%) and TRBV29.1 (5.32%±0.32%) (Figure S4A). Thirteen various Jβ gene segments were detected in every single sample, with the most frequent Jβ gene segments being T cell receptor beta joining (TRBJ) 2.1(18.17%±0.49%), TRBJ2.7 (16.78%±0.65%), TRBJ2.3 (12.11%±0.60%), TRBJ1.1 (11.29%±0.45%), TRBJ2.5 (9.33%±0.41%) (Figure S4B). Using R package DESeq2 to analyze the significantly differentially expressed genes in VJ Usage between PD group and PR + SD group, where the P value was set to 0.05 and fold change was set to 1.2, a total of 55 significantly differentially expressed genes were obtained, among which 33 genes were upregulated and 22 gene was downregulated. A heat map was drawn for the clustering analysis (Figure S4C).
TCR diversity is predictive for immune checkpoint inhibitors (ICIs) benefit
In order to identify the potential predictors of clinical outcomes of anti-PD-1 therapy, we also analyzed the correlation between diversity indices (Shannon diversity, evenness and DE50) and response to treatment using Chi-square test (Figure 1). There was no significant difference in Shannon diversity between responders (PR + SD) and non-responders (12.56 vs. 11.72, P=0.1905) (Figure1A). However, evenness value in responders was higher than that in non-responders (0.8875 vs. 0.8349, P=0.0392) (Figure 1B). The DE50 score quantifies the proportion of rearrangements required to explain the most frequent rearrangements accounting for 50% of cumulative sum of each rearrangement’s frequency, relative to the total number of rearrangements. A TCR repertoire exhibiting an even frequency distribution of V-J rearrangements is indicative of low clonality and consequently yields a higher DE50 score (12). We further investigated the DE50 scores, and observed a higher DE50 score in responders compared to non-responders (0.09334 vs. 0.04254, P=0.018) (Figure 1C).
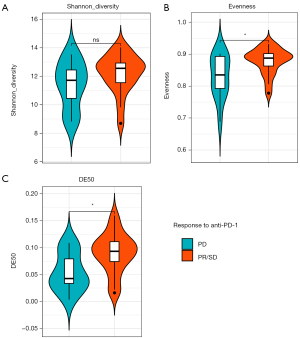
To further confirm the relationship between baseline clinical parameters (including TCR diversity) and anti-PD-1 therapy response, univariable and multivariable logistic regression analyses were performed using median as the cut-off of the evenness (5.2) and Shannon diversity (3.62), respectively. The univariable model identified baseline DE50 [odds ratio (OR) =0.15, 95% confidence interval (CI): 0.03–0.78, P=0.024] as the only variable significantly associated with response to therapy (Figure 2A). In the multivariable logistic regression analyses where variables with P values <0.2 were included. Patient with higher DE50 levels tended to enjoy better response to anti-PD-1 therapy, although difference did not reach a significant level (OR =0.21, 95% CI: 0.03–1.32, P=0.096) (Figure 2B).
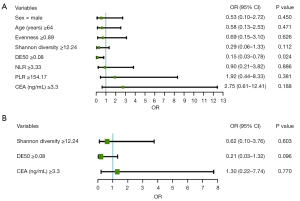
DE50 was an independently prognostic factor in GIC treated with anti-PD-1 antibody
To further explore the relationship between the baseline TCR diversity and PFS, Kaplan-Meier survival analyses were performed. In the included cohort, all the patients were divided into high- and low-TCR diversity groups using median as the cut-off the evenness (0.89) and Shannon diversity (12.24), respectively. As a result, the Kaplan-Meier analysis showed that the patients with either high-evenness diversity or Shannon diversity failed to enjoy a favorable PFS (P=0.77 and P=0.53, respectively) (Figure 3A,3B). However, patients with higher baseline DE50 had significantly longer PFS than patients with low baseline DE50 (P=0.0022) (Figure 3C). Thus, in order to assess the independent predictive value of DE50, univariable and multivariable Cox regression analyses were performed. In the Cox proportional hazards regression analysis, we discovered that baseline DE50 [hazard ratio (HR) =0.24, 95% CI: 0.09–0.64, P=0.005] and PLR (HR =2.46, 95% CI: 1.04–5.79, P=0.040) as the only two variables significantly associated with PFS (Figure 4A). After adjustment for age, gender, tumor site and histological type, to our surprise, DE50 (HR =0.22, 95% CI: 0.08–0.64, P=0.006) and PLR (HR =2.73, 95% CI: 1.15–6.45, P=0.022) were found to be independent factors associated with PFS (Figure 4B). Furthermore, DE50 (C-index =0.670) and NLR (C-index =0.628) had higher discrimination ability in the prediction of PFS than the remaining factors, including CEA with a C-index of 0.577.
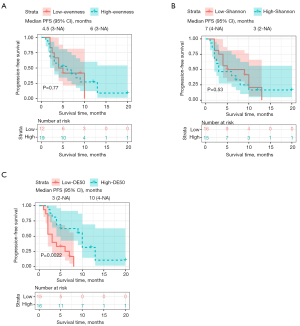

Baseline DE50 levels, combined with PLR and CEA, was able to better predict PFS for patients with GIC treated with anti-PD-1 antibody
The above results indicated that the level of DE50 and PLR were independent prognostic factors for improvement of PFS in patients with GIC treated with anti-PD-1 antibody. Since serum CEA is recommended as a tumor marker in GIC for tumor detecting and monitoring response to therapy. A nomogram for prediction of survival probabilities, which included DE50 levels, PLR and CEA were constructed (Figure 5). ROC curve was used to analyze the power of nomogram to predict PFS among GIC patients treated with anti-PD-1 antibody. According to the ROC analysis, the area under the curves (AUCs) of this system at 3-, 6- and 12-month PFS reached 0.825, 0.802, and 0.954, respectively (Figure 6A). The nomogram had a C-index of 0.768 (95% CI: 0.658–0.879). Meanwhile, the calibration curves demonstrated considerable agreement between the nomogram predicted and actual survival at 3-, 6- and 12-month PFS, respectively (Figure 6B). These validations suggesting that this model can accurately predict the possibility of PFS among GIC patients treated with anti-PD-1 antibody. In addition to address the accuracy, DCA was introduced to evaluate the clinical utility of this nomogram. Figure 6C showed that the established nomogram had favorable clinical applicability in predicting PFS. To validate the nomogram model, the internal validation data was used to evaluate the accuracy and calibrate the model (Figure S5). The adjusted C-index was 0.804 (95% CI: 0.679–0.929) after 100 bootstrapping internal validations, demonstrating a good discrimination power.
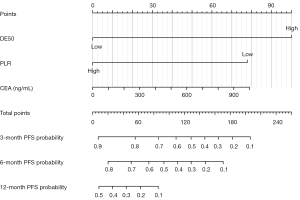
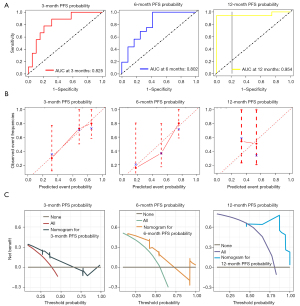
Furthermore, to establish a risk stratification system based on our nomogram, we calculated the total score for each patient in the training cohort, and then stratified the patients according to the median of total scores into two subgroups: low-risk group and high-risk group. Kaplan-Meier analysis indicated that the survival time of patients in the high-risk group was much shorter than that of the low-risk group (Figure 7A). Furthermore, the risk score reached an AUC value of 0.825, 0.802, and 0.954 for 3-, 6- and 12-month PFS, respectively (Figure 7B).
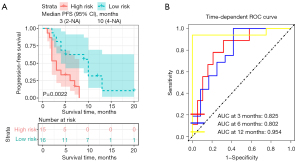
Discussion
Recently, anti-PD-1 antibodies have been approved for the treatment of GICs, exhibiting encouraging outcomes (2,13,14). However, patients’ responses are often unpredictable. Hence, reliable biomarkers are needed to identify patients who might benefit from PD-1 inhibitors (13,15-18).
T-cell-mediated cellular immunity plays an important role in antitumor responses to immunotherapy (19). The TCR is an essential membrane protein that can recognize specific antigens on tumor and participate in the activation of T-cells. Monitoring TCR repertoire diversity may be helpful in assessing the immune therapy efficacy and prognosis. The antigen-specificity of tumor-reactive T-cells in peripheral blood and tumor tissue seem to be very much alike (20,21). Liquid biopsy is non-invasive and has become an alternative choice in clinical practice. Recent advances in next-generation sequencing (NGS) technology provide a detailed and complete description of multiple TCR clones in order to determine the diversity of TCR in the repertoire. Here, our study sought to use multiplex PCR amplification and deep sequencing of CDR3 region to assess TCR repertoire profiling in the peripheral blood.
Previous studies have demonstrated that pre-treatment TCR repertoire diversity might be associated with clinical outcome of immune therapy (12,21-26). However, the results of these studies are sometimes not consistent or even contradictory. These results highlight the need for more detailed studies regarding some particular tumor type. To our knowledge, little is known about clinical significance of peripheral TCR repertoire profiling in patients with GIC treated with anti-PD-1 antibody. In the present study, we demonstrated that higher DE50 scores were associated with better prognosis and increased response to anti-PD-1 inhibitors in GICs. Importantly, this association was independent of other clinical factors such as age, gender, CEA. A possible explanation for these results is that higher TCR diversity may provide greater opportunities for our adaptive immune system to recognize antigens, increasing the possibility of more tumor-specific T cells to be activated to eliminate tumor cells (23,24,27). Conversely, low TCR diversity may be associated with the impaired immune status that contribute to poor immunotherapy response (23,24,28).
In order to obtain better predictive power, a combined model based on peripheral DE50 score and PLR is developed in this study at the pre-treatment time point. PLR is a routinely systemic inflammatory marker and may be a significant factor for predicting survival and response to therapies in cancer (29-31). Thrombocytosis and the release of platelet‐derived chemokines in the tumor microenvironment may promote tumor progression (32). In contrast, lymphocytes are associated with immune surveillance and prevent development of malignancy (33). Therefore, thrombocytosis and lymphocytopenia have been suggested as negative prognostic markers in various cancers (30,31). On this basis, we speculated that the biomarker combining PLR and DE50, may better reflect the information of inflammatory/immune system status and predict the prognosis of GICs patients. The result suggested that such combinations of two different biomarkers could help to more accurately identify patients who may benefit from anti-PD-1 treatment strategies in GICs. Further prospective research is needed to validate and refine the model.
There are some limitations in this study. First, this is a small study with a limited number of patients. Second, the study only focused on the β chain of the TCR, which can not entirely represent the characteristics of the whole TCR repertoire. The third limitation in this study was a lack of dynamic changes of TCR repertoire profiles during tumor treatment and evolution as well as a lack of longer follow-up. Further study with a larger cohort and longer follow-up period would be required to validate these findings thus provide significant insight in the immunotherapy of GICs.
Conclusions
TCR repertoire profiling could serve as a useful indicator for predicting treatment response to anti-PD-1 immunotherapy and prognosis in GICs. In addition, DE50 was an independent predictive factor for PFS according to multivariable Cox regression analysis. Notably, these findings could be utilized to direct future immunotherapy if validated in further prospective studies.
Acknowledgments
We gratefully acknowledge the support from Medical Oncology Department of Zhongshan Hospital. We also express our sincere thanks to the patients who participated in this study.
Funding: This study was funded by Shanghai Municipal Natural Science Foundation (No. 21SQBS00200).
Footnote
Reporting Checklist: The authors have completed the TRIPOD reporting checklist. Available at https://tgh.amegroups.com/article/view/10.21037/tgh-23-61/rc
Data Sharing Statement: Available at https://tgh.amegroups.com/article/view/10.21037/tgh-23-61/dss
Peer Review File: Available at https://tgh.amegroups.com/article/view/10.21037/tgh-23-61/prf
Conflicts of Interest: All authors have completed the ICMJE uniform disclosure form (available at https://tgh.amegroups.com/article/view/10.21037/tgh-23-61/coif). B.L., L.C., C.L. and H.P. are employees from Shanghai Dunwill Medical Technology Co., Ltd. and the company is not involved in this study. The other authors have no conflicts of interest to declare.
Ethical Statement: The authors are accountable for all aspects of the work in ensuring that questions related to the accuracy or integrity of any part of the work are appropriately investigated and resolved. This study was conducted in accordance with the Declaration of Helsinki (as revised in 2013) and approved by Ethics Committee of Zhongshan Hospital Affiliated to Fudan University (approval number: B2018-219). All patients signed the written informed consents before participation.
Open Access Statement: This is an Open Access article distributed in accordance with the Creative Commons Attribution-NonCommercial-NoDerivs 4.0 International License (CC BY-NC-ND 4.0), which permits the non-commercial replication and distribution of the article with the strict proviso that no changes or edits are made and the original work is properly cited (including links to both the formal publication through the relevant DOI and the license). See: https://creativecommons.org/licenses/by-nc-nd/4.0/.
References
- Vaseghi Maghvan P, Rezaei-Tavirani M, Zali H, et al. Network analysis of common genes related to esophageal, gastric, and colon cancers. Gastroenterol Hepatol Bed Bench 2017;10:295-302. [PubMed]
- Güthle M, Ettrich T, Seufferlein T. Immunotherapy in Gastrointestinal Cancers. Visc Med 2020;36:231-7. [Crossref] [PubMed]
- Davis MM, Bjorkman PJ. T-cell antigen receptor genes and T-cell recognition. Nature 1988;334:395-402. [Crossref] [PubMed]
- Hodges E, Krishna MT, Pickard C, et al. Diagnostic role of tests for T cell receptor (TCR) genes. J Clin Pathol 2003;56:1-11. [Crossref] [PubMed]
- Morris GP, Allen PM. How the TCR balances sensitivity and specificity for the recognition of self and pathogens. Nat Immunol 2012;13:121-8. [Crossref] [PubMed]
- Sherwood AM, Emerson RO, Scherer D, et al. Tumor-infiltrating lymphocytes in colorectal tumors display a diversity of T cell receptor sequences that differ from the T cells in adjacent mucosal tissue. Cancer Immunol Immunother 2013;62:1453-61. [Crossref] [PubMed]
- Jia Q, Zhou J, Chen G, et al. Diversity index of mucosal resident T lymphocyte repertoire predicts clinical prognosis in gastric cancer. Oncoimmunology 2015;4:e1001230. [Crossref] [PubMed]
- Wang H, Yuan Y, Lu C, et al. Analysis of T-cell receptor repertoire in peripheral blood of patients with pancreatic cancer and other pancreatic diseases. J Cell Mol Med 2021;25:3991-4000. [Crossref] [PubMed]
- Ji S, Li J, Chang L, et al. Peripheral blood T-cell receptor repertoire as a predictor of clinical outcomes in gastrointestinal cancer patients treated with PD-1 inhibitor. Clin Transl Oncol 2021;23:1646-56. [Crossref] [PubMed]
- Zhang L, Cham J, Paciorek A, et al. 3D: diversity, dynamics, differential testing - a proposed pipeline for analysis of next-generation sequencing T cell repertoire data. BMC Bioinformatics 2017;18:129. [Crossref] [PubMed]
- Looney TJ, Topacio-Hall D, Lowman G, et al. TCR Convergence in Individuals Treated With Immune Checkpoint Inhibition for Cancer. Front Immunol 2019;10:2985. [Crossref] [PubMed]
- Hogan SA, Courtier A, Cheng PF, et al. Peripheral Blood TCR Repertoire Profiling May Facilitate Patient Stratification for Immunotherapy against Melanoma. Cancer Immunol Res 2019;7:77-85. [Crossref] [PubMed]
- Zeng Z, Yang B, Liao Z. Biomarkers in Immunotherapy-Based Precision Treatments of Digestive System Tumors. Front Oncol 2021;11:650481. [Crossref] [PubMed]
- Mizrahi J, Pant S. Immunotherapy in Gastrointestinal Malignancies. Adv Exp Med Biol 2020;1244:93-106. [Crossref] [PubMed]
- Fuchs CS, Doi T, Jang RW, et al. Safety and Efficacy of Pembrolizumab Monotherapy in Patients With Previously Treated Advanced Gastric and Gastroesophageal Junction Cancer: Phase 2 Clinical KEYNOTE-059 Trial. JAMA Oncol 2018;4:e180013. Erratum in: JAMA Oncol 2019;5:579. [Crossref] [PubMed]
- Wang F, Wei XL, Wang FH, et al. Safety, efficacy and tumor mutational burden as a biomarker of overall survival benefit in chemo-refractory gastric cancer treated with toripalimab, a PD-1 antibody in phase Ib/II clinical trial NCT02915432. Ann Oncol 2019;30:1479-86. [Crossref] [PubMed]
- Samstein RM, Lee CH, Shoushtari AN, et al. Tumor mutational load predicts survival after immunotherapy across multiple cancer types. Nat Genet 2019;51:202-6. [Crossref] [PubMed]
- Kim RD, Kovari BP, Martinez M, et al. A phase I/Ib study of regorafenib and nivolumab in mismatch repair proficient advanced refractory colorectal cancer. Eur J Cancer 2022;169:93-102. [Crossref] [PubMed]
- Xia A, Zhang Y, Xu J, et al. T Cell Dysfunction in Cancer Immunity and Immunotherapy. Front Immunol 2019;10:1719. [Crossref] [PubMed]
- Schumacher TN, Scheper W. A liquid biopsy for cancer immunotherapy. Nat Med 2016;22:340-1. [Crossref] [PubMed]
- Akyüz N, Brandt A, Stein A, et al. T-cell diversification reflects antigen selection in the blood of patients on immune checkpoint inhibition and may be exploited as liquid biopsy biomarker. Int J Cancer 2017;140:2535-44. [Crossref] [PubMed]
- Hopkins AC, Yarchoan M, Durham JN, et al. T cell receptor repertoire features associated with survival in immunotherapy-treated pancreatic ductal adenocarcinoma. JCI Insight 2018;3:e122092. [Crossref] [PubMed]
- Snyder A, Nathanson T, Funt SA, et al. Contribution of systemic and somatic factors to clinical response and resistance to PD-L1 blockade in urothelial cancer: An exploratory multi-omic analysis. PLoS Med 2017;14:e1002309. [Crossref] [PubMed]
- Liu YY, Yang QF, Yang JS, et al. Characteristics and prognostic significance of profiling the peripheral blood T-cell receptor repertoire in patients with advanced lung cancer. Int J Cancer 2019;145:1423-31. [Crossref] [PubMed]
- Guo L, Bi X, Li Y, et al. Characteristics, dynamic changes, and prognostic significance of TCR repertoire profiling in patients with renal cell carcinoma. J Pathol 2020;251:26-37. [Crossref] [PubMed]
- Han J, Duan J, Bai H, et al. TCR Repertoire Diversity of Peripheral PD-1(+)CD8(+) T Cells Predicts Clinical Outcomes after Immunotherapy in Patients with Non-Small Cell Lung Cancer. Cancer Immunol Res 2020;8:146-54. [Crossref] [PubMed]
- Price DA, West SM, Betts MR, et al. T cell receptor recognition motifs govern immune escape patterns in acute SIV infection. Immunity 2004;21:793-803. [Crossref] [PubMed]
- Chen DS, Mellman I. Elements of cancer immunity and the cancer-immune set point. Nature 2017;541:321-30. [Crossref] [PubMed]
- Toyokawa T, Muguruma K, Yoshii M, et al. Clinical significance of prognostic inflammation-based and/or nutritional markers in patients with stage III gastric cancer. BMC Cancer 2020;20:517. [Crossref] [PubMed]
- Fan X, Wang D, Zhang W, et al. Inflammatory Markers Predict Survival in Patients With Advanced Gastric and Colorectal Cancers Receiving Anti-PD-1 Therapy. Front Cell Dev Biol 2021;9:638312. [Crossref] [PubMed]
- Qi Y, Liao D, Fu X, et al. Elevated platelet-to-lymphocyte corresponds with poor outcome in patients with advanced cancer receiving anti-PD-1 therapy. Int Immunopharmacol 2019;74:105707. [Crossref] [PubMed]
- Sierko E, Wojtukiewicz MZ. Platelets and angiogenesis in malignancy. Semin Thromb Hemost 2004;30:95-108. [Crossref] [PubMed]
- Dunn GP, Old LJ, Schreiber RD. The immunobiology of cancer immunosurveillance and immunoediting. Immunity 2004;21:137-48. [Crossref] [PubMed]
Cite this article as: Wu J, Yu Y, Zhang S, Zhang P, Yu S, Li W, Wang Y, Li Q, Lu B, Chen L, Luo C, Peng H, Liu T, Cui Y. Clinical significance of peripheral T-cell receptor repertoire profiling and individualized nomograms in patients with gastrointestinal cancer treated with anti-programmed death 1 antibody. Transl Gastroenterol Hepatol 2024;9:5.