Pancreatic cancer detection and characterization—state of the art cross-sectional imaging and imaging data analysis
Introduction
Pancreatic adenocarcinoma (PDAC) currently ranks as 14th most common cancer and 7th most common cause of cancer related death worldwide. Both are estimated to further increase especially in the developed countries due to an aging population, alimentary risk factors (e.g., obesity, smoking, alcohol) and a lack of specific treatment options (1-3). In the absence of specific clinical symptoms, late diagnosis with usually advanced disease stages at first presentation contribute to the overall poor prognosis in PDAC. The introduction of advanced multimodal treatment protocols with novel chemotherapeutic combinations, now commonly additionally performed in the neoadjuvant setting, have resulted in a less severe disease course. A recent meta-analysis reported median overall survival times of 26.1 months for neoadjuvant chemotherapy followed by surgery (4). Similarly, the introduction of a combination chemotherapy of oxaliplatin, irinotecan and leucovorin (FOLFIRINOX) has improved survival times e.g., in patients diagnosed with a metastatic disease stage (5). Molecularly, several genetic risk factors [e.g., family history, germline mutations (BRCA1, 2; PALB2 Peutz-Jeghers-Syndrome, DNA repair mismatch genes MLH1, MSH2, MSH6, PMS2), genomic aberrations (KRAS, TP53, CDKN2A and SMAD4), and transcriptomic subtypes (squamous, also called basal like or quasi-mesenchymal versus classical, i.e., immunogenic progenitor or pure classical progenitor and exocrine like, i.e., ADEX)] have been identified (6). Despite these significant achievements few factors (e.g., BRCA-ness) currently inform clinical management decision.
The two major challenges from a radiological standpoint are early tumor detection and non-invasive sub-classification of molecularly distinct, albeit (histo-)morphologically similar if not indistinguishable tumors. State-of-the-art cross-sectional imaging [computed tomography (CT) and magnetic resonance imaging (MRI)] can support intensified screening programs, for instance in hereditary and familial disease. However, whereas intraductal papillary mucinous neoplasias (IPMN) and mucinous cystic neoplasms (MCN) present macroscopically visible precursor lesions, pancreatic intraepithelial neoplasms (PanIN) remain undetectable by these imaging modalities and—as such—a domain of invasive imaging by endoscopic ultrasound (EUS). Major advancements in abdominal image quality have been achieved with the introduction of iterative reconstruction algorithms in CT and MRI, spectral CT (i.e., multi-energy CT) and the more widespread availability of higher field strength MRI (3.0 Tesla) in combination with tailored sequences, accelerated acquisition (parallel imaging) and post-processing schemes as well as combinations thereof (e.g., compressed SENSE) (7). Regarding the pre-operative visualization and subtyping along currently developed taxonomies, high-end post-processing and image data analysis tools (radiomics, machine learning) are being developed.
Methods
A PubMed (http://www.ncbi.nlm.nih.gov/pubmed) keyword search (computed/computer tomography, MD/CT, magnetic resonance imaging, MRI, pancreatic ductal adenocarcinoma, PDAC, PCA and radiomics, machine/deep learning) without starting date limitation and up to March 2019 was performed. Reference lists of identified papers were searched. Images were taken from our institution’s picture archiving system (PACS). Individual patient consent was waived by the hospital ethics committee (AZ 180/17S).
Cross-sectional imaging techniques applied in pancreatic cancer
CT
Multiphasic, multi-detector computed tomography (MDCT, CT) with iodine contrast agent (CA) is recommended for PDAC patient staging, including an arterial or parenchymal (30–50 s) and a portal-venous (60–80 s) enhancement phase. CA injection rates should be intermediate with 3–5 mL/s. Patients should be prepared with a negative oral CA (e.g., water) and intestinal motility should be reduced by intravenous application of N-butyl scopolamine. From the raw CT data, a sub-mm isotropic dataset is reconstructed and stored for multiplanar reformation (MPR) and further postprocessing (see Imaging Data Analysis). Reported sensitivities (SE), specificities (SP) and accuracy for the CT-based detection of PDAC are 90% (95% CI, 87–93), 87% (95% CI, 79–93) and 89% (95% CI, 85–93) according to a recent meta-analysis of 15 studies, including 815 PDAC patients (8). However, detection of small PDAC requires expert review of the imaging data (9) and individual retrospective studies report SE/SP values of up to 97%/98%, accordingly (10). Equivalent SE/SP values and higher reader confidence have been reported for dual-energy CT (11) which better delineates tumor from normal adjacent tissue, compared to conventional CT (Figure 1). There are several advantages in CT imaging compared to MRI. CT provides a one-stop-shop staging exam that usually covers thorax and abdomen/pelvis. With the current scanner generation exam times are short (in the range of seconds) and motion artifacts are therefore generally not encountered. CT is widely available and cost effective (with the lower purchase cost resulting in lower exam cost, robustness leading to fewer repeat exams and therefore faster amortization). The necessity for high ionizing radiation doses represents an obstacle for the routine implementation of dynamic scan protocols that could provide additional valuable imaging-based biomarkers (12) and for its use as a screening tool in high-risk patients (e.g., branch-duct IPMN patients). Furthermore, despite the quantitative nature of CT, with scanners routinely being calibrated and radiodensity values of common tissue types having been set by convention and globally valid, acquisition protocol (e.g., pitch), tube current and voltage and detector type and age can influence the final image. As such, the harmonization of protocols for CT has been proposed to limit variance to an acceptable minimum.
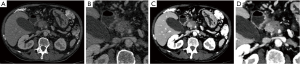
MRI
Multiparametric magnetic resonance imaging (mpMRI) in cases of suspected PDAC should be performed at 3.0T scanners. Since MRI is highly susceptible to motion artifacts, fast imaging protocols (using parallel imaging, compressed sensing) are required to achieve robust image quality. In addition, patient training/preparation and intravenous N-butyl scopolamine (e.g., 20 mg) are recommended to reduce controllable (breathing) and uncontrollable (bowel) motion. For T2w images fast spin echo sequences should be applied for thin (3 mm) axial and coronal acquisitions. Diffusion weighted-MRI (DW-MRI), i.e., the detection of random motion of water molecules, is a CA free technique that allows the quantification of tissue microstructure, e.g., increased cellularity and macromolecular extracellular matrix content in PDAC, which is calculated from multiple b-value acquisitions and expressed as apparent diffusion coefficient (ADC). Most often echo planar imaging (EPI) sequences are used in combination with respiratory triggering. For time constraints a maximum of up to 4 b-values are measured and ADC values are calculated by mono-exponential fitting. A dynamic 3D gradient-echo (GRE) T1w sequence using macrocyclic gadolinium-based CAs (2–3 mL/s) is recommended despite current concerns regarding Gd-deposition in the brain (13). Magnetic resonance cholangiopancreatography (MRCP), applying heavily T2w sequences, is the method of choice for the morphology-based classification of IPMN lesions into main-duct (MD), side-branch and mixed type lesions according to the revised Fukuoka criteria (14,15). MRCP data sets should be carefully reviewed in combination with high-resolution (i.e., reduced field of view) DW-MRI for the presence of solid lesions that may indicate the development of malignancy within an IPMN or concomitant PDAC and that require further work-up by EUS. Navigated or single breath-hold 3D MRCP acquisitions that allow for MPRs should be used (16).
Reported sensitivity, specificity and accuracy of mpMRI for the detection of PDAC are higher compared to CT with 93% (95% CI, 88–96), 89% (95% CI, 82–94) and 90% (95% CI, 86–94) from pooled data of 11 studies that included 349 PDAC patients (8). However, the same reservations brought up for reported SE/SP values for CT imaging of PDAC also apply for MRI. In addition, abdominal mpMRI has greatly benefited from recent technical developments, also not adequately reflected in older studies (17).
In addition to the detection and characterization of the primary tumor, mpMRI including DW-MRI presents a highly sensitive technique for the detection of liver metastases, making a combination of staging CT and mpMRI of the upper abdomen the ideal baseline imaging protocol for PDAC patients.
Cross sectional imaging findings in pancreatic cancer
Indirect signs of cancer
Indirect signs of PDAC are pancreatic duct dilations and the so-called “double-duct sign” with dilation of the pancreatic and the bile duct, seen with tumors developing in the pancreatic head region, albeit not specific for PDAC and also seen with tumors originating from the distal bile duct or papilla. Duct dilations are detected with very high sensitivity on T2w images and by MRCP. In cases of a pancreas divisum the separately at the minor papilla draining dorsal duct may appear normal with tumors arising in the head region near or at the ventral duct/major papilla. Conversely, even small lesions can (partially) obstruct and result in a dilation of the main pancreatic duct (MPD) or side branches as an early sign of tumor development that has a high sensitivity, albeit low specificity, since duct dilations are also frequently seen as a result of prior inflammatory episodes. Nevertheless, when present, a careful review of mpMRI data should follow and even further work-up by EUS or short-term follow-up imaging may be indicated since it can provide an opportunity to detect PDAC at an early (i.e., curable) tumor stage (Figure 2). In contrast, in the case of focal inflammatory processes, ductal structures are often partially maintained (i.e., penetrating duct sign).
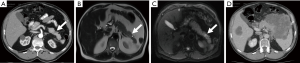
Precursor lesion detection and characterization
The direct detection of small pancreatic malignancies remains challenging despite major technological advances such as dual energy CT, 3.0 T MRI and improved post-processing techniques. Direct visualization of the most common PDAC precursor lesion, pancreatic intraepithelial neoplasias (PanIN), which are typically <5 mm in size, is beyond resolution of routine cross-sectional imaging. However, the presence of non-communicating microcysts (best visualized on T2w imaging and MRCP) identified in an atrophic pancreas has shown association with histopathologically confirmed PanIN lesions (18), a finding requiring prospective evaluation.
The other common precursor lesions, intraductal papillary mucinous neoplasms (IPMN) are morphologically subdivided into main-duct (MD), branch-duct (BD) and mixed-type (MT) IPMN. MD- and MT-IPMN have a high prevalence of malignancy and are easily identified by a MPD diameter of ≥10 mm and therefore do not present a radiological challenge. In BD-IPMNs the revised Fukuoka guidelines specify imaging features that correlate with the risk of the development of malignancy within 3 years. High risk stigmata (50%) include new onset jaundice, the detection of contrast-agent (CA) affine nodules ≥5 mm and/or a MPD diameter ≥10 mm, whereas worrisome features (5%) include a cyst diameter ≥3 cm at diagnosis or a >5 mm increase in cyst diameter within 2 years, thickened cyst walls, enhancing nodules <5 mm/enhancing septations, MPD diameter of 5–9 mm with distal atrophy and/or lymphadenopathy. More recent publications propose an increased risk for the presence of nodules >5 mm independent of CA uptake and/or cyst growth >5 mm/year (19) (Figure 3).
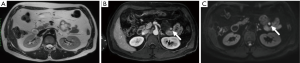
Diffusion weighted-MRI
In DW-MRI high b-value images have a high sensitivity for the detection of focal pathologies. However, further differentiation of e.g., focal pancreatitis from PDAC presents a major challenge, as both pathologies can result in an increased cellularity compared to normal pancreas. In addition, varying degrees of tumor desmoplasia, differences in tumor perfusion and the presence of tumor necrosis further complicate the interpretation of a given DW-MRI derived, calculated ADC value and both, lower and higher ADC values, have been reported for PDAC in comparison to focal pancreatitis (20,21). Nevertheless, DW-MRI holds a great potential for the detection of solid cancer development in IPMN (22) and it may be helpful in the non-invasive differentiation of tumor subtypes and monitoring of therapy response (12,23,24). The inclusion of high b-values of up to 2,000 s/mm2 may further improve the delineation of PDAC from adjacent inflammatory changes because of the increased diffusion-weighted and decreased T2w contribution (25). Advanced DW-MRI techniques (i.e., intravoxel incoherent motion, IVIM) including b-values below 200 s/mm2 allow for the quantification of the relative contribution of tissue perfusion and true molecular diffusion to the diffusion of water molecules, which may enable a better characterization of tissue microstructure (26), such as the differentiation of focal pancreatic lesions (e.g., mass forming pancreatitis) and PDAC based on an IVIM derived perfusion fraction (27).
Contrast enhanced imaging
CT and MRI enable the reliable identification of PDAC lesions ≥5 mm. In native CT and MRI scans, tumors may be revealed by their solid growth pattern compared to the lobulated appearance of the normal and especially the atrophic glandular pancreas, which shows interleaved fat. The signal intensity of PDAC depends on the imaging modality and technique; on native imaging PDAC usually presents as isodense (CT), hypointense (T1w), hypo-/hyperintense (T2w) or hyperintense (b600) lesion. Ductal obstruction can lead to edema of the adjacent “normal” pancreas tissue distal to the tumor and thus may obscure tumor borders. An unequivocal morphological distinction of PDAC from acute or chronic pancreatitis can be challenging, especially if these are focal, requiring EUS- or CT-guided biopsy.
In contrast enhanced CT/-MRI PDAC generally appear hypodense/-intense compared to the surrounding normal pancreatic tissue. This has been related to particularly low microvessel density (MVD) values compared to other tumor entities, abundant matrix deposition [mainly collagen type I and III, fibronectin and hyaluronic acid (28)] and a subsequent increase in intra-tumoral pressure (i.e., solid stress), also resulting in vessel collapse (29,30). Contradicting these findings at first sight (31) a positive correlation of stroma content and perfusion has been described, suggesting a more complicated interplay of the tumor microenvironment, where tumor cellularity in the presence of matrix deposition can be an additional if not dominating factor in the build-up of solid stress and hypoperfusion (29,30,32). Furthermore, the detection of a high lesion to background ratio at the tumor border has been identified as an aggressive imaging features that predicts poor clinical outcome (31).
Tumor growth beyond organ borders is often seen at primary staging and is regularly accompanied by a strong desmoplastic reaction of hazy or solid appearance that may help distinguish PDAC from focal inflammation especially at the vascular interface (Figure 4). However, as tumor development is often accompanied by inflammation, peri-pancreatic changes are no proof of tumor growth beyond organ borders.

Structured reporting
Imaging findings should be reported in a standardized manner according to current guidelines (33), preferably using simplified and user-friendly templates, to improve acceptance among radiologists (34). Most importantly, templates must include a detailed description of the tumor to vessel interface, which (in combination with the identification/rule out of metastatic disease) directly impacts patients stratification into primary resectable, borderline resectable and non-resectable tumors (33). It should differentiate between tumor to vessel contact and signs of vascular infiltration that require vascular reconstruction in case of surgery (35). Reports should be saved in machine readable formats to enable further processing.
Cross sectional imaging data analysis in pancreatic cancer
Technical advances have enabled significant gains in both image quality and acquisition speed. However, tumor identification and response evaluation still rely primarily on naked eye methods. For this reason, the output quality of radiological reports is determined to a great extent by radiologist training, experience and even external factors such as noise levels within the reporting room, stress and rest (36,37). Therefore, techniques to facilitate tumor detection and quantify image information in a more objective way have generated substantial interest and advances in computational performance of image reconstruction and postprocessing systems as well as electronic health records have enabled a multitude of workflow improvements for radiologists (38). These can be divided into tools facilitating the visualization and communication of findings and tools for quantitative image analysis.
Workflow, visualization and communication improvements
Due to significant improvements in computation and especially due to the widespread availability of parallel computing solutions, such as GPU-based rendering, coupled with the acceleration of data transfer speeds, radiologists can now routinely gain access to e.g., thin isotropic CT slices within minutes after their acquisition (39). These can then be processed on the fly at the reporting station to generate custom views, such as MPRs, and volume-rendered images such as maximum intensity projection (MIP) or cinematic rendering series. Such tools can greatly facilitate not only the radiologist’s understanding of pathology and pathological anatomy, but also aid in the demonstration of findings to referring clinicians and assist e.g., in operative planning or the production of custom medical devices, such as 3D-printed prosthetics or implants (40). Furthermore, the abovementioned advances in communications technology mean that images can now be stored in high-efficiency compressed and encrypted formats in the cloud and made available at a moment’s notice in the case of future need (41). Lastly, radiological reporting or expert opinion can now be outsourced to third parties over secure high-speed internet connections (42). This has led to the emergence of virtual tumor boards over state-of-the-art videoconferencing systems and greatly improved availability of radiological reporting in underprivileged areas of the world (43).
Quantitative imaging analysis
To gain a better terminological understanding, one must differentiate between the two common uses of the term quantitative imaging. On one hand, it can refer to the quantitative assessment of specific parameters which relate to concrete physical measurements, as can be performed with positron emission tomography, which is said to be a quantitative modality since the (radio-)activity per unit volume can be directly measured. In this sense, CT can also be referred to as quantitative since the scanners are calibrated and radiodensity is expressed in Hounsfield Units, which are similar or identical for the same materials. Certain MRI sequences can also produce quantitative measurements, for instance so-called T1-mapping sequences, which allow statements about the relaxivity or the precise quantification of contrast media uptake within a volume of interest.
In its second guise, the term quantitative imaging has come to denote the derivation of objective, mathematically quantifiable data from a certain part of the image (44). With the advent of so-called -omics approaches, this ports radiological images into the domain of advanced statistical analysis by high-performance computer systems, a field which within the last decade has become known as Data Science. The -omics approach to radiology is commonly called radiomics (45). Typically, a radiomics workflow consists of the definition of a volume of interest within the image, the derivation of data from this region and the statistical exploration of this data and its correlation with clinical, histopathological or genetic parameters as delineated below:
Volume of interest definition
The first step towards quantitative analysis of radiological images consists of the selection of a specific image sub-area, a process commonly called image segmentation. In its simplest form, it can be performed by positioning for instance a circular region of interest (ROI) in a specific area to gain insight into its radiodensity, a process very common to the radiologist for deciding for instance whether an adrenal lesion contains fat. In a more advanced setting such as pancreatic cancer, complete analysis of the tumor requires precise definitions of the tumor margins in all slices and/or in multiple axes. In high-contrast settings, segmentation can be performed by the computer itself (46). This can be observed for instance for pulmonary lesions, since it is easy to program a computer to search for high-contrast areas and for specific density/intensity values. In pancreatic cancer, typically an ill-defined, hypovascular entity within a complexly formed organ which often carries the mark of chronic disease or is altered by the presence of the tumor itself, however, even modern, artificial-intelligence-based algorithms have great difficulties delineating the tumor margins and—at worst—reach accuracies only as high as about 60% (47). The fully automatic segmentation of pancreatic cancers is a subject of ongoing research with the most successful approaches currently first having the algorithm identify the pancreas, and consecutively the tumor region, all while integrating previously gained knowledge from manually segmented datasets (48).
In any case, the end result of the segmentation process is a region or volume of interest, in essence just than a subsample of image pixels/voxels of the original acquired volume.
Derivation of imaging parameters
The second step in the radiomics approach regards deriving mathematical descriptions about the spatial relationship and the intensity values of the pixels within the volumes of interest, which are commonly referred to as imaging features (49). Some features common to radiologist and clinician alike are for instance tumor volume or mean, maximum and minimum intensity, the latter important in the abovementioned setting of deciding whether a lesion contains fat (i.e., negative CT-value pixels). However, innumerable other approaches, some with substantial mathematical complexity, exist for the derivation of features which attempt to quantify more abstract concepts, such as tumor heterogeneity (50). These approaches are based on statistics and probability and extract information from the spatial positions or the probability of occurrence of pixels with a certain density or signal intensity and include for example histogram metrics, gray-level-cooccurence matrices, local binary patterns and wavelet decompositions (51).
Exploration and correlation of imaging features with clinical, pathological or genomic data
At this point in the radiomic workflow, the transformation of what was originally visual data into a quantitative, mathematical entity has taken place. Imaging features can now be used within the context of a biostatistics workflow and treated like clinical information such as tumor marker levels or tumor stage. Typically, however, some pre-processing of these features has to take place, as is common in other bioinformatics workflows, to separate useful predictors from uninformative features. For this purpose, a feature selection step takes place using statistical or machine learning methods such as regularization or principal component analysis, which aims to reduce the number of features and increase their significance for the overall analysis (52). The remaining features are then included in statistical models of e.g., tumor progression, survival models or compared to features derived from other entities, such as for the prediction of genetic signatures or histopathological tumor subtypes.
With the increase in data quantity and complexity, traditional statistical approaches are increasingly complemented or superseded by artificial intelligence methods, or—more specifically—machine learning techniques (53). Machine learning denotes any algorithm which does not require explicit (i.e., imperative or declarative) programming but is capable of handling new information in a process akin to human learning, i.e., by integrating the new information and improving over time. With the exponential increase in computing power, a specific type of machine learning algorithm, so called artificial neural networks (54), which are designed with a similar architecture as the human nervous system, i.e., with inputs, processing “neurons” and outputs, have shown great promise in use-cases such as image analysis. Very complex neural networks, using several stages (or layers) of “neurons” are commonly referred to as deep neural networks, and the process of training these neurons, i.e., generating a model for the prediction of a certain state from the input data, is called deep learning. Although it is beyond the scope of this article to deliberate the specifics of deep learning (55), suffice it to denote that they represent a major advance in image analysis and can potentially replace the traditional radiomics workflow as it is currently practiced: they have shown considerable success in the automatic detection and segmentation of structures and are supremely suitable for image classification (56). This makes deep learning based approaches an ideal candidate for future quantitative image analysis workflows (57). The great challenge lies in the training of such algorithms, which in many cases requires large amounts of precisely annotated data to ascertain sufficient model performance and the ability of the model to generalize beyond the scope of specific datasets. In any case, machine learning and artificial intelligence hold great potential for radiological research and can be envisioned as integral tools of the clinical radiological workflow of the future (58), for instance for triaging exams for high-relevance findings, assisting with tumor volumetry or as a decision support tool for patient risk stratification.
Summary and conclusions
Early tumor detection, non-invasive tumor subtyping and treatment response evaluation present major challenges in the radiological work-up of patients at risk and patients who have already developed pancreatic cancer. Intensified screening programs with state-of-the-art imaging techniques and advanced image post-processing algorithms are being developed and hold the potential to improve patient outcome.
Acknowledgements
R Braren is supported by funding of the German Research Foundation (DFG, http://www.dfg.de/en/) within the SFB-Initiative 824 (collaborative research center, http://www.sfb824.de/en/), “Imaging for Selection, Monitoring and Individualization of Cancer Therapies” (SFB824, project C6).
Footnote
Conflicts of Interest: The authors have no conflicts of interest to declare.
References
- McGuigan A, Kelly P, Turkington RC, et al. Pancreatic cancer: A review of clinical diagnosis, epidemiology, treatment and outcomes. World J Gastroenterol 2018;24:4846-61. [Crossref] [PubMed]
- Siegel RL, Miller KD, Jemal A. Cancer statistics, 2017. CA Cancer J Clin 2017;67:7-30. [Crossref] [PubMed]
- Rahib L, Smith BD, Aizenberg R, et al. Projecting Cancer Incidence and Deaths to 2030: The Unexpected Burden of Thyroid, Liver, and Pancreas Cancers in the United States. Cancer Res 2014;74:2913-21. [Crossref] [PubMed]
- Versteijne E, Vogel JA, Besselink MG, et al. Meta-analysis comparing upfront surgery with neoadjuvant treatment in patients with resectable or borderline resectable pancreatic cancer. Br J Surg 2018;105:946-58. [Crossref] [PubMed]
- Conroy T, Hammel P, Hebbar M, et al. FOLFIRINOX or Gemcitabine as Adjuvant Therapy for Pancreatic Cancer. N Engl J Med 2018;379:2395-406. [Crossref] [PubMed]
- Collisson EA, Bailey P, Chang DK, et al. Molecular subtypes of pancreatic cancer. Nat Rev Gastroenterol Hepatol 2019;16:207-20. [Crossref] [PubMed]
- Sartoretti T, Reischauer C, Sartoretti E, et al. Common artefacts encountered on images acquired with combined compressed sensing and SENSE. Insights Imaging 2018;9:1107-15. [Crossref] [PubMed]
- Toft J, Hadden WJ, Laurence JM, et al. Imaging modalities in the diagnosis of pancreatic adenocarcinoma: A systematic review and meta-analysis of sensitivity, specificity and diagnostic accuracy. Eur J Radiol 2017;92:17-23. [Crossref] [PubMed]
- Corrias G, Huicochea Castellanos S, Merkow R, et al. Does Second Reader Opinion Affect Patient Management in Pancreatic Ductal Adenocarcinoma? Acad Radiol 2018;25:825-32. [Crossref] [PubMed]
- Gangi S, Fletcher JG, Nathan MA, et al. Time interval between abnormalities seen on CT and the clinical diagnosis of pancreatic cancer: retrospective review of CT scans obtained before diagnosis. AJR Am J Roentgenol 2004;182:897-903. [Crossref] [PubMed]
- Quiney B, Harris A, McLaughlin P, et al. Dual-energy CT increases reader confidence in the detection and diagnosis of hypoattenuating pancreatic lesions. Abdom Imaging 2015;40:859-64. [Crossref] [PubMed]
- Klauß M, Stiller W, Pahn G, et al. Dual-energy perfusion-CT of pancreatic adenocarcinoma. Eur J Radiol 2013;82:208-14. [Crossref] [PubMed]
- Gulani V, Calamante F, Shellock FG, et al. Gadolinium deposition in the brain: summary of evidence and recommendations. Lancet Neurol 2017;16:564-70. [Crossref] [PubMed]
- Tanaka M, Fernández-del Castillo C, Kamisawa T, et al. Revisions of international consensus Fukuoka guidelines for the management of IPMN of the pancreas. Pancreatology 2017;17:738-53. [Crossref] [PubMed]
- European Study Group on Cystic Tumours of the Pancreas. European evidence-based guidelines on pancreatic cystic neoplasms. Gut 2018;67:789-804. [Crossref] [PubMed]
- Lohöfer FK, Kaissis GA, Rasper M, et al. Magnetic resonance cholangiopancreatography at 3 Tesla: Image quality comparison between 3D compressed sensing and 2D single-shot acquisitions. Eur J Radiol 2019;115:53-8. [Crossref] [PubMed]
- Zhang B, Peeters H. Efficient MR inhomogeneity correction by regularized entropy minimization and proximal alternations. In: 2015 IEEE 12th International Symposium on Biomedical Imaging (ISBI). IEEE 2015:1069-72.
- Vullierme MP, Menassa L, Couvelard A, et al. Non-branched microcysts of the pancreas on MR imaging of patients with pancreatic tumors who had pancreatectomy may predict the presence of pancreatic intraepithelial neoplasia (PanIN): a preliminary study. Eur Radiol 2019. [Epub ahead of print]. [Crossref] [PubMed]
- Kwong WT, Hunt GC, Fehmi SM, et al. Low Rates of Malignancy and Mortality in Asymptomatic Patients With Suspected Neoplastic Pancreatic Cysts Beyond 5 Years of Surveillance. Clin Gastroenterol Hepatol 2016;14:865-71. [Crossref] [PubMed]
- Matsuki M, Inada Y, Nakai G, et al. Diffusion-weighed MR imaging of pancreatic carcinoma. Abdom Imaging 2007;32:481-3. [Crossref] [PubMed]
- Wang Y, Chen ZE, Nikolaidis P, et al. Diffusion-weighted magnetic resonance imaging of pancreatic adenocarcinomas: Association with histopathology and tumor grade. J Magn Reson Imaging 2011;33:136-42. [Crossref] [PubMed]
- Hoffman DH, Ream JM, Hajdu CH, et al. Utility of whole-lesion ADC histogram metrics for assessing the malignant potential of pancreatic intraductal papillary mucinous neoplasms (IPMNs). Abdom Radiol (NY) 2017;42:1222-8. [Crossref] [PubMed]
- Collisson EA, Sadanandam A, Olson P, et al. Subtypes of pancreatic ductal adenocarcinoma and their differing responses to therapy. Nat Med 2011;17:500-3. [Crossref] [PubMed]
- Trajkovic-Arsic M, Heid I, Steiger K, et al. Apparent Diffusion Coefficient (ADC) predicts therapy response in pancreatic ductal adenocarcinoma. Sci Rep 2017;7:17038. [Crossref] [PubMed]
- Fukukura Y, Shindo T, Hakamada H, et al. Diffusion-weighted MR imaging of the pancreas: optimizing b-value for visualization of pancreatic adenocarcinoma. Eur Radiol 2016;26:3419-27. [Crossref] [PubMed]
- Le Bihan D, Breton E, Lallemand D, et al. Separation of diffusion and perfusion in intravoxel incoherent motion MR imaging. Radiology 1988;168:497-505. [Crossref] [PubMed]
- De Robertis R, Cardobi N, Ortolani S, et al. Intravoxel incoherent motion diffusion-weighted MR imaging of solid pancreatic masses: reliability and usefulness for characterization. Abdom Radiol (NY) 2019;44:131-9. [Crossref] [PubMed]
- Bailey JM, Swanson BJ, Hamada T, et al. Sonic Hedgehog Promotes Desmoplasia in Pancreatic Cancer. Clin Cancer Res 2008;14:5995-6004. [Crossref] [PubMed]
- Provenzano PP, Cuevas C, Chang AE, et al. Enzymatic Targeting of the Stroma Ablates Physical Barriers to Treatment of Pancreatic Ductal Adenocarcinoma. Cancer Cell 2012;21:418-29. [Crossref] [PubMed]
- Jacobetz MA, Chan DS, Neesse A, et al. Hyaluronan impairs vascular function and drug delivery in a mouse model of pancreatic cancer. Gut 2013;62:112-20. [Crossref] [PubMed]
- Koay EJ, Truty MJ, Cristini V, et al. Transport properties of pancreatic cancer describe gemcitabine delivery and response. J Clin Invest 2014;124:1525-36. [Crossref] [PubMed]
- Stylianopoulos T, Martin JD, Chauhan VP, et al. Causes, consequences, and remedies for growth-induced solid stress in murine and human tumors. Proc Natl Acad Sci 2012;109:15101-8. [Crossref] [PubMed]
- Al-Hawary MM, Francis IR, Chari ST, et al. Pancreatic Ductal Adenocarcinoma Radiology Reporting Template: Consensus Statement of the Society of Abdominal Radiology and the American Pancreatic Association. Radiology 2014;270:248-60. [Crossref] [PubMed]
- Kambadakone AR, Zaheer A, Le O, et al. Multi-institutional survey on imaging practice patterns in pancreatic ductal adenocarcinoma. Abdom Radiol (NY) 2018;43:245-52. [Crossref] [PubMed]
- Kaissis GA, Lohöfer FK, Ziegelmayer S, et al. Borderline-resectable pancreatic adenocarcinoma: Contour irregularity of the venous confluence in pre-operative computed tomography predicts histopathological infiltration. PLoS One 2019;14:e0208717. [Crossref] [PubMed]
- Bruno MA, Walker EA, Abujudeh HH. Understanding and Confronting Our Mistakes: The Epidemiology of Error in Radiology and Strategies for Error Reduction. RadioGraphics 2015;35:1668-76. [Crossref] [PubMed]
- Waite S, Scott J, Gale B, et al. Interpretive Error in Radiology. AJR Am J Roentgenol 2017;208:739-49. [Crossref] [PubMed]
- Wildman-Tobriner B, Thorpe MP, Said N, et al. Moving Radiology Workflow to the Electronic Health Record: Quantitative and Qualitative Experience From a Large Academic Medical Center. Acad Radiol 2019. [Epub ahead of print]. [Crossref] [PubMed]
- Shi L, Liu W, Zhang H, et al. A survey of GPU-based medical image computing techniques. Quant Imaging Med Surg 2012;2:188-206. [PubMed]
- Mitsouras D, Liacouras P, Imanzadeh A, et al. Medical 3D Printing for the Radiologist. RadioGraphics 2015;35:1965-88. [Crossref] [PubMed]
- Mezrich JL, Siegel E. Storing Medical Images in the Digital Age: The Need for Universal and Technologically Appropriate Guidelines. J Am Coll Radiol 2017;14:752-4. [Crossref] [PubMed]
- Davis A. Outsourced radiology: will doctors be deskilled? BMJ 2008;337:a785. [Crossref] [PubMed]
- Shea CM, Teal R, Haynes-Maslow L, et al. Assessing the feasibility of a virtual tumor board program: a case study. J Healthc Manag 2014;59:177-93. [Crossref] [PubMed]
- Rosenkrantz AB, Mendiratta-Lala M, Bartholmai BJ, et al. Clinical utility of quantitative imaging. Acad Radiol 2015;22:33-49. [Crossref] [PubMed]
- Kumar V, Gu Y, Basu S, et al. Radiomics: The process and the challenges. Magn Reson Imaging 2012;30:1234-48. [Crossref] [PubMed]
- Larue RT, Defraene G, De Ruysscher D, et al. Quantitative radiomics studies for tissue characterization: a review of technology and methodological procedures. Br J Radiol 2017;90:20160665. [Crossref] [PubMed]
- Zhou Y, Xie L, Shen W, et al. Pancreas Segmentation in Abdominal CT Scan: A Coarse-to-Fine Approach. Available online: https://www.semanticscholar.org/paper/Pancreas-Segmentation-in-Abdominal-CT-Scan%3A-A-Zhou-Xie/788f341d02130e1807edf88c8c64a77e4096437e
- Wolz R, Chu C, Misawa K, et al. Automated Abdominal Multi-Organ Segmentation With Subject-Specific Atlas Generation. IEEE Trans Med Imaging 2013;32:1723-30. [Crossref] [PubMed]
- Gillies RJ, Kinahan PE, Hricak H. Radiomics: Images Are More than Pictures, They Are Data. Radiology 2016;278:563-77. [Crossref] [PubMed]
- Lubner MG, Smith AD, Sandrasegaran K, et al. CT Texture Analysis: Definitions, Applications, Biologic Correlates, and Challenges. RadioGraphics 2017;37:1483-503. [Crossref] [PubMed]
- Zwanenburg A, Leger S, Vallières M, et al. Image biomarker standardisation initiative. 2016. Available online: https://arxiv.org/abs/1612.07003
- Yip SS, Aerts HJ. Applications and limitations of radiomics. Phys Med Biol 2016;61:R150-66. [Crossref] [PubMed]
- Parmar C, Grossmann P, Bussink J, et al. Machine Learning methods for Quantitative Radiomic Biomarkers. Sci Rep 2015;5:13087. [Crossref] [PubMed]
- van Gerven M, Bohte S. Editorial: Artificial Neural Networks as Models of Neural Information Processing. Front Comput Neurosci 2017;11:114. [Crossref] [PubMed]
- LeCun Y, Bengio Y, Hinton G. Deep learning. Nature 2015;521:436. [Crossref] [PubMed]
- Krizhevsky A, Sutskever I, Hinton GE. ImageNet classification with deep convolutional neural networks. Commun ACM 2017;60:84-90. [Crossref]
- Greenspan H, van Ginneken B, Summers RM. Guest Editorial Deep Learning in Medical Imaging: Overview and Future Promise of an Exciting New Technique. IEEE Trans Med Imaging 2016;35:1153-9. [Crossref]
- Esteva A, Robicquet A, Ramsundar B, et al. A guide to deep learning in healthcare. Nat Med 2019;25:24-9. [Crossref] [PubMed]
Cite this article as: Kaissis G, Braren R. Pancreatic cancer detection and characterization—state of the art cross-sectional imaging and imaging data analysis. Transl Gastroenterol Hepatol 2019;4:35.