Artificial intelligence and computer-aided diagnosis for colonoscopy: where do we stand now?
Introduction
Colorectal cancer (CRC) is the third most common cancer and the fourth leading cause of death from cancer worldwide (1). With the early detection and removal of neoplastic lesions, CRC is considered to be efficacy prevented (2). However, a meta-analysis including six studies on patients undergoing two same-day colonoscopies showed a pooled miss rate of 22% for colorectal polyps (3) and post-colonoscopy CRC is reported to account for about 8.6% of all the CRC (4) Low adenoma detection rates (ADRs) and incompletely resected are recognized as main causes of this kind of CRC (5,6). To overcome this situation, numerous attempts have been adopted to improve ADR including educational interventions, enhanced imaging techniques and mechanical devices to improve mucosal exposure. Computer-aided diagnosis (CAD) systems using AI have been expected to be a new modality that can improve ADR (7). The major roles of CAD in colonoscopy include automated detection (Figure 1) and pathological prediction (i.e., optical biopsy) of colorectal polyps during real-time endoscope (8).
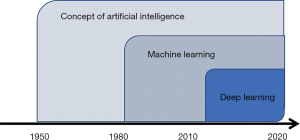
Definition of AI
Figure 2 illustrates the general concept of AI, machine learning, and deep learning (DL). AI has been basically designed to imitate human’s thinking way and show intelligence similar to that of human beings. The concept of AI was firstly presented at the Dartmouth Conference held in 1956 by McCarthy et al. (9) Machine learning is a type of AI, which allows automated learning on data sets without the need for explicit programming of prediction rules (10). DL approaches, one of the advanced machine learning methods, have been revolutionizing the area by applying artificial neural networks (11). The DL algorithm was inspired by the concept of neurons and synapses in the human brain to discover image features that optimally represent the data for a specific task. DL is currently considered as one of the most prominent prediction methods in the AI field.
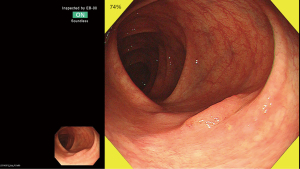
Computer-aided characterization of colorectal lesions
CAD for polyp characterization is generally designed to predict lesion pathology with help of machine learning based algorithm. Various imaging technologies are considered as the targets for CAD for polyp characterization such as white-light endoscopy (12,13), magnifying narrow band imaging (NBI) (14,15), magnifying chromoendoscopy (16), endocytoscopy (17-20), confocal laser endomicroscopy (21) and laser-induced fluorescence spectroscopy (22-25) (Table 1).
Table 1
Reference | Journal | Year | Endoscopic modality | Study design | Subjects | Outcomes |
---|---|---|---|---|---|---|
Chen et al. (15) | Gastroenterology | 2018 | Magnifying NBI | Retrospective study | 284 lesions | 96.3% sensitivity and 78.1% specificity for adenomas |
Mori et al. (17) | Ann Intern Med | 2018 | Endocytoscopy | Prospective study | 791 patients with 466 lesions | 92.7% sensitivity and 89.8% specificity for adenomas |
Renner et al. (26) | Scand J Gastroenterol | 2018 | White light endoscopy and non-magnifying NBI | Retrospective study | 100 polyps | 92.3% sensitivity and 62.5% specificity for adenomas |
Ito et al. (27) | Oncology | 2019 | White light endoscopy | Retrospective study | 190 images | 67.5% sensitivity and 89.0% specificity for deeply invasive submucosal cancer (cross validation) |
Byrne et al. (28) | Gut | 2019 | Magnifying NBI | Retrospective study | 125 lesions | 98% sensitivity and 83% specificity for adenomas |
Horiuchi et al. (29) | Scand J Gastroenterol | 2019 | Autofluorescence imaging | Prospective study | 95 patients with 258 lesions | 80.0% sensitivity and 95.3% specificity for adenomas |
Sánchez-Montes et al. (13) | Endoscopy | 2019 | White light endoscopy and non-magnifying NBI | Retrospective study | 225 polyps | 92.3% sensitivity and 89.2% specificity for adenomas |
Magnifying NBI
The application of CAD to magnifying NBI was firstly reported by Tischendorf et al. (14) and Gross et al. (30) in 2010 and 2011, respectively. In their model, nine vessel features such as length, brightness, diameter, and others, were extracted from magnified NBI images and used for machine learning referring corresponding pathological diagnoses as ground truth. Their developed model provided an accuracy of 85.3% in differentiation between neoplastic and non-neoplastic polyps. However, these studies were based on off-site assessment of already captured images. Subsequently, Japanese research team (31,32) reported the differently designed model based on machine learning and validated it in a real-time clinical practice, succeeding in vivo classification of polyps during endoscopy with an accuracy of over 90%. However, generalization of the study result is still required because of the limited number of the included patients (i.e., 41 patients) of the validation study.
Endocytoscopy
Endocytoscopy (EC, CF-H290ECI; Olympus Corp, Tokyo, Japan) allows in vivo visualization of cellular imaging with ultra-magnifying power of 520-fold) has also been investigated as an attractive target for CAD in colonoscopy. EC is considered one of the ideal modalities for CAD, because it realizes focused, consistent images of fixed size that enable easier image analysis for AI. Several pilot studies including one large-scale prospective study demonstrated an approximately 90% accuracy of EC for identification of adenomas with only a 0.4-second latency after capturing an image (18-20,33,34) (Figure 3).
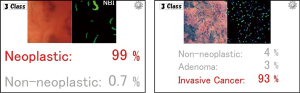
Laser-induced fluorescence spectroscopy
Rath et al. (23) prospectively investigated the performance of real-time running CAD for laser-induced fluorescence spectroscopy (WavSTAT4; Pentax Corp., Tokyo, Japan), reporting 100% sensitivity, 80.6% specificity for diminutive colorectal adenomas. On the other hand, Kuiper et al. (22) demonstrated less-impressive results, with 83.0% sensitivity, 59.7% specificity, 71.6% for diminutive adenomas, thus further assessment would be required to clarify the clinical usefulness of this modality.
White light endoscopy
CAD for white-light endoscopy has not been the hottest research topic though it is the most available endoscopic modality, because white-light endoscopy is considered to have limitation in its accuracy of optical diagnosis of colorectal lesions (8). Recently, Komeda et al. (12) developed a deep-learning model, providing 75.1% accuracy with a cross-validation method, while Sánchez-Montes et al. (13) developed a handcrafted, predictive model based on 3 measures (contrast, tubularity, and branching) of the polyp surface pattern, resulting in 95.0% sensitivity, 87.9% specificity, 82.6% PPV, and 96.7% NPV for diminutive rectosigmoid adenomas. However, there has been no study evaluating the real-time use of CAD for white light endoscopy.
Advantages of AI
Some colorectal lesions including flat and depressed neoplasia are endoscopically subtle but sometimes harbor advanced histopathology (35). These lesions including sessile serrated lesions are sometime difficult to identify its histopathology even with experienced endoscopists’ eyes (36). Therefore, the major goal of CAD for colonoscopy is to predict the histology of these kinds of “difficult” polyps and help endoscopists perform appropriate treatment options during ongoing endoscopy. Given precise identification of polyp histology particularly for diminutive (≤5 mm) polyps is allowed with use of CAD, the resect-and-discard strategy can also be implemented, leading to significant reduction in costs related to unnecessary polypectomy and pathological assessment (37,38).
The optical biopsy of the lesions suspected of the early invasive cancers or the prediction of depth of invasion is another important research topic (Figure 3). CAD will be able to play significant role in differentiating “endoscopically curative” lesions; endoscopic treatments can be considered curative for lesions with superficial invasion into submucosal layer, while surgical resection is recommended for those with deeply invasive sub-mucosal cancers. Validated classification systems based on advanced imaging using magnifying chromoendoscopy and NBI have been developed to predict cancer invasion into deep submucosal layer, however these classification including the Kudo pit pattern classification, Sano capillary pattern classification, Hiroshima classification, and NBI International Colorectal Endoscopic Classification allow highly accurate prediction only in limited situations (e.g., effective only when used in tertiary care centers or with experts’ hands) (39). Therefore, use of AI can be an attractive option for predicting such “difficult-to-diagnose” lesions in near future.
Disadvantages of CAD
Nowadays, AI is becoming one of the hottest research topics in medical fields, attracting the interest of many medical doctors, however, from the perspective of clinical application, we should not ignore the weak points and limitations which the AI potentially harbors.
Firstly, increased time required for endoscopy examination can be a limitation of practice. A prospective study investigating AI for endocytoscopy by Mori et al. (17) pointed out that additional 35–47 s will be required to assess a polyp. Secondly, AI’s output displayed in the monitor might distract endoscopists attention, resulting in missing and/or misdiagnosis of the target/different lesions. Thirdly, misdiagnosis from CAD sometimes affects endoscopists decision in a bad way even if the initial diagnosis based on endoscopists’ visual inspection is correct. Fourthly, dependence on such machine-aided diagnosis possibly makes the new generation of endoscopists less skillful.
To overcome these drawbacks of AI, some measures can be proposed. Firstly, education programs regarding how to use and interpret the outputs from AI would be mandatory. At least, endoscopists should understand how wrong predictions by AI can affect endoscopists’ final diagnoses and decision. In addition, potential legal issues should be shared via these training program; legal responsibilities basically belong to the users rather than AI or companies developing AI. On the other hand, CAD can be a valuable tool for training of less experienced doctors as they are always able to compare their own optical diagnosis with outputs of CAD during ongoing colonoscopy.
Conclusions
Strong collaborations between clinicians and computer scientists has been contributing to overcome translational barriers that AI in colonoscopy harbors and it is now being implemented into clinical colonoscopy; actually, a couple of AI medical devices have already secured regulatory approval and are commercially available depending on the countries. Of course, clinician’s acceptance of these newly developed devices is crucial for wider implementation, because it may change a bit clinical procedure workflow. At the same time, robust clinical trials will be required to demonstrate improvements in performance, because currently available clinical studies provide very limited evidence of AI in colonoscopy. However, with increasing industry involvement and governmental incentives, AI will be rapidly implemented into colonoscopy practice in the next few years.
Acknowledgments
Funding: None.
Footnote
Provenance and Peer Review: This article was commissioned by the Guest Editors (Krish Ragunath, Philip WY Chiu) for the series “Advanced Endoscopic Imaging of the GI Tract” published in Translational Gastroenterology and Hepatology. The article has undergone external peer review.
Conflicts of Interest: All authors have completed the ICMJE uniform disclosure form (available at http://dx.doi.org/10.21037/tgh.2019.12.14). The series “Advanced Endoscopic Imaging of the GI Tract” was commissioned by the editorial office without any funding or sponsorship. YM and MM received speaking honorarium from Olympus Corporation. KM received research grant from Cybernet Corporation. The authors have no other conflicts of interest to declare.
Ethical Statement: The authors are accountable for all aspects of the work in ensuring that questions related to the accuracy or integrity of any part of the work are appropriately investigated and resolved.
Open Access Statement: This is an Open Access article distributed in accordance with the Creative Commons Attribution-NonCommercial-NoDerivs 4.0 International License (CC BY-NC-ND 4.0), which permits the non-commercial replication and distribution of the article with the strict proviso that no changes or edits are made and the original work is properly cited (including links to both the formal publication through the relevant DOI and the license). See: https://creativecommons.org/licenses/by-nc-nd/4.0/.
References
- Torre LA, Bray F, Siegel RL, et al. Global cancer statistics, 2012. CA Cancer J Clin 2015;65:87-108. [Crossref] [PubMed]
- Winawer SJ, Zauber AG, Ho MN, et al. Prevention of colorectal cancer by colonoscopic polypectomy. The National Polyp Study Workgroup. N Engl J Med 1993;329:1977-81. [Crossref] [PubMed]
- van Rijn JC, Reitsma JB, Stoker J, et al. Polyp miss rate determined by tandem colonoscopy: a systematic review. Am J Gastroenterol 2006;101:343-50. [Crossref] [PubMed]
- Morris EJ, Rutter MD, Finan PJ, et al. Post-colonoscopy colorectal cancer (PCCRC) rates vary considerably depending on the method used to calculate them: a retrospective observational population-based study of PCCRC in the English National Health Service. Gut 2015;64:1248-56. [Crossref] [PubMed]
- le Clercq CM, Bouwens MW, Rondagh EJ, et al. Postcolonoscopy colorectal cancers are preventable: a population-based study. Gut 2014;63:957-63. [Crossref] [PubMed]
- Kaminski MF, Regula J, Kraszewska E, et al. Quality indicators for colonoscopy and the risk of interval cancer. N Engl J Med 2010;362:1795-803. [Crossref] [PubMed]
- Byrne MF, Shahidi N, Rex DK. Will Computer-Aided Detection and Diagnosis Revolutionize Colonoscopy? Gastroenterology 2017;153:1460-4.e1. [Crossref] [PubMed]
- Mori Y, Kudo SE, Berzin TM, et al. Computer-aided diagnosis for colonoscopy. Endoscopy 2017;49:813-9. [Crossref] [PubMed]
- McCarthy J, Minsky ML, Rochester N, et al. A proposal for the dartmouth summer research project on artificial intelligence, August 31, 1955. AI Mag 2006;27:12-4.
- Murphy KP. Machine learning: a probabilistic perspective. Cambridge: MIT Press, 2012.
- Chartrand G, Cheng PM, Vorontsov E, et al. Deep Learning: A Primer for Radiologists. Radiographics 2017;37:2113-31. [Crossref] [PubMed]
- Komeda Y, Handa H, Watanabe T, et al. Computer-Aided Diagnosis Based on Convolutional Neural Network System for Colorectal Polyp Classification: Preliminary Experience. Oncology 2017;93:30-4. [Crossref] [PubMed]
- Sánchez-Montes C, Sanchez FJ, Bernal J, et al. Computer-aided prediction of polyp histology on white light colonoscopy using surface pattern analysis. Endoscopy 2019;51:261-5. [Crossref] [PubMed]
- Tischendorf JJ, Gross S, Winograd R, et al. Computer-aided classification of colorectal polyps based on vascular patterns: a pilot study. Endoscopy 2010;42:203-7. [Crossref] [PubMed]
- Chen PJ, Lin MC, Lai MJ, et al. Accurate Classification of Diminutive Colorectal Polyps Using Computer-Aided Analysis. Gastroenterology 2018;154:568-75. [Crossref] [PubMed]
- Takemura Y, Yoshida S, Tanaka S, et al. Quantitative analysis and development of a computer-aided system for identification of regular pit patterns of colorectal lesions. Gastrointest Endosc 2010;72:1047-51. [Crossref] [PubMed]
- Mori Y, Kudo SE, Misawa M, et al. Real-Time Use of Artificial Intelligence in Identification of Diminutive Polyps During Colonoscopy: A Prospective Study. Ann Intern Med 2018;169:357-66. [Crossref] [PubMed]
- Misawa M, Kudo SE, Mori Y, et al. Characterization of Colorectal Lesions Using a Computer-Aided Diagnostic System for Narrow-Band Imaging Endocytoscopy. Gastroenterology 2016;150:1531-2.e3. [Crossref] [PubMed]
- Mori Y, Kudo SE, Chiu PW, et al. Impact of an automated system for endocytoscopic diagnosis of small colorectal lesions: an international web-based study. Endoscopy 2016;48:1110-8. [Crossref] [PubMed]
- Takeda K, Kudo S, Mori Y, et al. Accuracy of diagnosing invasie colorectal cancer using computer-aided endocytoscopy. Endoscopy 2017;49:798-802. [Crossref] [PubMed]
- Ştefănescu D, Streba C, Cârţână ET, et al. Computer Aided Diagnosis for Confocal Laser Endomicroscopy in Advanced Colorectal Adenocarcinoma. PLoS One 2016;11:e0154863 [Crossref] [PubMed]
- Kuiper T, Alderlieste YA, Tytgat KM, et al. Automatic optical diagnosis of small colorectal lesions by laser-induced autofluorescence. Endoscopy 2015;47:56-62. [PubMed]
- Rath T, Tontini GE, Vieth M, et al. In vivo real-time assessment of colorectal polyp histology using an optical biopsy forceps system based on laser-induced fluorescence spectroscopy. Endoscopy 2016;48:557-62. [Crossref] [PubMed]
- Aihara H, Saito S, Inomata H, et al. Computer-aided diagnosis of neoplastic colorectal lesions using 'real-time' numerical color analysis during autofluorescence endoscopy. Eur J Gastroenterol Hepatol 2013;25:488-94. [Crossref] [PubMed]
- Aihara H, Sumiyama K, Saito S, et al. Numerical analysis of the autofluorescence intensity of neoplastic and non-neoplastic colorectal lesions by using a novel videoendoscopy system. Gastrointest Endosc 2009;69:726-33. [Crossref] [PubMed]
- Renner J, Phlipsen H, Haller B, et al. Optical classification of neoplastic colorectal polyps - a computer-assisted approach (the COACH study). Scand J Gastroenterol 2018;53:1100-6. [Crossref] [PubMed]
- Ito N, Kawahira H, Nakashima H, et al. Endoscopic Diagnostic Support System for cT1b Colorectal Cancer Using Deep Learning. Oncology 2019;96:44-50. [Crossref] [PubMed]
- Byrne MF, Chapados N, Soudan F, et al. Real-time differentiation of adenomatous and hyperplastic diminutive colorectal polyps during analysis of unaltered videos of standard colonoscopy using a deep learning model. Gut 2019;68:94-100. [Crossref] [PubMed]
- Horiuchi H, Tamai N, Kamba S, et al. Real-time computer-aided diagnosis of diminutive rectosigmoid polyps using an auto-fluorescence imaging system and novel color intensity analysis software. Scand J Gastroenterol 2019;54:800-5. [Crossref] [PubMed]
- Gross S, Trautwein C, Behrens A, et al. Computer-based classification of small colorectal polyps by using narrow-band imaging with optical magnification. Gastrointest Endosc 2011;74:1354-9. [Crossref] [PubMed]
- Takemura Y, Yoshida S, Tanaka S, et al. Computer-aided system for predicting the histology of colorectal tumors by using narrow-band imaging magnifying colonoscopy (with video). Gastrointest Endosc 2012;75:179-85. [Crossref] [PubMed]
- Kominami Y, Yoshida S, Tanaka S, et al. Computer-aided diagnosis of colorectal polyp histology by using a real-time image recognition system and narrow-band imaging magnifying colonoscopy. Gastrointest Endosc 2016;83:643-9. [Crossref] [PubMed]
- Mori Y, Kudo SE, Wakamura K, et al. Novel computer-aided diagnostic system for colorectal lesions by using endocytoscopy (with videos). Gastrointest Endosc 2015;81:621-9. [Crossref] [PubMed]
- Misawa M, Kudo SE, Mori Y, et al. Accuracy of computer-aided diagnosis based on narrow-band imaging endocytoscopy for diagnosing colorectal lesions: comparison with experts. Int J Comput Assist Radiol Surg 2017;12:757-66. [Crossref] [PubMed]
- Endoscopic Classification Review Group. Update on the paris classification of superficial neoplastic lesions in the digestive tract. Endoscopy 2005;37:570-8. [Crossref] [PubMed]
- Hazewinkel Y, Lopez-Ceron M, East JE, et al. Endoscopic features of sessile serrated adenomas: validation by international experts using high-resolution white-light endoscopy and narrow-band imaging. Gastrointest Endosc 2013;77:916-24. [Crossref] [PubMed]
- Hassan C, Pickhardt PJ, Rex DK. A resect and discard strategy would improve cost-effectiveness of colorectal cancer screening. Clin Gastroenterol Hepatol 2010;8:865-9, 869.e1-3.
- Ignjatovic A, East JE, Suzuki N, et al. Optical diagnosis of small colorectal polyps at routine colonoscopy (Detect InSpect ChAracterise Resect and Discard; DISCARD trial): a prospective cohort study. Lancet Oncol 2009;10:1171-8. [Crossref] [PubMed]
- Ferlitsch M, Moss A, Hassan C, et al. Colorectal polypectomy and endoscopic mucosal resection (EMR): European Society of Gastrointestinal Endoscopy (ESGE) Clinical Guideline. Endoscopy 2017;49:270-97. [Crossref] [PubMed]
Cite this article as: Kudo SE, Mori Y, Abdel-aal UM, Misawa M, Itoh H, Oda M, Mori K. Artificial intelligence and computer-aided diagnosis for colonoscopy: where do we stand now? Transl Gastroenterol Hepatol 2021;6:64.